Mastering Demand Planning Forecasting Techniques
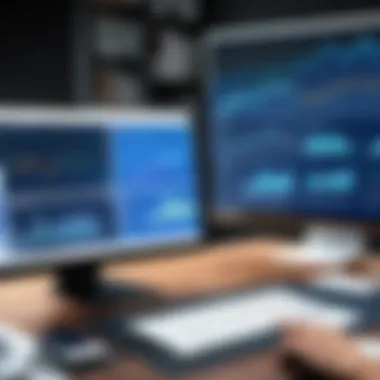
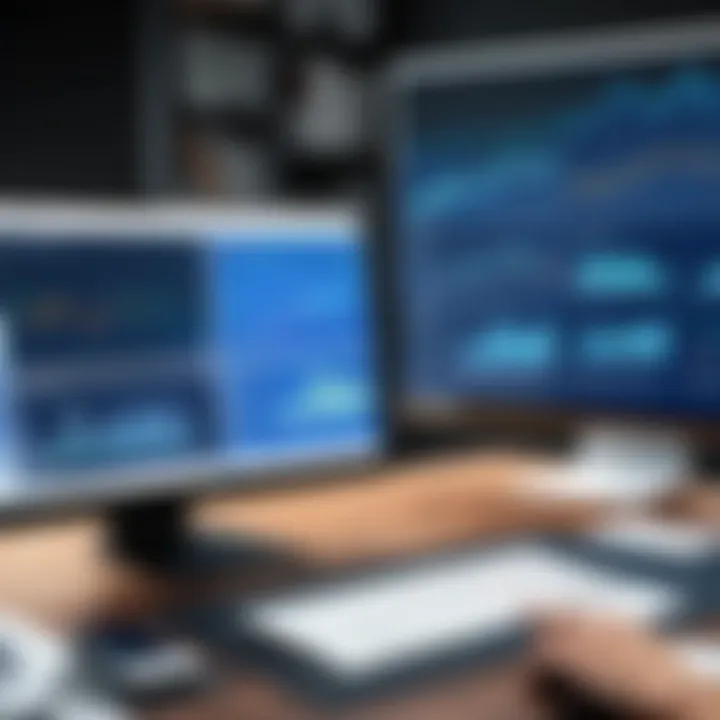
Intro
In today’s fast-paced market environment, understanding how to anticipate and manage customer demand is pivotal. The essence of demand planning forecasting lies in its capacity to not only meet customer needs but to also ensure that resources are utilized efficiently. With technology evolving and consumer behaviors shifting, organizations are often left scrambling to keep up. Hence, a deep dive into the nuances of demand planning forecasting is not just an academic exercise—it's a necessity.
This guide will illuminate critical aspects surrounding demand planning forecasting. From examining key features that enhance forecasting accuracy to identifying target users in various industries, every facet will be explored. Moreover, we will discuss pricing models and the associated costs involved, providing a well-rounded perspective. By the end of this narrative, readers will be well-equipped with the insights and strategies needed to navigate the complexities of modern demand forecasting.
Demand forecasting is more than just a buzzword; it's the backbone of operational efficiency. By aligning production, inventory, and customer expectations, organizations can achieve a competitive edge—and that’s the crux of our exploration.
Foreword to Demand Planning Forecasting
Demand planning forecasting goes far beyond just estimating future sales. It lays the groundwork for how businesses align their production, supply chain, and inventory management with the ever-shifting ebbs and flows of customer demand. In today’s competitive landscape, accurate forecasting isn’t just a nice-to-have; it’s essential. Companies that get this right not only streamline their operations but also create a smoother experience for their customers.
At its core, demand planning forecasting helps organizations anticipate market needs, allowing them to adjust their resources effectively. Decisions around production schedules, inventory levels, and staffing are heavily influenced by accurate forecasts. If a company can predict surges in demand for specific products, it can ramp up production before hitting that spike, thereby avoiding potential stockouts and lost revenue. Conversely, poor demand estimation can lead to overproduction, excess inventory, and consequent financial strain.
Definition of Demand Planning Forecasting
Demand planning forecasting can be defined as the process of predicting future customer demand for a product or service based on historical data and market analysis. This discipline amalgamates various techniques—from statistical analysis to market research—to generate reliable predictions. It’s not a simple matter of looking at past sales figures; it requires a nuanced understanding of various factors that could affect future demand, such as market trends, seasonality, and even sociopolitical influences.
By using demand planning forecasting, businesses can allocate resources more proficiently, thereby reducing waste and improving overall efficiency.
The Importance of Accurate Forecasting
Accurate forecasting is more than a technical exercise; it’s a strategic imperative. Consider the consequences of getting it wrong. An under-forecast might mean you’re scrambling to fulfill unexpected demand, risking customer dissatisfaction and lost loyalty. On the flip side, overestimating demand can lead to warehouses full of unsold inventory.
