Understanding Splunk Demo Data: A Comprehensive Guide
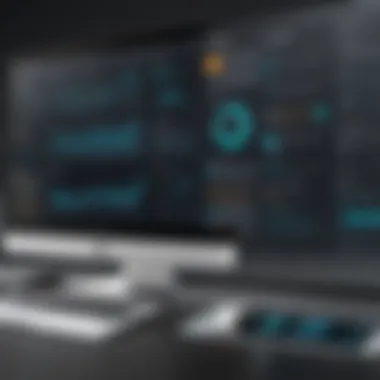
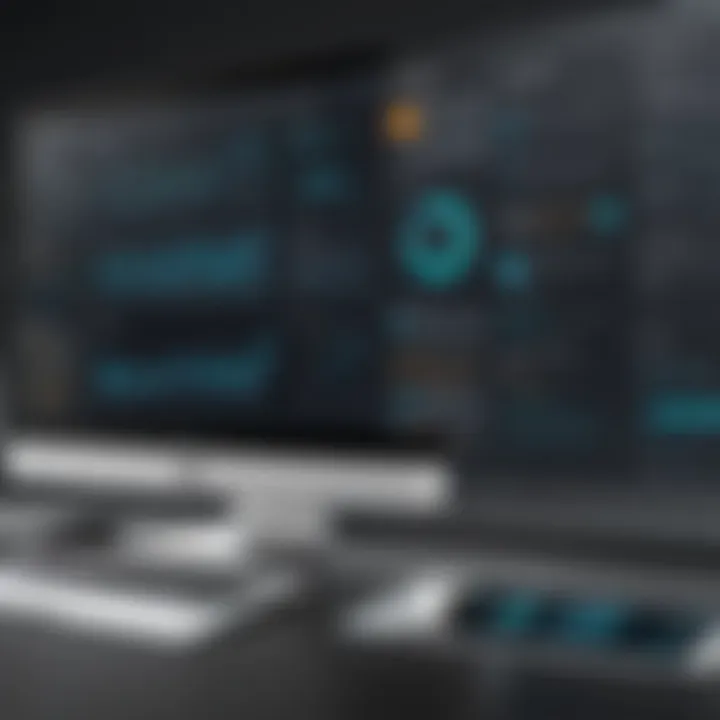
Intro
In todayβs rapidly evolving tech landscape, data is more than just numbers; it is an essential asset that informs decision-making processes across industries. Splunk, a leading platform for operational intelligence, offers capabilities to harness vast amounts of machine-generated data. Central to understanding its potential is exploring Splunk's demo data. This guide aims to provide comprehensive insights into what Splunk demo data encompasses, how it can be leveraged effectively, and its significance in various software applications.
A thoughtful examination of demo data allows stakeholders, from IT professionals to decision-makers, to evaluate Splunk's capabilities thoroughly. Understanding the facets of this demo data can lead to informed decisions that align with business goals and technical requirements.
Key Features and Functionalities
Comprehensive Overview
Splunk demo data is designed to showcase the powerful features of the platform. It provides realistic scenarios that mimic real-world data challenges, enabling users to explore functionalities without deploying live environments. This demo data typically includes sample logs, metrics, and alerts, all crafted to highlight Splunkβs data indexing, search, and visualization capabilities.
The platform also incorporates various data formats, enabling users to see how Splunk performs across different data types. This illustrates not only the versatility of the software but also prepares potential users for an array of applications tailored to specific industry needs.
Target Users
The target audience for Splunk demo data extends beyond data engineers. It includes:
- Decision-makers: Leaders examining how data analytics can optimize operations.
- IT professionals: Technicians seeking to align technical capabilities with business needs.
- Software buyers: Individuals conducting thorough evaluations before purchases.
- Data analysts: Those looking at how Splunk can aid in data interpretation and visualization.
By targeting these groups, Splunk ensures that the demo data addresses the relevant questions for stakeholders, making the evaluation process smoother and more insightful.
