Understanding Data Analytics Software in Business
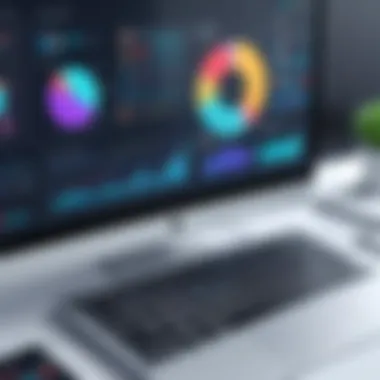
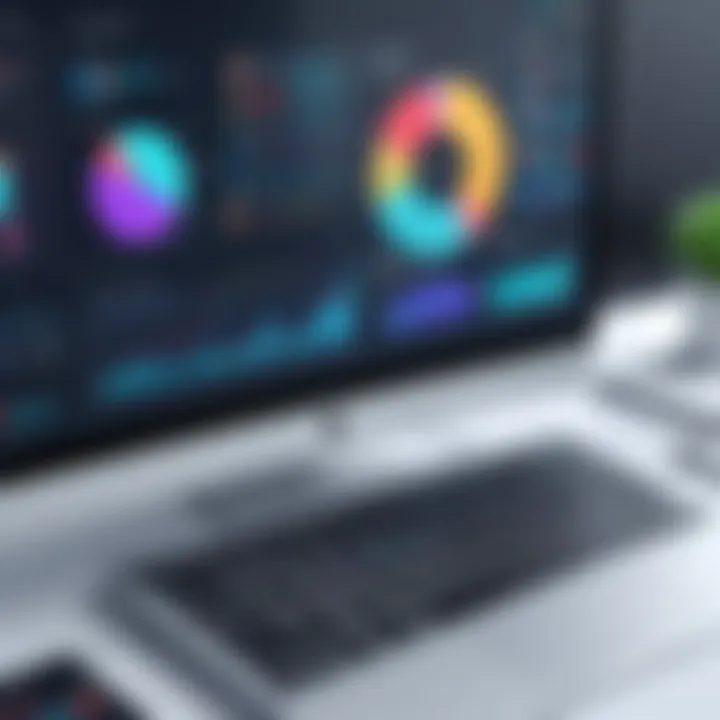
Intro
In today’s fast-paced digital world, data analytics software stands as a cornerstone for businesses aiming to leverage information to make insightful decisions. The importance of understanding such software cannot be understated, as its role shapes strategies and operational outcomes. Data analytics tools help organizations sift through vast amounts of data, transforming raw numbers into actionable insights.
But, what exactly are these tools? From tracking customer behavior to improving internal processes, their applications are diverse and wide-reaching. This overview aims to shine a light on fundamental principles, various types of analytics software, and the critical functionalities they offer. In doing so, we’ll also touch on the target audience for these technologies, ensuring a well-rounded comprehension.
In this guide, we will explore:
- The key features and functionalities of data analytics software.
- An analysis of pricing models and considerations for choosing the right tools.
As we dive deeper, the reader will uncover how these technologies can enhance operational efficiencies and inform strategic decision-making.
Key Features and Functionalities
Data analytics software is equipped with a range of features that cater to different business needs. Understanding these functionalities is crucial for decision-makers who want to harness the full potential of their data analytics investments.
Comprehensive Overview
At its core, data analytics software should provide a comprehensive suite of tools to facilitate various analytical tasks. Here are some key features typically found:
- Data Visualization: Graphs, charts, and dashboards that make data interpretation straightforward.
- Predictive Analytics: Tools that help forecast future trends based on historical data.
- Data Mining: The ability to discover patterns and relationships in large datasets.
- Reporting Tools: Automated reporting capabilities to generate insights without constant manual intervention.
- Integration Options: Compatibility with other software solutions, such as CRM systems or marketing platforms.
Each of these elements plays a vital role in ensuring that the data-driven decisions are not just based on gut feelings but rather informed by factual analysis.
Target Users
Data analytics software appeals to a diverse range of users. It is not just technology professionals but also business managers, marketers, and data scientists, among others. Understanding who benefits from the tools can help tailor its features:
- Decision-Makers: Executives need data that informs strategic business decisions.
- IT Professionals: They ensure the proper deployment and maintenance of the software.
- Marketers: They analyze consumer behavior to tailor campaigns effectively.