"Organizations that master the art of demand forecasting can better navigate market turbulence, staying one step ahead of competitors and ensuring customer satisfaction."
Some specific benefits of accurate forecasting include:
- Informed Decision-Making: Access to reliable data helps teams make better operational decisions—from procurement to staffing.
- Cost Savings: Reducing excess stock lowers storage costs and waste, enhancing the bottom line.
- Enhanced Customer Satisfaction: Meeting customer need promptly builds trust and loyalty.
- Sales and Revenue Growth: Businesses can seize market opportunities with the right product at the right time, maximizing sales potential.
In essence, demand planning forecasting serves as the backbone of efficient supply chain management. Without it, organizations risk operational inefficiencies, financial loss, and reputational damage.
Key Components of Demand Planning
Demand planning forecasting is a multifaceted discipline. At the core of effective demand planning are the essential components that guide the entire forecasting process. These components serve as the backbone, ensuring decisions are made on solid ground. When well-executed, they empower organizations to align their production, supply, and inventory strategies with actual consumer demand.
Data Collection Methods
The first step in demand planning lies in data collection. This phase is crucial because the quality of data influences every subsequent aspect of demand forecasting. Traditional methods involve reviewing historical sales data, but the scope goes beyond just number crunching. It includes various formats and aspects:
- Point-of-sales data: This involves detailed records from retail environments where actual sales occur. Understanding daily sales fluctuations at multiple locations offers a real-time view of consumer behavior.
- Market research and surveys: Collecting feedback directly from customers allows businesses to grasp emerging trends and preferences that may not yet affect sales figures.
- Social media and web analytics: Monitoring online interactions provides insights into consumer sentiments. Tools that track engagements across platforms like Facebook or Reddit can reveal trends before they might impact the bottom line.
Utilizing a combination of these methods gives a richer picture, which helps assess future demands more accurately.
Statistical Techniques in Forecasting
Once data is on hand, the focus shifts to analyzing it through statistical techniques. Here’s where numbers transform into foresight. One notable approach is time series analysis, where historical data is evaluated to predict future trends. This method considers seasonal variations, making it particularly valuable for businesses facing cyclical demand.
- Moving averages: A straightforward technique that smooths out short-term fluctuations, allowing for clearer long-term trends.
- Regression analysis: This technique helps identify relationships between different variables, providing a more nuanced understanding of demand drivers. For instance, better comprehension of how economic variables like employment rates affect sales can enhance predictions significantly.
"Good data leads to good decisions, and good decisions are essential for successful demand planning."
By effectively applying these statistical methods, organizations can improve accuracy in their forecasts, minimizing gaps between supply and demand.
Customization for Industry-Specific Needs
Every industry has its peculiarities and demands. A one-size-fits-all approach doesn't often cut it. Customization is key to ensuring that the forecasting model aligns with industry nuances. For example, in the fast-paced world of fashion retail, demand can swing dramatically based on seasonal shifts and trends. Thus, using quick response practices becomes beneficial.
On the other hand, industries like pharmaceuticals rely on steady consumption patterns and regulatory timelines, highlighting the need for a different approach. Using industry-specific indicators enables organizations to forecast effectively. Considerations include:
- Historical data relevance: Evaluate how past trends specifically correlate with future results in each industry.
- External factors: Economic shifts and competitive dynamics play unique roles. Monitoring these helps adapt quickly to changing landscapes.
In summary, understanding demand planning's core components is vital for effective forecasting. From robust data collection methods to industry-tailored customization, each piece contributes to the larger puzzle of supply chain efficiency and operational success. Adjustments in these areas will not only help illuminate future demand but also enhance responsiveness in a volatile market.
Forecasting Techniques
Forecasting techniques stand as the backbone of effective demand planning. They allow businesses to predict customer preferences, adjust their production schedules, and maintain optimal inventory levels. Understanding these techniques is not just an academic exercise; it is crucial for anyone involved in making strategic decisions. Businesses that excel in demand forecasting optimize their resources, improve customer satisfaction, and, importantly, enhance their bottom line.
By utilizing the proper forecasting method, organizations can respond swiftly to market fluctuations and prepare for shifts in consumer demand. There’s a rich variety of approaches available, from qualitative methods that capitalize on expert insights to quantitative techniques grounded in mathematical analysis.
Qualitative Forecasting Methods
Qualitative forecasting is rooted in the subjective interpretation of market conditions. These methods are beneficial when dealing with new products or markets lacking historical data. The strength of these methods lies in their ability to incorporate human judgment, intuition, and expertise into the forecasting process.