Pricing Models and Cost Analysis
Breakdown of Pricing Tiers
Understanding the pricing structure of Splunk is pivotal for conducting a thorough evaluation. The software typically offers several pricing models based on usage, scale, and required features. The tiers often range from free options with limited capabilities to enterprise solutions that provide comprehensive support.
- Free Tier: Ideal for hobbyists or those wishing to explore basic functionalities.
- Essential Tier: Suitable for small-to-medium businesses looking for a balanced feature set.
- Enterprise Tier: Comprehensive package for larger businesses needing extensive data handling and support services.
Additional Costs to Consider
Beyond the basic pricing models, it's crucial to account for potential additional costs:
- Trainig and Support: While the software offers tutorials, formal training sessions may incur extra fees.
- Infrastructure Costs: Users should consider hardware requirements for running Splunk efficiently.
- Add-on Features: Advanced functionalities or integrations could lead to higher expenses.
Engaging with Splunk demo data not only showcases the platformβs offerings but also helps users map out potential financial commitments related to their needs.
"Effective utilization of demo data can significantly enhance the software evaluation process, providing clarity and confidence to stakeholders in their decision-making."
Intro to Splunk Demo Data
The topic of Splunk demo data is essential in grasping the overall capabilities of Splunk as a software tool. This section serves as a foundational aspect of this article, guiding readers through the significance of demo data in real-world applications. Demo data offers an opportunity to evaluate how well Splunk performs in simulating realistic environments without the risk of compromise or failure.
What is Splunk?
Splunk is a powerful platform designed for analyzing machine-generated data. It processes logs and various forms of unstructured data, providing insights that help organizations monitor and understand their IT infrastructure, security posture, and operational performance. The capabilities of Splunk extend beyond mere data collection; it facilitates deep analysis through real-time monitoring, searching, and visualization.
As organizations increasingly generate vast amounts of data daily, the ability to correlate and derive meaningful insights from this data becomes crucial. Splunk emerges as a tool that can sift through noise, allowing enterprises to focus on what's important.
Importance of Demo Data
Demo data plays a crucial role in the effective evaluation and testing of the Splunk software environment. Here are several intrinsic benefits it offers:
- Realistic Testing Scenarios: By using demo data, potential users can simulate their actual data sets, providing a realistic overview of Splunk's functionality and performance.
- User Familiarization: It allows IT professionals to become acquainted with the user interface and features of Splunk without the complexity of real production data.
- Cost-Efficiency: Relying on demo data minimizes the need for costly infrastructure changes or data handling issues in testing phases.
- Risk Mitigation: Users can practice operations on demo data, reducing the risk associated with experimenting on live data.
The engagement with demo data helps unravel the potential of Splunk while providing insights into the softwareβs operational strengths and weaknesses. This practice not only aids informed decision-making but also enhances skill development among team members who handle data analytics.
"Demo data is like a sandbox. It allows developers and analysts to explore without the consequences of production data."