- Analysts: Individuals responsible for diving deep into datasets to derive meaningful insights.
For each target group, the needs and expectations from the software can differ significantly, underscoring the importance of selecting the right solution that aligns with business objectives.
Pricing Models and Cost Analysis
When it comes to adopting data analytics software, understanding pricing models is essential. The cost can vary significantly based on the features, user count, and services offered.
Breakdown of Pricing Tiers
Data analytics software often employs various pricing structures. Here are some common models:
- Subscription Model: This is often based on a monthly or yearly fee, giving users access to software updates and support.
- One-Time Purchase: Some solutions require a single upfront cost, but they might have limited updates or support.
- Tiered Pricing: Different levels of features available at different price points, allowing users to select a package that fits their needs.
Each model has its pros and cons, making it essential to evaluate them carefully based on the expected utilization and budget.
Additional Costs to Consider
Besides the basic pricing, there are several hidden costs that businesses should factor in:
- Training and Onboarding: New software often requires training sessions, which can add up.
- Maintenance and Support: Some plans may not cover extensive tech support.
- Customization: Tailoring the software to fit specific operational needs can incur extra expenses.
Evaluating these aspects will ensure that businesses make well-informed decisions when selecting data analytics solutions.
Defining Data Analytics Software
In an era where data plays a pivotal role in shaping business strategies, defining data analytics software becomes essential for decision-makers and IT professionals alike. Understanding what constitutes this software not only clarifies its components but also highlights its significance in today’s data-driven world.
This section aims to break down the fundamental elements of data analytics software, emphasizing its utility and impact on various business processes. The importance of this topic cannot be overstated; as organizations increasingly rely on data insights, comprehending analytics software helps in selecting the right tools that align with specific needs and objectives.
The Concept of Data Analytics
Data analytics is the systematic computational analysis of data. This process involves inspecting, cleansing, transforming, and modeling data to discover useful information, inform conclusions, and support decision-making. In a practical sense, data analytics allows businesses to harness vast amounts of data, converting it into actionable insights.
Understanding the concept of data analytics is akin to grasping the foundation of a building—without it, any efforts made on top can be precarious at best. Organizations depend on three primary forms of analytics: descriptive, predictive, and prescriptive analytics. Each serves a unique purpose:
- Descriptive Analytics: This type focuses on historical data to summarize what has happened in the past. It’s like looking in a rear-view mirror to understand your journey so far.
- Predictive Analytics: This form estimates future outcomes based on historical data patterns. It allows businesses to forecast trends, akin to checking the weather before heading out.
- Prescriptive Analytics: This advanced analytics technique suggests potential actions based on predictions. Imagine having a seasoned guide offering you the best routes based on traffic conditions.
By grasping these concepts, decision-makers can better navigate their approach to data and analytics, ensuring strategic choices foster growth and efficiency.
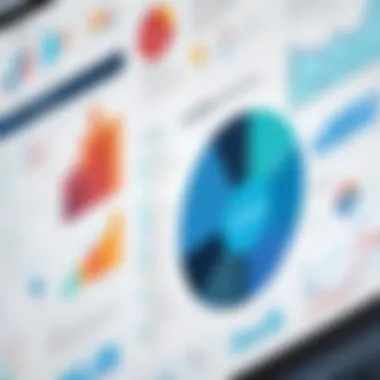
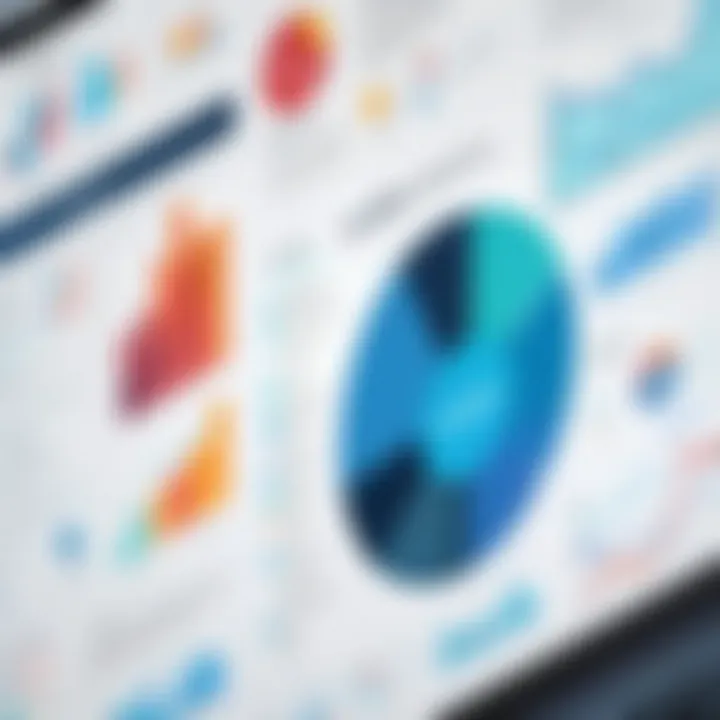
Characteristics of Data Analytics Software
When discussing data analytics software, certain characteristics distinguish the effective from the mediocre. Knowing these traits allows professionals to evaluate their options more judiciously. Here are key characteristics that one should consider:
- User-Friendly Interface: Software with an intuitive design ensures that users, regardless of their technical background, can interact with data seamlessly. A complicated interface can deter even the most seasoned analysts.