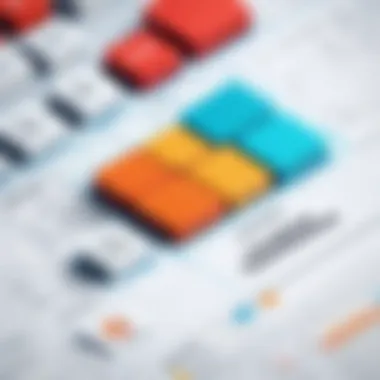
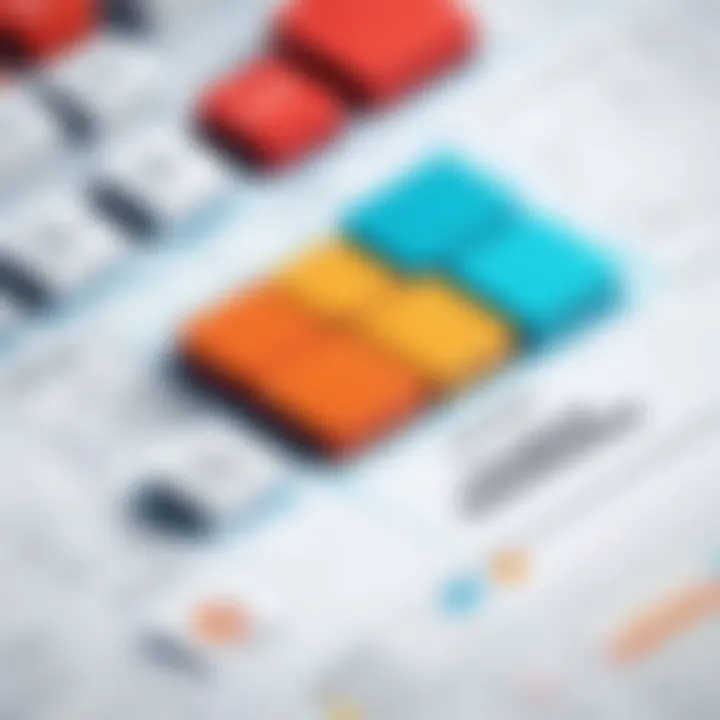
Common qualitative methods include:
- Focus groups: Engaging with diverse customer segments to gauge perceptions and preferences.
- Expert opinion: Consulting industry veterans who bring invaluable insights.
- Market research surveys: Gathering data directly from consumers about their future buying intentions.
Although these methods can bring forward some unique insights, it’s important to recognize their limitations as they can be influenced by biases. Therefore, combining these methods with quantitative techniques is often beneficial for a more balanced approach.
Quantitative Forecasting Techniques
On the other side of the coin, quantitative forecasting involves the analysis of numerical data to predict future outcomes. This method is heavily rooted in past sales data and statistical analysis, making it easier to validate assumptions. For decision-makers focused on precision and objectivity, quantitative methods can offer a convincing narrative.
Popular quantitative techniques include:
- Moving averages: Smoothing out fluctuations in sales data over specific periods to discern patterns.
- Regression analysis: Identifying relationships between variables to predict future demand based on those associations.
- Exponential smoothing: Utilizing weighted averages of past observations with a diminishing weight applied to older data points.
These techniques provide a level of rigor and backup that many organizations favor, particularly in industries with stable demand patterns.
Time Series Analysis
Time series analysis dives into sales data trends over a specific period. It enables forecasters to identify underlying patterns and seasonal variations. Retailers often use this method around holiday seasons, gauging how previous years’ sales spiked during specific weeks.
Time series methods often include:
- Additive model: Decomposing the data into trend, seasonal, and irregular components, allowing for the understanding of each element.
- Multiplicative model: Assuming that different factors combine to impact demand, allowing for a more dynamic interpretation of data.
Understanding how past decisions correlate with these time-based patterns can significantly improve future tactical business moves.
Causal Forecasting Models
Causal forecasting models look for relationships between demand and other influencing factors, such as economic indicators or marketing campaigns. For instance, a company may observe that purchasing behavior tends to increase during specific promotional periods or when consumer confidence rises.
These models require identifying the various elements that influence demand, and they can utilize techniques such as:
- Regression analysis: As discussed earlier, determining how different variables interact can be crucial.
- Leading indicators: Using indicators that tend to change before demand shifts to predict upcoming demands.
Causal models provide a more holistic view of the market, allowing organizations to strategize beyond mere sales data.
Tools and Technologies for Demand Forecasting
Demand forecasting is akin to holding a crystal ball for businesses trying to predict how well products will fly off the shelves. In this digital age, it becomes even more important to leverage the right tools and technologies. They not only offer the ability to gather data but also to analyze it in ways that drive strategic decisions.
When organizations invest in demand forecasting tools, it's not just about keeping pace; it's about gaining a competitive edge. The benefits of modern tools extend beyond mere number crunching. They support better inventory management, optimize resource allocation, and ensure alignment with market dynamics. As companies adopt these technologies, they should consider not only the initial cost but also the ongoing enhancements that keep their forecasting sharp.