Types of Splunk Demo Data
Understanding the various types of Splunk demo data is crucial for effective utilization. Each type serves distinct analytical purposes and aids users in simulating real-world scenarios. Being familiar with these data types enhances decision-making, especially for professionals evaluating Splunk's capabilities. Here are the primary types of demo data you should consider:
Log Files
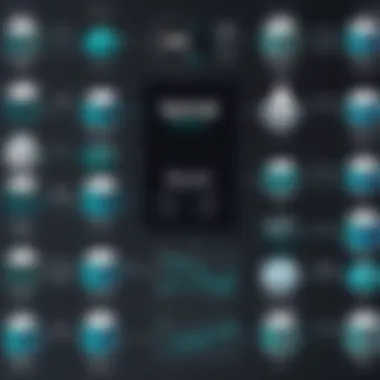
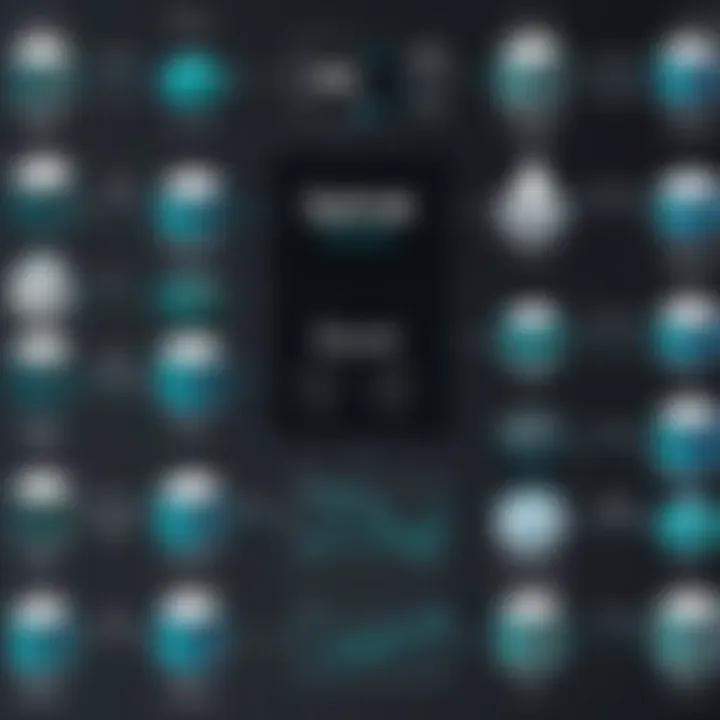
Log files are a foundational component of Splunk demo data. They record various events and transactions generated by systems, applications, or devices. Typically, log files include information such as timestamps, severity levels, and message content. This comprehensive logging enables users to analyze system performance and troubleshoot issues effectively.
The importance of log files cannot be understated. They provide insights into operational discrepancies and security events. Organizations can simulate common scenarios by leveraging synthetic log data. This assists IT teams in understanding how to utilize Splunk for error tracking and compliance auditing. Users can also examine data patterns over time, allowing for informed decision-making.
Metrics Data
Metrics data in Splunk is centered around quantitative measurements over time, reflecting system or application performance. Unlike log files, which capture events, metrics data aggregates measurements such as CPU usage, memory consumption, and network throughput. This data type is essential for monitoring system health and performance trends.
Metrics allow users to visualize performance metrics in real-time. Splunkβs ability to analyze metrics data empowers organizations to respond proactively to potential issues. By using demo metrics data, teams can fine-tune alerts for deviations from expected performance, making it vital for maintaining operational efficiency. Users exploring this data type can improve system monitoring strategies and engage in deeper performance analysis.
Event Data
Event data captures specific occurrences relevant to a business's operations and system functions. This data encompasses information such as user interactions, transaction logs, and security events. Event data is critical for understanding user behavior and system dynamics on a granular level.
Using event data enhances analytical capabilities within Splunk. Organizations can dissect user sessions and interactions to gather insights on product usage and service effectiveness. Moreover, event data combined with appropriate analytics can help identify potential security vulnerabilities or operational inefficiencies. By analyzing this data type in demo environments, users can strengthen their preventive measures and refine their operational strategies.
"Each type of Splunk demo data provides unique insights that can significantly improve operational decision-making."
Creating a Splunk Demo Environment
Creating a Splunk demo environment is fundamental for anyone aiming to fully explore the capabilities of Splunk. This section addresses why it's essential and what to consider when setting this up. The demo environment allows users to familiarize themselves with the user interface, simulate data analysis, and test various functions before committing to a production system. Understanding how to create this environment effectively can save significant time and resources in a real-world application.
System Requirements
Setting up a Splunk demo environment requires meeting specific system requirements. This ensures that Splunk runs smoothly and can handle the demo data effectively. Here are the primary requirements:
- Operating System: Splunk is supported on various operating systems, including Windows and Linux distributions. Choose one that suits your current infrastructure.
- Memory: At least 8 GB of RAM is recommended for optimal performance. More is better, especially when dealing with larger datasets.