- Robust Data Integration: Excellent analytics software can bring together disparate data from various sources. Being able to merge cloud-based information with on-premises data is crucial for a holistic view.
- Customization Options: Every organization has unique needs; therefore, the ability to customize dashboards and reports can make software far more valuable. This flexibility allows for tailored insights exactly where they’re needed most.
- Advanced Data Visualization: The ability to transform complex data sets into visual formats—like charts, graphs, and dashboards—facilitates better understanding and communication of insights across the board.
"Organizations that prioritize effective data analytics software set themselves apart in their abilities to gain meaningful insights and react swiftly to market demands."
These characteristics collectively enhance the efficacy of data analytics software, driving better decision-making and ultimately contributing to operational excellence.
Types of Data Analytics Software
The realm of data analytics software encompasses several categories, each tailored to specific needs and objectives. Recognizing the types is the first step in understanding how to leverage data effectively. Each type of software offers distinct advantages and serves various purposes within business environments. By categorizing these tools, decision-makers can better assess what fits their requirements and goals.
Descriptive Analytics Tools
Descriptive analytics provides a foundational layer for all data analytics by telling you what has happened in your organization. These tools are like rearview mirrors for businesses, allowing them to look back on historical data and gauge performance trends. This particularly is useful for generating reports and dashboards that present data in an accessible format.
Key Points:
- Visualization: Often, descriptive analytics makes heavy use of visualization tools like Tableau or Google Data Studio. It turns complex data sets into straightforward graphs or charts that can be easily interpreted.
- Trend Analysis: By understanding past behaviors and outcomes, businesses can identify trends which can inform future strategies. For example, retailers can analyze sales data from previous years to optimize inventory.
- Data Aggregation: These tools consolidate data from multiple sources into a single view, enabling comprehensive insights from often siloed information.
Predictive Analytics Solutions
Predictive analytics moves beyond the historical scope of descriptive analytics to forecast future outcomes. It blends statistics, modeling, and machine learning to identify patterns that can drive actionable insights. Decision-makers can utilize these tools for a range of applications, from risk assessment to marketing campaigns.
Key Elements:
- Customer Insights: For instance, a marketing department can leverage predictive analytics to anticipate consumer behavior, determining what products to promote based on historical purchase data.
- Risk Management: Financial institutions often deploy predictive models to assess the risk of loan applicants, helping optimize approval processes with better accuracy.
- Scenario Analysis: Organizations can simulate different scenarios and their impacts, an essential aspect of strategic planning.
Prescriptive Analytics Platforms
Prescriptive analytics takes the insights gained from both descriptive and predictive analytics and offers recommendations for action. These tools act like navigational aids, guiding businesses towards optimal decisions based on data-driven forecasts. Understanding and implementing these systems can significantly enhance operational effectiveness.
Considerations:
- Recommendation Systems: An example would be logistics companies using prescriptive analytics for supply chain management, where it can suggest adjustments to routing and inventory levels in real-time.
- Resource Allocation: Businesses can optimize resource distribution based on prescriptive insights, ensuring maximum efficiency and reduced waste.
- Automated Decision-Making: With advances in AI, some prescriptive platforms can automatically adjust processes without human intervention, streamlining operations further.