"Forecasting is about managing uncertainty and mitigating risk. The right tools can help navigate this complex landscape."
Software Solutions Overview
A variety of software solutions are available today, each designed to cater to different aspects of demand forecasting. Some popular options include tools like Oracle Demantra, which excels in statistical forecasting and demand collaboration, or SAP Integrated Business Planning, known for its expansive capabilities in supply chain planning. These software platforms introduce automation and streamline processes that once required tedious manual efforts.
Key advantages of utilizing dedicated forecasting software include:
- Enhanced Accuracy: Advanced algorithms provide more precise forecasts.
- Time-Saving Automation: Automating data entry and calculations allows employees to focus on strategic tasks.
- User-Friendly Interfaces: Many modern tools offer intuitive dashboards that simplify the complexities of data.
- Collaboration Features: Facilitating communication between departments can lead to more synchronized efforts.
Integration with ERP Systems
Integrating demand forecasting tools with established Enterprise Resource Planning (ERP) systems is essential for a seamless flow of information. For instance, if a business uses Microsoft Dynamics 365, incorporating forecasting tools provides a holistic view of operations. This integration ensures that forecasts align closely with current inventory levels, sales trends, and customer demands.
The benefits of this integration include:
- Real-Time Data: Instant access to updated information is crucial for making timely decisions.
- Improved Resource Utilization: Enhanced visibility helps in optimizing stock levels and resources.
- Consistency Across Departments: Shared data reduces the chances of miscommunication between teams, leading to a more efficient workflow.
Real-Time Data Analytics
Incorporating real-time data analytics into demand forecasting is like having a pulse on the market. Being able to analyze data as it comes in allows businesses to swiftly adapt to changing market conditions. Tools that facilitate real-time analytics can utilize cloud computing, big data processing, and machine learning techniques that reduce latency and enhance responsiveness.
Some benefits of leveraging real-time data include:
- Immediate Insights: Fast access to trends helps in adjusting strategies promptly.
- Better Customer Understanding: Analysing buying patterns can reveal valuable customer preferences.
- Proactive Decision-Making: Identifying potential issues before they escalate allows organizations to be one step ahead.
By adopting these tools and technologies, businesses enhance their forecasting capabilities. This not only drives better decision-making but also fosters a culture of continuous improvement. The journey towards achieving effective demand forecasting is paved with the right tools that lead to data-driven strategies.
Challenges in Demand Planning Forecasting
Navigating the currents of demand planning forecasting is no easy feat. Organizations today are caught in a whirlwind of customer expectations, fluctuating market trends, and ever-evolving technologies. Understanding the challenges in demand planning isn’t just helpful; it’s essential for built strategies that are resilient and effective.
One major hurdle organizations face is data quality issues. Poor or inaccurate data can lead to misguided forecasts, causing inventory piles up or stockouts that can tarnish a company’s brand. For instance, if a retail company relies on historical sales data without considering recent market shifts, it might end up overstocking seasonal items that no longer attract consumers. Thus, ensuring data cleanliness and relevance becomes paramount.
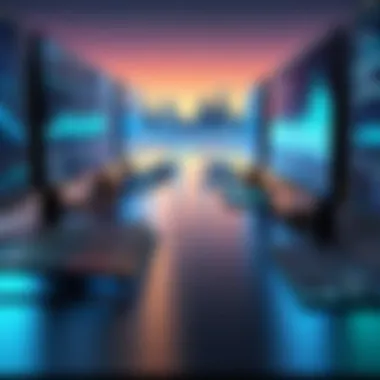
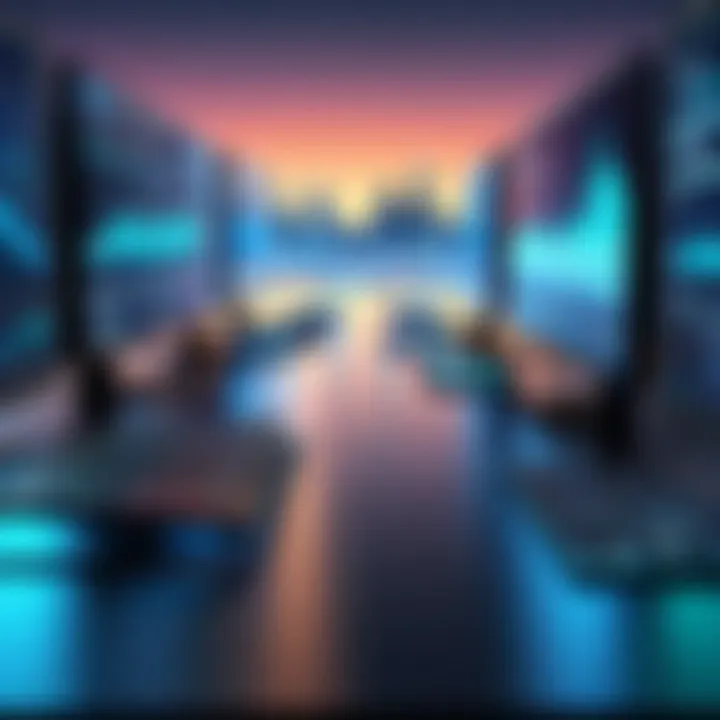
Another point of concern is market volatility. The market can change on a dime, influenced by political, economic, or social factors. A great example would be the textile industry, where fluctuations in cotton prices can suddenly inflate costs, leading to unexpected price changes for consumers. Forecasts based solely on historical trends can mislead companies, making them unprepared to cope with sudden changes in demand.