- CPU: A multi-core processor will enhance Splunk's ability to process data in real time without lag.
- Disk Space: Adequate storage is necessary. A minimum of 20 GB is advisable to accommodate the installation and demo datasets.
- Network Connectivity: Ensure that your system can access the internet to download the software and any needed updates.
Meeting these requirements ensures that users enjoy a seamless experience when navigating Splunk with demo data.
Installation Process
Once the system requirements have been satisfied, the next step is the installation process. This can vary slightly depending on the operating system, but the core steps remain largely consistent. Here is a simple guide for installation:
- Download Splunk: Visit the official Splunk website. Select the version that aligns with your system and download the appropriate installer.
- Run the Installer: After downloading, locate the installer file and execute it. For Windows, this typically means double-clicking the file. For Linux, you may need terminal commands.
- Follow the Prompts: The installation wizard will guide you through the setup. Accept the default settings unless there is a specific need to alter them.
- Choose the Installation Type: Select whether you want a standalone installation or to set up a distributed environment.
- Log In: After installation, you will be prompted to create an admin username and password. This sets up the initial administrative access to your Splunk instance.
- Load Demo Data: Once the setup is complete, you can import Splunk demo data either through the web interface or by using command line options.
By following these steps, you can establish a functional Splunk demo environment that will be invaluable for testing and learning. This practical experience lays the groundwork for effective data analysis and system management.
Navigating Splunk Interface with Demo Data
Navigating the Splunk interface effectively is crucial for users who are exploring demo data. This knowledge allows users to utilize the platform's capabilities to their fullest potential. A well-structured understanding of the interface enhances the learning experience, enabling quicker diagnosis of issues, discovery of insights, and overall operational efficiency.
Various elements of the Splunk interface should be mastered. This includes dashboards, search functionalities, and the ability to visualize data. Understanding how to navigate these elements allows users to evaluate the performance of Splunk through interactive and visual means. Moreover, familiarity with these tools can significantly enhance the analysis of demo data.
Dashboard Overview
The dashboard serves as the primary workspace in Splunk. It is an interactive display comprising various visual elements such as graphs and tables, which are tailored to represent data insights effectively. A dashboard can showcase multiple data sources, creating a holistic view of system performance and security events.
When navigating the dashboard, users should note the significance of layout and data arrangement. For instance:
- Widgets: These are the fundamental building blocks. They can represent different types of data visualizations, including pie charts, line graphs, or statistics.
- Panels: Grouping widgets logically into panels allows for better organization of information.
- Filters: Users can apply filters to refine data displayed on the dashboard, enabling the focus on specific metrics or timeframes.
The effectiveness of dashboards in a demo environment can be prominent when evaluating potential use cases or system performance trends. Users gain deeper insights into the behavior of demo data through continuous interaction with dashboard components.
Search Functionality
The search functionality is one of the hallmark features of Splunk. It allows users to retrieve and analyze data from various sources. Mastering this functionality simplifies querying demo data efficiently, which leverages Splunk's powerful search processing language (SPL).
When utilizing search features, users should pay attention to:
- Search Bar: This is where users execute their queries. Understanding basic SPL syntax can enhance search effectiveness.
- Search Commands: Commands like , , and are essential for retrieving data accurately.
- Time Range Picker: This allows users to define specific time periods for data retrieval. Accurate time selection is critical for contextually rich analysis.