Real-time Analytics Applications
In today's fast-paced business environment, real-time analytics has become indispensable. Rather than relying on historical data, these applications continually analyze incoming information and deliver insights almost instantaneously. This capability allows organizations to respond quickly to changes and capitalize on emerging opportunities.
Key Advantages:
- Immediate Insights: Think about a stock trader who uses real-time analytics to react to market fluctuations. The difference between a good and great trade often comes down to timing.
- Operational Efficiency: Manufacturing industries, for instance, can leverage real-time analytics to monitor equipment health, predicting failures before they occur—hence minimizing downtime.
- Customer Engagement: Real-time customer analytics allows businesses to tailor interactions in the moment, giving a personalized touch that enhances customer satisfaction.
In summation, understanding the various types of data analytics software is crucial for businesses aiming to make informed decisions and improve operational efficiency. With the right tools in hand, organizations can transform the way they utilize data, fostering an environment that thrives on insight-driven strategies.
Key Features to Consider
When selecting data analytics software, it’s not just about picking any tool off the shelf. Organizations need to scrutinize key features that can make or break their analytical endeavors. These features play a pivotal role in determining how effectively the software can address specific business needs and enhance overall operational efficiency. Understanding these elements empowers decision-makers, IT professionals, and entrepreneurs to make informed choices that align with their strategic goals.
Data Visualization Capabilities
Having a powerful data analytics tool means little if the information it processes is hard to grasp. Data visualization is the lens through which data becomes understandable and actionable. The best analytical software provides intuitive visual representations like charts, graphs, and dashboards. These capabilities can turn mountains of complex data into user-friendly visuals that reveal trends, patterns, and anomalies in a blink.
- Interactive Dashboards: Users appreciate dashboards that refresh data in real-time. This interactivity allows them to dig deeper into datasets by filtering and drilling down, uncovering insights that might otherwise remain hidden.
- Customization Options: Not all users need the same visuals. Ability to customize visual formats to suit specific reporting requirements makes a significant difference.
- Collaboration Tools: Sharing insights with team members should be seamless. Robust software includes features that allow multiple users to annotate and discuss visuals together—ideal for fostering group discussions and strategic brainstorming.
Integration with Other Systems
In today’s interconnected business world, a data analytics solution often needs to play nice with existing systems. Integration capabilities are paramount, facilitating smooth data flows between various platforms—think CRMs, ERPs, and other databases.
- APIs and Data Connectors: An effective data analytics software should support various Application Programming Interfaces (APIs) and connectors to pull in data from diverse sources. This reduces the chances of data silos, ensuring that decision-makers have a holistic view of their operations.
- Automation Capabilities: An ideal system should enable automated data uploads from external software. This kind of automation not only saves time but also reduces human error in data handling.
Scalability and Flexibility
As businesses grow, their analytical demands evolve. Scalable solutions can adapt to changing data loads without compromising performance. This adaptability ensures that organizations don’t have to abandon their existing software as they expand.
- Subscription Models: Some platforms offer tiered subscription models allowing users to adjust their usage as needs change. This ensures that businesses only pay for what they use, which is often a more cost-effective approach.
- Support for Big Data: As businesses accumulate larger datasets, it’s critical to select software that effectively manages big data. Check if the system can handle high volumes of information without lagging.