Lastly, there’s the challenge of collaboration across departments. In many organizations, different departments operate in silos, leading to fragmented perspectives on demand. Sales teams may have their finger on the pulse of customer sentiment, while supply chains tighten their grips based on their own data. When departments don’t share insights or align strategies, the forecasts may miss the mark. As a result, businesses could end up overestimating or underestimating demands, which is less than ideal.
Effective demand planning requires a concerted effort across the organization, sharing insights and aligning strategies to create a coherent forecasting approach.
Addressing these challenges is more than just fixing a leaky faucet; it involves rethinking and restructuring how organizations perceive and process demand forecasting altogether.
Data Quality Issues
Data quality can make or break a forecast. Errors, inconsistencies, or outdated information can skew predictions, leading firms to make decisions based on shaky ground. High-quality data is like a reliable compass that guides businesses through the fog of uncertainty. To tackle this, consider the following:
- Routine Data Audits: Regularly checking the integrity of data can help to identify inaccuracies before they lead to large-scale ramifications.
- Utilize Advanced Tools: Software solutions, such as those from Salesforce or SAP, can aid in data cleansing and ensure consistency.
- Train Employees: Ensuring staff understands the importance of data quality encourages a culture of continuous improvement.
With these steps, organizations can improve their data foundations, enhancing the overall reliability of their forecasts.
Market Volatility
Market volatility introduces a layer of complexity in demand forecasting that can feel like trying to juggle while riding a unicycle. The unpredictable nature of markets can stem from various sources, including:
- Economic Changes: Shifts in the economy often impact consumer spending, disrupting expected demand cycles.
- Competitive Dynamics: New entrants into the market or aggressive pricing strategies from competitors can change demand overnight.
- Consumer Behavior Trends: With social media influencing buying habits, trends can rise and fall rapidly, leaving businesses scrambling to catch up.
Organizations should embrace agility by:
- Adopting Real-Time Analytics: Leveraging tools that provide live updates on market conditions will allow for swifter adjustments to forecasts.
- Scenario Planning: Assessing multiple scenarios for different market conditions can prepare businesses to pivot when necessary.
Collaboration Across Departments
Silos within organizations can lead to disjointed approaches to demand forecasting. Without collaboration, teams may end up with varied data interpretations, resulting in less reliable forecasts. To foster collaboration:
- Regular Meetings: Establish a cadence for interdepartmental meetings to share insights and discuss demand forecasts.
- Shared Technology Platforms: Use integrated software where all departments can upload data and communicate seamlessly.
- Cross-Functional Teams: Creating teams that include members from various departments can ensure a well-rounded approach to demand planning.
Overcoming these challenges requires a proactive and integrated mindset. By addressing data quality, adapting to market volatility, and fostering collaboration, organizations can enhance the effectiveness of their demand planning forecasting efforts.
Best Practices for Effective Demand Planning
Demand planning isn't just a routine task; it's the lifeblood of any organization that aims to meet customer needs effectively while maximizing resources. Following best practices in this area is essential for businesses to optimize their supply chain management, enhance customer satisfaction, and drive overall profitability. By embracing these practices, organizations can align their operational activities more closely with market demands, ensuring they stay ahead of the competition.
Regular Review and Adjustment of Forecasts
One of the cornerstones of effective demand planning is the regular review and adjustment of forecasts. Things change—consumer preferences fluctuate, and unexpected events can shake up market stability. A static forecast can quickly turn into a recipe for disaster. Instead, organizations should establish a schedule for revisiting their forecasts. This could be monthly, quarterly, or in closer intervals, depending on how volatile the market is.
By regularly analyzing actual sales against forecasts, a company can spot trends and discrepancies early on. For instance, if a consumer electronics company finds that sales of a new smartphone are significantly lower than anticipated, this could signal the need to adjust production schedules to avoid excess inventory. This proactive approach not only helps in maximizing sales but also aids in preventing cash flow issues that arise from overstocking.