For example:
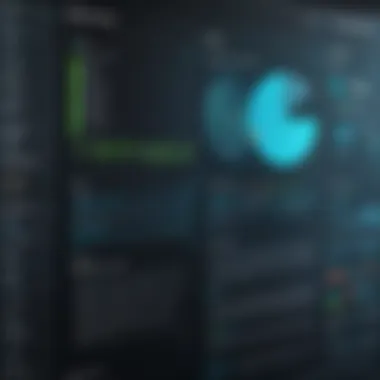
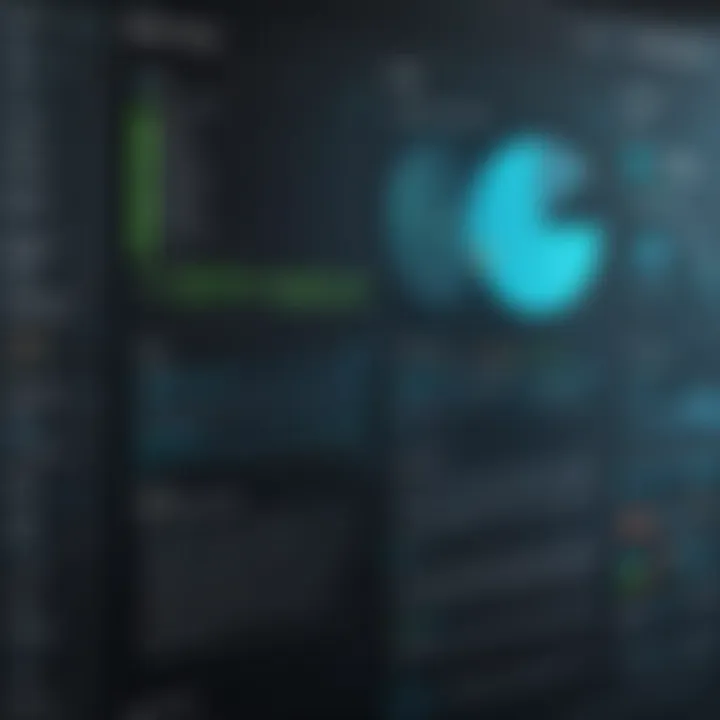
This query finds the count of logs grouped by event type within the demo data index.
These functionalities will aid users in uncovering patterns and anomalies within demo data, contributing to a better understanding of Splunk's capabilities. It is through diligent navigation and effective use of search tools that users can maximize their evaluations of demo data's potential.
Practical Use Cases of Splunk Demo Data
Understanding the practical applications of Splunk demo data serves as a critical aspect in grasping how businesses can leverage this tool for enhanced decision-making. Splunk allows organizations to simulate various scenarios, enabling better preparedness and informed choices. It is about seeing how hypothetical situations translate to real-world challenges and successes.
Operational Monitoring
One prominent use case of Splunk demo data is operational monitoring. Companies often face difficulties in pinpointing system bottlenecks or unusual behavior that may indicate underlying issues. Splunkβs capabilities allow IT professionals to monitor operations continuously. This monitoring includes not just logs from applications but also metrics from network devices and databases. By utilizing demo data:
- Trends and Patterns: Insights can be derived about typical system performance, identification of irregular patterns, and forecasting possible outages.
- Incident Management: Quick responses to incidents become feasible. Testing scenarios with demo data can help in understanding what might go wrong and how to resolve such issues.
- Alert Configurations: With a practical use of demo data, users can experiment with alert conditions to ensure relevant notifications are generated without being overwhelmed by noise.
These aspects highlight the necessity of robust operational monitoring in todayβs complex environments.
Security Information and Event Management (SIEM)
The realm of security is another area where Splunk demo data reveals its potential. Security Information and Event Management (SIEM) helps organizations in managing security threats effectively. Demo data can simulate various security events, from network intrusions to insider threats. This serves various pivotal functions:
- Threat Detection: By examining demo datasets, security teams can develop processes to identify potential threats quickly, even before they manifest in the real world.
- Compliance Testing: Organizations must adhere to multiple compliance regulations. Using demo data can aid organizations in testing their security controls against these standards without risking real data breaches.
- Incident Response Drills: Practicing incident response using realistic data helps in refining and enhancing the team's responsiveness during actual threats.
In both operational monitoring and security management, the practical use of Splunk demo data arms organizations with valuable insights and preparedness strategies. This foundation ultimately leads to better resource utilization and risk mitigation.