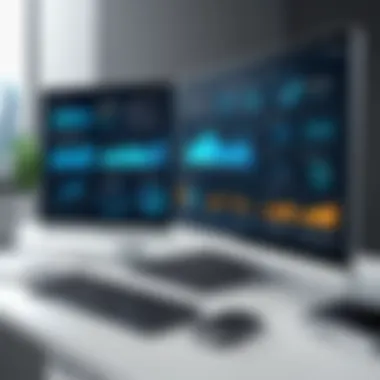
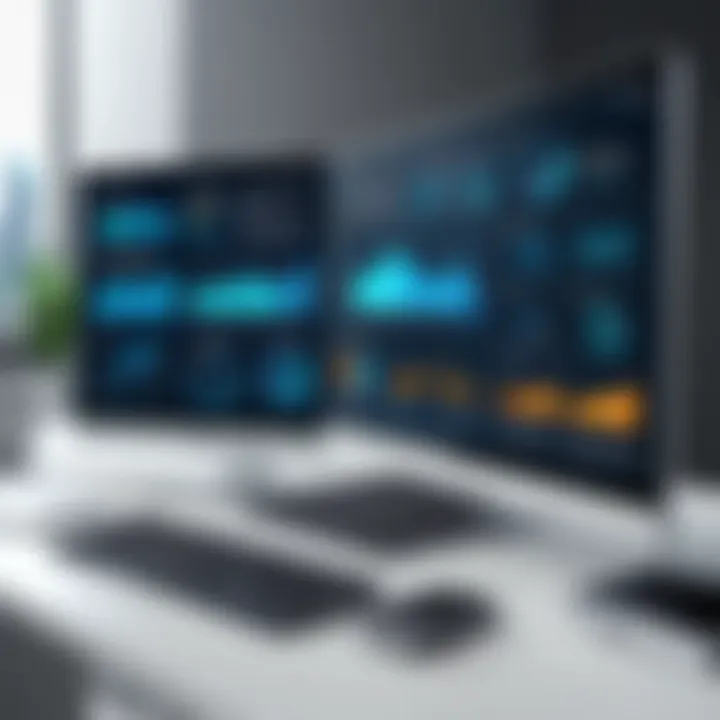
User-Friendly Interface
No matter how advanced the software, if users find it complex and challenging, it will likely lead to frustration and errors. A user-friendly interface is key to ensuring that teams can effectively navigate the software with minimal training.
- Intuitive Design: Look for software that follows familiar design conventions. This lowers the learning curve, allowing team members to start leveraging insights almost immediately.
- Help Resources: It’s also essential to have access to help documents, tutorials, or customer support in case users hit a snag.
Achieving data-driven success hinges on finding software that not only meets technical requirements but also caters effectively to the end-user’s experience.
In summary, evaluating these key features can significantly tilt the scales in favor of a data analytics software that is not only powerful but also perfectly aligned with an organization’s needs. Each feature has its own value, and collectively, they ensure that the analytical tools function optimally in extracting actionable insights from raw data.
The Role of Data Analytics Software in Business
Data analytics software plays a pivotal role in today’s business landscape. It serves not just as a tool but as a fundamental driver of strategic thinking and operational practices. Understanding the role of data analytics software is crucial for decision-makers keen on leveraging insights derived from data. It aids in identifying patterns, understanding customer behaviors, and ultimately contributes to crafting better business strategies.
Enhancing Decision-Making Processes
In a world overflowing with data, the challenge lies not in gathering information but in deriving actionable insights from it. Data analytics software equips businesses with the information they need to make informed decisions, propelling them forward rather than leaving them floundering in a sea of numbers.
To illustrate, consider how a retail chain uses analytics tools to sift through sales data. By pinpointing the preferences of consumers, the store’s managers can make tactile decisions around stock levels and promotional strategies. For instance, if the data reveals a sudden spike in the sales of summer apparel, decision-makers can quickly ramp up inventory or tweak marketing messages to match consumer demand.
"Data is the new oil. It’s valuable, but if unrefined it cannot really be used."
— Clive Humby
Driving Operational Efficiency
Improving efficiency is not just about trimming unnecessary costs; it’s about making processes work smarter. By integrating data analytics software into day-to-day operations, companies can identify bottlenecks, reduce waste, and enhance productivity.
For example, a manufacturing firm might use analytics tools to monitor production lines in real time. If the software highlights a slowdown on a specific machine, immediate action can be taken to resolve the issue, preventing a potential backlog that could be costly. This not only saves time but also ensures that resources are allocated where they are needed most.
Here are some operational efficiencies gained through analytics:
- Streamlined workflows
- Improved resource allocation
- Reduction in redundancies
- Enhanced quality control processes
Predicting Market Trends
Anticipating market shifts is a game-changer for businesses aiming to stay ahead of the curve. Data analytics software can analyze vast datasets to forecast trends, unraveling patterns that might not be apparent at first glance. Companies that invest in predictive analytics are more likely to spot opportunities and mitigate risks effectively.
For example, a financial services firm might analyze transaction data alongside external economic indicators to spot emerging market trends. On recognizing a trend towards sustainable investment, the firm can adjust its strategies accordingly, making it a frontrunner in a niche market before competitors even realize what’s happening.
Personalizing Customer Experiences
Customer experience has become a battlefield, with brands competing to offer the most tailored interactions. Data analytics allows businesses to delve deeply into the preferences and behaviors of their customers. With data-driven insights, companies can craft personalized marketing messages, improving engagement and loyalty.