Cross-Functional Collaboration Techniques
Collaboration across departments is vital. Often, demand planning efforts fall short because stakeholders work in silos. To combat this, fostering cross-functional collaboration is crucial. Involving teams from sales, marketing, finance, and operations ensures that everyone is working toward a shared objective. Each department has its insights and data that can inform the demand planning process.
For example, a marketing team might be gearing up for a campaign that could significantly boost product awareness. By keeping the supply chain team in the loop, they can better prepare for the expected increase in demand. Similarly, sales personnel, who have firsthand experience with customer interactions, can provide feedback on buyer behavior that might not be captured through traditional forecasting methods. This holistic view paves the way for a more accurate demand forecast, reducing the risk of stockouts and subsequent lost sales.
Utilizing Technology for Enhancements
In an era where technology reigns supreme, tapping into the right tools can significantly enhance demand planning efforts. Utilizing advanced software solutions such as forecasting tools or analytical platforms can streamline data collection and analysis, offering greater accuracy in predictions. For instance, a business can implement AI-driven tools that can process vast amounts of data quickly and provide insights that were previously unattainable.
Moreover, integrating these tools with existing Enterprise Resource Planning (ERP) systems promotes seamless information flow. This connectivity allows for real-time data updates, which means decisions can be based on the most current information available. As a result, companies can react faster to market changes, aligning their inventory levels more closely with actual demand.
Consider companies like Amazon, which leverage advanced algorithms and machine learning to forecast demand with impressive accuracy. By following suit, other businesses can improve their efficiency and responsiveness in the marketplace.
"In the world of demand planning, agility is key. Regular adjustments, collaboration, and technology can be transformational."
In summary, the best practices outlined above—regular reviews, cross-departmental collaboration, and technology utilization—are not mere suggestions; they are necessities for businesses aiming for success in today's dynamic market landscape. Embracing these practices leads to more informed decisions, better resource allocation, and ultimately, enhanced customer satisfaction.
The Role of Customer Insights in Forecasting
In today’s fast-paced and ever-changing market, understanding customer behavior can make or break a company’s demand planning strategy. Customer insights serve as a vital pillar for forecast accuracy, helping organizations better align their inventory and production schedules with real market needs. When demand planners tap into the thoughts and preferences of their customers, they turn abstract sales data into actionable intelligence. This section will delve into the essential role customer insights play in refining forecasting processes.
Gathering Customer Feedback
One of the most direct ways to gather customer insights is through effective feedback mechanisms. Companies have historically relied on surveys, focus groups, and interviews. However, the landscape has evolved.
Now, many organizations utilize online platforms and social media to glean real-time insights. For instance, a retail brand can analyze comments and reviews on platforms like Facebook or Reddit to understand customer sentiments about a product. These insights can reveal not just what customers like or dislike, but also emerging trends that may not yet be pervasive.
- Surveys: Conducting targeted surveys post-purchase can yield valuable insights. Ask specific questions about how a product fits in the customer's life, not just its functionality.
- Social Media Listening: Tools that monitor customer interactions on social channels offer an organic feel of current trends.
- Usability Testing: Observing customers as they interact with a product can reveal pain points that might affect demand.
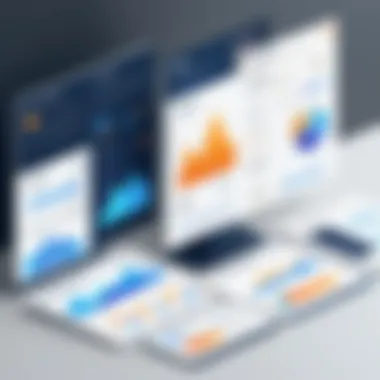
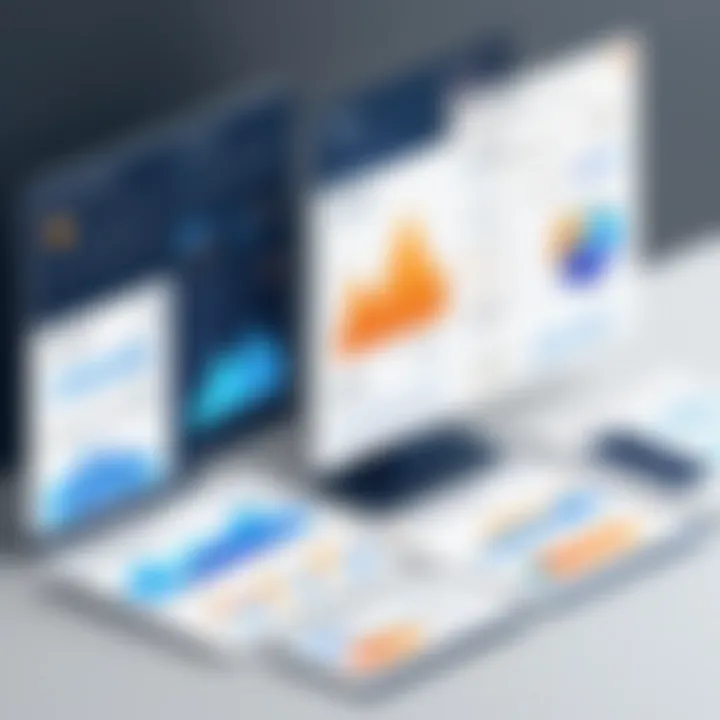
By implementing these techniques, organizations can create a feedback loop that continuously informs forecasting models.