Analyzing Demo Data Effectively
Analyzing demo data is crucial for extracting meaningful insights and drawing actionable conclusions in any data-driven environment. Splunk's demo data offers a platform for users to familiarize themselves with the toolβs capabilities and to perform analytical tasks without the complexities of real-world data complications. Effectively using this demo data can lead to a clearer understanding of how Splunk operates, as well as its potential applications in various business scenarios.
Data Visualization Techniques
Data visualization is the process of representing data in a graphical format. It allows stakeholders to observe patterns, trends, and anomalies in a visual context. With Splunk demo data, users can employ various visualization techniques to communicate their findings clearly and effectively.
Some common visualization techniques include:
- Charts and Graphs: These are basic yet effective tools for showcasing data trends over time. Line graphs can depict changes, while bar charts can compare various data sets.
- Heat Maps: Heat maps provide a color-coded overview of data points. They are particularly effective for spotting areas of high activity or problems.
- Dashboards: A dashboard integrates multiple visualizations in one screen, enabling real-time monitoring and quick data interpretation.
Utilizing these techniques enhances the user experience, making data easier to grasp and decisions more informed. Clear and intuitive visual representations foster collaboration among team members as they discuss findings.
Generating Reports
Generating reports is another key aspect of analyzing demo data effectively. It involves summarizing the insights derived from the demo data and presenting them in a structured format. Splunk provides tools to create comprehensive reports that can be tailored to specific needs.
When generating reports with Splunk demo data, consider the following:
- Customizable Templates: Splunk allows users to use and modify templates that fit various reporting requirements. This flexibility makes it easier to present information relevant to specific stakeholders.
- Data Drill-Downs: Reports can be inclusive of drill-down capabilities. This means users can click into summary data to explore more detailed underlying information.
- Automated Schedule: It is possible to automate report generation based on a certain frequency. Regular updates can ensure that all stakeholders have the latest information to inform their decisions.
Reports generated from Splunk demo data can greatly enhance knowledge sharing across teams, ensuring that everyone is aligned on data insights. As a result, decision-makers can act on accurate and timely information, driving their organizations forward effectively.
"Data visualization and reporting are not just tools; they are essential for deriving true value from data."
Benefits of Using Splunk Demo Data
Utilizing Splunk demo data is crucial for both organizations and individuals aiming to maximize the potential of data analytics. This method allows for exploration without the overhead of affecting live data systems. The benefits span several facets, notably realistic testing scenarios and skill development.
Realistic Testing Scenarios
With Splunk demo data, users can simulate a wide variety of operational contexts. This aspect is particularly significant for testing how systems will respond under various conditions. Users can explore different types of log files and metrics, examining potential system behaviors without risking disruption to live environments.
By utilizing realistic data sets, teams can thoroughly evaluate the capabilities of their systems. For example, they can test security measures by simulating attacks or anomalies in a controlled setting. This aspect not only aids in understanding system limits, but also in ensuring readiness for unexpected incidents that may occur in real-world scenarios.
Moreover, using demo data helps in adjusting configurations and testing new features before deploying them. Testing in such a controlled environment can lead to discovering optimal configurations that enhance performance.
Skill Development
Engagement with Splunk demo data offers significant opportunities for skill development. As IT professionals interact with this data, they enhance their ability to analyze and extract insights effectively. The process of working through various data types aids in understanding the underlying mechanics of data handling, from ingestion to visualization.