Take, for instance, an online streaming service. By analyzing viewing habits, the platform can create tailored recommendations that resonate with user interests. When a viewer sees personalized suggestions, they’re not just more likely to engage – they feel seen and valued as a customer.
Evaluating Data Analytics Solutions
Evaluating data analytics solutions is a pivotal process in maximizing the potential of data-driven insights within an organization. The right software can transform raw data into actionable intelligence, driving business strategies and operational improvements. As businesses navigate the complexities of data and analytics, understanding how to assess and select the right tools becomes essential. In this section, we’ll probe the key elements involved in the evaluation process, encompassing the significance of identifying business needs, engaging in comparative analysis of software options, and considering budget implications.
Identifying Business Needs
When diving into the evaluation of data analytics solutions, the first step is to pinpoint the specific needs of your business. This clarity allows organizations to align analytics capabilities with their objectives. Factors to consider include:
- Goals and Objectives: Determine what you hope to achieve with data analytics. Are you looking to enhance operational efficiency, drive customer insights, or predict market trends?
- Data Sources: Understand where your data is coming from—internal databases, third-party data, or real-time data feeds. Each source might require specific tools or integration capabilities.
- User Demographics: Identify who will be using the software. Certain teams might need different functionalities; for instance, marketing may prioritize user-friendly dashboards while data analysts may require advanced modeling tools.
A solid grasp of these elements aids in narrowing down software options that meet practical requirements, ensuring the end solution is not only fitting but also scalable for future needs.
Comparative Analysis of Software Options
Once business needs have been established, the next logical step is to conduct a comparative analysis of potential software options. This exploration provides clarity on feature sets, ease of use, and integration capabilities. Here’s how to approach it:
- Feature Comparison: List down all the critical features that each software provides. Consider components like data visualization, predictive modeling, and reporting tools.
- Usability Assessment: User experience is paramount. Engaging with trial versions or demos can give a better sense of the software’s intuitiveness and practicality.
- Integration Capabilities: Assess how well the software integrates with existing systems. The more seamless the integration, the less time will be wasted on adjustments and troubleshooting.
- Vendor Reputation: Research vendors thoroughly. Look for reviews, case studies, and user testimonials to gauge reliability and customer satisfaction.
Undertaking a meticulous comparative analysis enables informed decision-making and reduces the risk of poor investment choices down the line.
Cost Considerations
The financial aspect cannot be overlooked when evaluating data analytics solutions. While investing in analytics software can drive significant returns, it’s essential to weigh costs against potential benefits. Considerations here include:
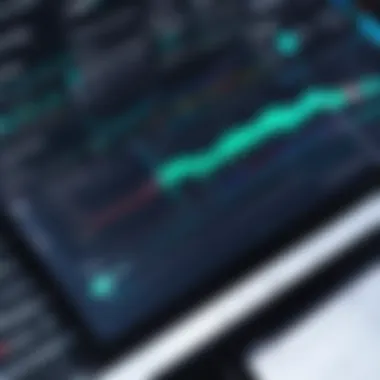
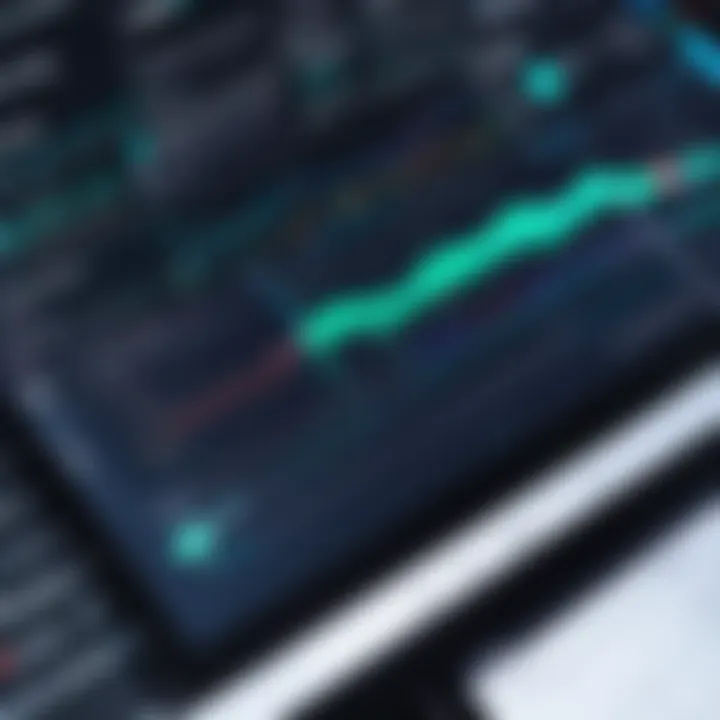
- Upfront Costs vs. Ongoing Fees: Analyze the initial costs for purchasing software and any recurring charges for licenses, support, or upgrades.