Analyzing Consumer Behavior Trends
Gathered insights are only as useful as the analysis that follows. Analyzing consumer behavior trends involves looking at feedback through a critical lens to derive patterns and predictions.
Breaking down the data into more digestible forms helps identify key indicators that influence buying decisions. A few techniques in this area include:
- Segmentation Analysis: Not all customers are created equal. By segmenting the customer base, a business can tailor its forecasting around specific groups. For example, Gen Z prefers sustainability, which may lead to increased demand for eco-friendly products.
- Purchase History Trends: Examining past purchase behaviors can help predict future buying patterns. If a beverage company notices spikes in demand for certain flavors during summer months, they can anticipate inventory needs accordingly.
- Seasonality and Trends: Recognizing seasonal buying rhythms can be crucial. Consumers might splurge on gifts during the holidays, or fresh produce might see increased sales in the spring. Using historical data to forecast future needs is essential.
"Incorporating customer insights guides demand planners toward a deeper understanding of market conditions, enabling them to adapt swiftly and effectively."
Ultimately, leveraging these insights brings clarity to the ambiguity inherent in forecasting. When organizations prioritize customer feedback and behavior analysis, they are better equipped to anticipate shifts in demand. This not only enhances operational efficiency but also strengthens customer loyalty by meeting needs proactively.
Case Studies in Demand Planning Success
Understanding demand planning forecasting is not merely an academic exercise; it’s an essential practice that underpins successful business operations. By examining real-world case studies, decision-makers can glean vital insights into strategies that lead to effective demand forecasts. These cases illustrate the triumphs and pitfalls encountered by organizations in their forecasting journeys, serving as guiding lights for future endeavors.
Industry-Specific Applications
In varied industries, the application of demand forecasting varies significantly. Exploring how specific sectors implement demand planning reveals unique challenges and successes, contributing to a richer understanding of the topic.
- Retail: For a well-known fashion retailer, integrating seasonal trends with data analytics improved their ability to predict customer preferences. By applying advanced statistical techniques alongside qualitative insights, they reduced excess stock by 35% within one year.
- Food and Beverage: A beverage company faced volatile demand due to rapidly changing consumer trends. They established real-time feedback from distributors and adjusted their forecasts based on this real-time data. This adaptive approach allowed them to minimize waste and align production with current market demands smoothly.
- Technology Sector: A tech giant used predictive analytics to manage the lifecycle of its products. By aligning supply chain activities with anticipated demand shifts based on historical sales patterns, they improved their time-to-market by 20%. This was particularly crucial during product launches, which often require precise inventory levels.
These industry-specific applications demonstrate how tailored solutions can greatly enhance the effectiveness of demand forecasting initiatives. By learning from the successes of others, organizations can craft strategies that are bespoke to their needs.
Lessons Learned from Failed Forecasts
Not all forecasting attempts yield fruitful outcomes. Understanding mistakes can be just as enlightening as studying success stories. Failures often bring to light critical lessons that can reshape future forecasting strategies.
One notable case involved a consumer electronics company that overestimated the popularity of a new device, leading to significant overproduction.
- Inadequate Market Research: The company relied heavily on sales data from previous models without considering shifts in consumer preferences. The neglect of changing trends in technology caused a disconnect between production and actual consumer interest, resulting in substantial losses.
- Ignoring External Factors: This same company failed to account for macroeconomic factors such as a downturn in consumer spending. They moved forward with their forecasts regardless, demonstrating the need for comprehensive market analysis to inform demand planning. This oversight not only led to financial regret but also damaged their brand reputation among consumers.
These lessons emphasize the necessity for a multifaceted approach in demand forecasting. Understanding external influences and the importance of adaptable strategies can help avoid similar pitfalls.
"In the realm of demand planning, it's crucial to view failures as learning experiences. Each misstep is a stepping stone towards improved forecasting capabilities that can ultimately foster resilience in the face of market fluctuations."
Companies that take these lessons to heart and adjust their strategies will likely find themselves better equipped to handle the unpredictable nature of market demands, ensuring a stronger foothold in their respective industries.