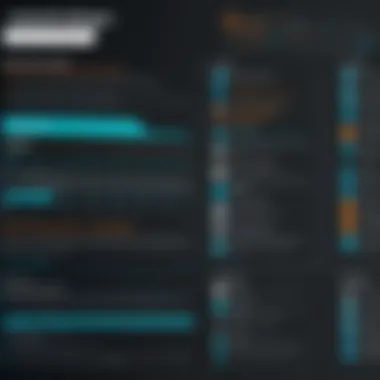
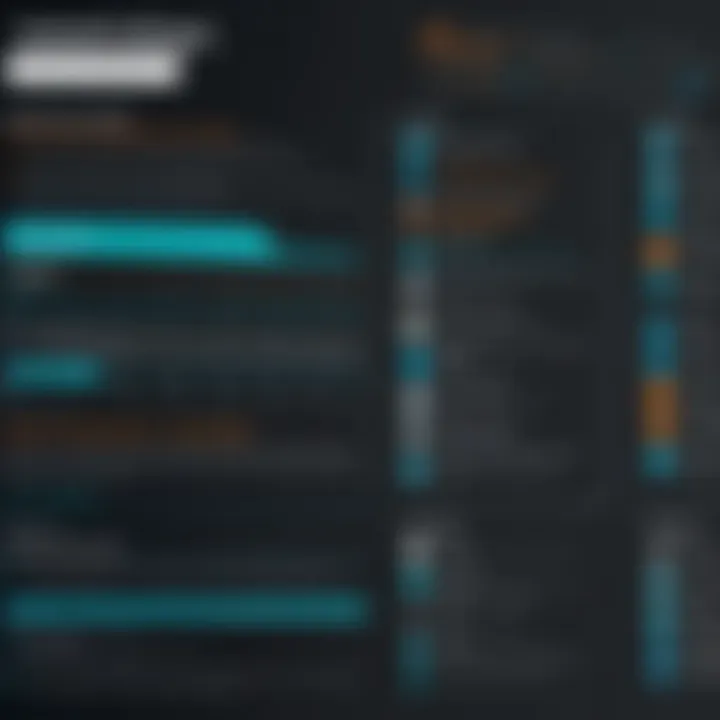
Experimentation with demo data cultivates familiarity with Splunk's various functionalities, which is invaluable for both new and seasoned users. Newcomers to Splunk can practice different search commands and data visualizations, thus building confidence in their abilities. Focused practice leads to a deeper comprehension of how to manipulate data for specific use casesβknowledge that can be pivotal in their careers.
In addition, conducting detailed analysis on demo data allows professionals to strengthen their analytical thinking and problem-solving skills. Being adept in these areas is essential in today's data-driven landscapes, where decisions are increasingly predicated on insightful data interpretations.
"Harnessing demo data not only amplifies oneβs technical skills but also forges a practical understanding of analytics that is essential in strategic decision-making contexts."
In summary, the benefits of using Splunk demo data are multifaceted. They provide organizations with the means to test systems realistically while simultaneously fostering the skill sets of the individuals handling this data. This dual advantage allows for robust analysis and better preparedness for future challenges.
Common Challenges with Demo Data
Navigating the world of Splunk demo data is not without its hurdles. Understanding these challenges is vital for anyone looking to utilize Splunk effectively. It aids in recognizing how to maximize benefits while minimizing potential risks. Analyzing demo data is crucial for demonstrating the features of the software, but it comes with certain limitations and complications that need careful consideration.
Data Relevance
The relevance of demo data to real-world scenarios is a paramount concern. When implementing Splunk demo data, it can often be tempting to assume that all available data is pertinent. However, demo data may not always reflect current conditions or specific organizational scenarios. The context in which the demo data is used must align with genuine operational needs.
- Outdated Data: Often, demo datasets are not frequently updated. This may lead to misinformed decision-making based on old information.
- Generic Data: Demo datasets can be overly generic, lacking specific characteristics that an organization needs. This disconnect can hinder a proper evaluation of the software's efficacy for a particular business case.
Understanding data relevance is crucial for ensuring the insights generated during the trial phase translate into real-world applicability.
Understanding Limitations
Recognizing the limitations of demo data is also essential to properly leverage its utility in Splunk. Without understanding these shortcomings, users may struggle to interpret data effectively.
Some common limitations include:
- Scale Issues: Demo environments may not represent the data scale typically encountered in production environments. Businesses risk underestimating resource needs for data processing when leveraging smaller datasets.
- Functionality Gaps: Certain features may not be available or fully operational within demo datasets. This can lead to a skewed understanding of what Splunk can actually do in a production setting.
In many situations, demo data serves as a starting point for assessments rather than a definitive proof of capability.