- Return on Investment: Think about how the software can lead to efficiencies that translate to financial gains. Will it save time, reduce labor costs, or lead to increased revenues?
- Hidden Costs: Be on the lookout for any hidden costs associated with training, integration, or maintenance that may arise later.
A detailed understanding of costs helps shape the decision, ensuring it aligns with budgetary constraints while maximizing value.
"Evaluating a software solution is not just about what it can do, but how well it fits within the puzzle of the business's unique needs."
In summation, evaluating data analytics solutions is critical for organizations seeking to capitalize on their data assets. By carefully identifying business needs, comparing options diligently, and considering costs thoughtfully, decision-makers can position their businesses to leverage data analytics effectively. This approach leads to informed choices that can enhance operational efficiency and support strategic objectives.
Challenges in Data Analytics
In the realm of data analytics, challenges are as prevalent as the data itself. Acknowledging these hurdles is crucial for decision-makers, IT professionals, and entrepreneurs. Understanding them allows for more effective planning and smoother implementation of data analytics software. The challenges can significantly impact overall efficiency and outcomes, making it essential to address them up front.
Data Quality Issues
Data quality plays a central role in the analytics process. If the underlying data is flawed—missing values, inaccurate entries, or outdated information—then the results derived from this data will be equally unreliable. Poor data quality can lead to misguided decisions, which might steer a company off course. To maintain high data integrity, organizations should adopt strict data governance policies. This may involve setting clear standards for data entry and regularly auditing the data warehouse to identify any inconsistencies. Adopting automated data cleaning tools can also help reduce the burden on staff and improve data accuracy.
Skill Gaps in Workforce
Even the finest software can't work miracles without the right talent behind it. The skill gap in the workforce is a pressing issue in the data analytics field. Many organizations struggle to find personnel proficient in data science, statistics, or advanced analytics tools. Training current employees can be a worthwhile investment. Online courses and workshops enable workers to upskill and stay up to date with industry trends. Additionally, fostering a culture of continuous learning can help to empower teams. In some cases, it may make sense to collaborate with educational institutions to bridge this gap directly, ensuring that the skills taught align closely with real-world applications.
Privacy and Security Concerns
With the explosive growth of data analytics comes an equally significant responsibility: safeguarding sensitive information. Privacy and security concerns can't be overlooked, especially when handling customer data. Organizations must comply with regulations like GDPR or HIPAA, safeguarding personal information and ensuring ethical use of data. Implementing robust security protocols is essential to protect against data breaches. Techniques such as data anonymization, encryption, and regular security audits can help mitigate these risks. Furthermore, having a well-established incident response plan will prepare organizations should a breach occur, minimizing damage and ensuring regulatory compliance.
"In today's data-driven age, overlooking challenges in data analytics is like sailing without a compass—aimless and fraught with risk."
By addressing these challenges directly, organizations can significantly enhance their analytics capabilities, leading to more informed decision-making and ultimately, a competitive edge in today's fast-paced business environment.
Future Trends in Data Analytics Software
The landscape of data analytics is always shifting, much like sand in an hourglass. As technology evolves, so do the methods and tools that businesses rely on for extracting insights from their data. Understanding these trends is not just about keeping up but strategically positioning oneself to harness potential opportunities. The implications of future trends in data analytics software encompass efficiencies, competitive advantages, and fundamentally changing the framework of decision-making processes in organizations.
Artificial Intelligence Integration
Artificial intelligence is becoming a game changer in data analytics. With the exponential growth of data, conventional analytic methods struggle to keep the pace. By integrating artificial intelligence into analytics tools, businesses can automate data processing and analysis, making insights not only quicker to obtain but more accurate.