The Future of Demand Planning Forecasting
Looking ahead, demand planning forecasting stands at a crossroads, where innovation meets evolving market needs. As we navigate through the intricacies of supply chains and shifting customer demands, understanding the future of demand planning becomes not just an option but a necessity. This section elaborates on key aspects shaping the trajectory of demand forecasting, underlining its significance in a world that thrives on adaptability and precision.
Emerging Trends in Forecasting Technology
The landscape of forecasting technology is undergoing rapid transformation. Businesses are increasingly relying on sophisticated tools to make sense of vast volumes of data. Here are some trends that are making waves:
- Cloud Computing: Companies are moving their forecasting processes to the cloud. This allows for greater flexibility, accessibility, and data security. Not only can teams collaborate from anywhere, but they can also harness powerful computing capabilities without breaking the bank.
- Big Data Analytics: With more data available than ever before, the integration of big data in forecasting is vital. It helps uncover patterns and trends that traditional methods might overlook. The sifting through complex datasets allows all sorts of insights that enable more accurate predictions.
- IoT Integration: The Internet of Things (IoT) provides real-time data from various sources, from customer behaviors to supply chain operations. This capability lets companies react faster and align their inventory levels with actual demand.
Each of these trends demonstrates how essential it is to stay ahead of the curve. Businesses that embrace these technologies can position themselves to thrive in a fiercely competitive landscape.
The Increasing Role of AI and Machine Learning
Artificial intelligence and machine learning are no longer just buzzwords; they are pivotal components in the supply chain forecasting toolkit. These technologies are reshaping how businesses understand and respond to customer needs, leading to streamlined operations and improved decision-making.
- Enhanced Predictive Accuracy: AI can analyze past demand patterns and external factors with a level of depth that was previously unattainable. This leads to more accurate forecasts, minimizing the risk of overstocking or stockouts.
- Efficiency in Decision Making: By automating routine forecasting tasks, machine learning frees up time for analysts to focus on interpreting results. This shift can result in quicker responses to changing market conditions.
- Personalized Customer Experience: AI systems can analyze individual customer behaviors to predict what products specific segments may need. Companies can thus tailor their offerings to meet those needs, enhancing customer satisfaction.
- Scenario Planning: AI enables organizations to build various demand scenarios based on changing factors—like shifts in consumer preferences or unexpected disruptions in supply chains. This readiness helps mitigate risks associated with unpredictability.
"As we move ahead, the collaboration between human insight and machine intelligence will redefine forecasting effectiveness. Companies that harness these tools will find themselves in a much stronger position."
In summary, the future of demand planning forecasting is not just about reacting to trends but proactively shaping them. The infusion of advanced technologies will ultimately offer businesses greater resilience and a clearer path to achieving their targets. As the demand landscape continues to evolve, understanding these advancements becomes crucial for anyone in the field.
The End
Demand planning forecasting emerges as a cornerstone in the foundation of successful business strategies. In an age where market dynamics shift faster than the blink of an eye, organizations that master the art of demand forecasting gain a definitive edge. The key lies in understanding how accurate and well-timed forecasts can bridge the gap between supply and demand, ultimately driving operational efficiency and enhancing customer satisfaction.
Accurate demand forecasting bolsters inventory management, aligning it with customer expectations. When businesses can predict demand accurately, they can optimize stock levels, reducing instances of both excess inventory and stockouts. This immediacy not only spares resources but also mitigates financial risk, benefiting the bottom line.
Summary of Key Insights
Throughout this comprehensive guide, several critical elements about demand planning forecasting have come to light:
- Integrative Approach: Successful forecasting stitches together data from various sources, enabling a holistic view of market trends. This informs decision-making processes across departments, ensuring alignment in strategy.
- Adoption of Technology: Implementing advanced tools and technologies—like AI and real-time analytics—can elevate forecasting accuracy. Automating data analysis helps to spot patterns that manual methods might overlook.
- Continuous Improvement: Regularly revisiting and adjusting forecasts in response to market fluctuations is vital. Dynamic environments necessitate flexible forecasting methodologies.
"In the world of business, if you're not ahead of the curve, you’re already behind it."
Final Thoughts on Demand Planning
Ultimately, demand planning forecasting is not merely a tactical exercise; it's an avenue for long-term success. For decision-makers, investing time and resources in refining forecasting processes translates directly into enhanced organizational agility. This agility allows companies to anticipate changes, respond proactively, and thrive amidst uncertainty. Staying informed on emerging trends—such as machine learning—even opens up new avenues for predictive precision.
The road to effective demand forecasting is not without its bumps; however, the benefits far outweigh the challenges. By embracing best practices, leveraging technology, and fostering a culture of collaboration, businesses are well-positioned to turn forecasting into a strategic advantage rather than just a necessary chore. The future belongs to those who wisely anticipate what lies ahead.