"Understanding the limitations of demo data is necessary for informed decision-making. Misrepresentation can lead to misguided expectations."
To effectively address these challenges, users should supplement demo experiences with practical insights and data relevant to their specific situations. This helps ensure that users achieve a comprehensive understanding of Splunk's capabilities and adopt it for their operational needs.
Future Trends in Data Simulation
In the frantic pace of the digital age, data simulation has emerged as a critical mechanism for businesses to understand and harness data effectively. This section explores the future trends in data simulation, particularly focusing on the enhanced integration of artificial intelligence and the emphasis on real-time data analysis. As industries grow increasingly reliant on data for decision-making, understanding these advancements is essential for decision-makers, IT professionals, and entrepreneurs.
Enhanced AI Integration
Artificial Intelligence is reshaping the landscape of data simulation significantly. AI models are becoming more sophisticated in their ability to mimic real-world scenarios. This capability allows organizations to test hypotheses and gather insights before deploying changes to live systems. By leveraging machine learning algorithms, businesses can simulate various data environments and predict outcomes with higher accuracy. This is especially valuable in complex systems where multiple variables interact.
- Adaptive Learning: AI systems can learn from new data inputs, providing updated simulations based on emerging trends.
- Predictive Analytics: Organizations can build models that anticipate future states based on current and historical data.
- Cost Efficiency: By using AI to streamline processes and reduce the need for extensive manual setups, businesses save time and resources.
AI integration into data simulation is not merely a trend; it represents a fundamental shift in how organizations approach data. With enhanced capabilities, companies can experiment and innovate within their operational frameworks more seamlessly.
Real-Time Data Analysis
Real-time data analysis is another critical trend that will dictate the future of data simulation. The ability to analyze data as it comes into a system provides organizations with immediate insights and opportunities for action.
"The power of real-time data analysis lies in its ability to inform decisions at the speed of thought."
Key elements of this trend include:
- Instant Decision-Making: Businesses can respond to changes in their environments almost instantaneously, which is crucial in sectors like finance and healthcare.
- Operational Efficiency: Through live data analysis, organizations can identify bottlenecks or inefficiencies in processes and rectify them immediately.
- Enhanced User Experiences: By understanding user behavior in real time, companies can tailor their products and services, improving overall customer satisfaction.
In summary, the integration of AI and real-time data analysis into data simulation markedly enhances an organization's capability to utilize its data. These trends represent the forefront of technology in data analysis and will be instrumental for any organization aiming to secure a competitive edge. As we continue to explore the implications of these advancements, it is clear that the landscape of data analytics will evolve, offering new opportunities and challenges.
The End
In the realm of data analytics, the significance of understanding demo data cannot be overstated. This article emphasizes how Splunk demo data serves as a critical tool for professionals seeking to evaluate software capabilities effectively. Through a thorough examination of its uses and benefits, we can appreciate how demo data not only aids in practical assessment but also enhances the overall proficiency in utilizing Splunk tools.
Key Takeaways
- Value of Demo Data: Splunk demo data provides a realistic platform for testing and familiarizing oneself with the software's features.
- Key Applications: It supports various functions, including operational monitoring and security management, making it indispensable in practical scenarios.
- Challenges: Recognizing the limitations and relevance of demo data is crucial for making informed decisions.
- Future Trends: Staying updated with advancements, such as AI integration and real-time analysis, can enhance the capabilities of data utilization.
Next Steps for Users
- Engage with Demo Data: Users should actively engage with the provided demo datasets to deepen their understanding of Splunk's functionalities.
- Participate in Forums: Visit platforms like Reddit and Facebook to connect with other users and share experiences.
- Explore Resources: Consult additional resources such as Wikipedia for broader knowledge about data analytics and Splunk. Take the initiative to stay informed on emerging trends and practices in the realm of data visualization and analysis.
"Understanding Splunk demo data opens up a resourceful experience for professionals to enhance their technical capabilities."
By following these next steps, users can maximize their proficiency and ensure they are making the most informed decisions regarding software applications.