Consider how AI algorithms can continuously learn from new data input to refine their models, enhancing predictive accuracy over time. This means less manual oversight is needed, freeing up human resources for tasks that demand creativity and strategic thought. Furthermore, AI can discover patterns that may go unnoticed by human analysts, allowing organizations to uncover hidden opportunities or risks.
Implementing AI does come with challenges. There’s the need for skilled personnel who understand not just the technology, but also the ethical implications of AI use in data analysis. Organizations must invest in both technology and talent to navigate this landscape effectively.
Increased Focus on Data Governance
As analytics tools proliferate, so too do concerns about data governance. This isn't just bureaucratic red tape; it's about ensuring that data is accurate, accessible, and used responsibly. With regulations like GDPR in Europe and CCPA in California making headlines, businesses must prioritize robust data governance strategies to mitigate risks associated with non-compliance.
Data governance also paves the way for better data quality. Clear policies and frameworks help prevent data silos, promoting a culture of shared insights across teams. Proper governance leads to accountability, ensuring that teams understand their roles in data management and analytics. Thus, organizations looking to future-proof their analytics capability must weave data governance into their fabric.
“Data governance is not an afterthought; it’s a necessity in the age of digital transformation.”
Growth of No-code Analytics Platforms
One of the more remarkable trends is the rise of no-code analytics platforms. These tools allow users without extensive technical expertise to engage with data analysis, democratizing access and promoting a culture of data-driven decision-making. Imagine a marketing manager who can create reports and dashboards without relying on IT—this is the reality that no-code platforms deliver.
No-code analytics platforms empower business units to get insights quickly, without the traditional bottleneck that comes from relying on dedicated data teams. However, it’s crucial to be aware of the limitations of these platforms. While they reduce the barrier to entry, there are still complexities in data interpretation that necessitate some foundational knowledge. Therefore, organizations should consider training and education around data literacy to maximize the benefits of these tools.
End
In reviewing the complexities and functionalities of data analytics software, it becomes clear that this area of technology is not just a trend but an essential component of effective business strategy. As organizations increasingly rely on data for decision-making, the importance of understanding how different analytics tools work cannot be overstated. A well-informed approach to choosing the right software can significantly enhance an organization’s operational capabilities and competitive standing.
Recap of Key Points
To summarize, several critical aspects of data analytics software have been discussed:
- Definition and Purpose: Data analytics software is designed to collect, process, and analyze data to derive meaningful insights. Its ultimate goal is to transform raw data into actionable information.
- Varieties of Analytics Tools: Key types include descriptive, predictive, prescriptive, and real-time analytics, each serving different purposes from summarizing historical data to forecasting future trends.
- Features to Evaluate: Essential features to consider include data visualization capabilities, user-friendliness, integration with existing systems, and scalability factors. These elements can significantly affect the efficacy of the software in real-world applications.
- Business Impacts: The role of data analytics in enhancing decision-making, improving operational efficiency, predicting market changes, and personalizing customer experiences was highlighted, showcasing its strategic importance to modern businesses.
- Evaluation and Selection: The process of assessing software options typically involves aligning business needs with available features, analyzing costs, and considering potential challenges that might arise during deployment.
- Emerging Trends: The landscape of data analytics is evolving with proven concepts like AI integration, a focus on data governance, and the rise of no-code platforms designed to make analytics more accessible.
Final Thoughts and Recommendations
As we draw conclusions from our exploration, it is imperative for decision-makers and IT professionals to remain vigilant regarding the fast-paced developments within data analytics. The future promises substantial innovations that can further empower business insights.
Nonetheless, selecting the right data analytics software should not be rushed. Organizations should conduct thorough research and trial different solutions to discern what best suits their unique operational needs. It’s wise to pay attention to ongoing developments in the field, continuously adapt, and invest in training for staff to bridge skill gaps.
Lastly, while data analytics can yield substantial benefits, businesses must be aware of the underlying ethical and security challenges that accompany data collection and usage. Addressing these aspects responsibly will not just build trust with customers but also lay the groundwork for sustainable growth.
"The application of data analytics is not merely about having access to information; it is about knowing how to use it effectively. The insights derived from data are only as valuable as the actions taken based on them."
In closing, the role of data analytics software is crucial in enriching business strategies, and with the right approach, companies can leverage data to outperform competitors in today’s data-driven market.