Fraud Detection Strategies in Financial Services
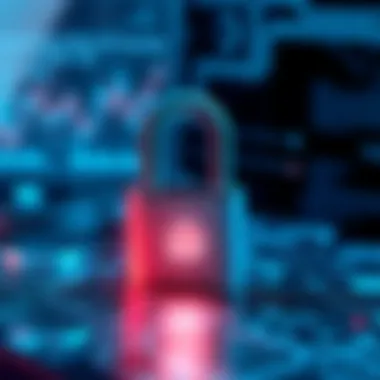
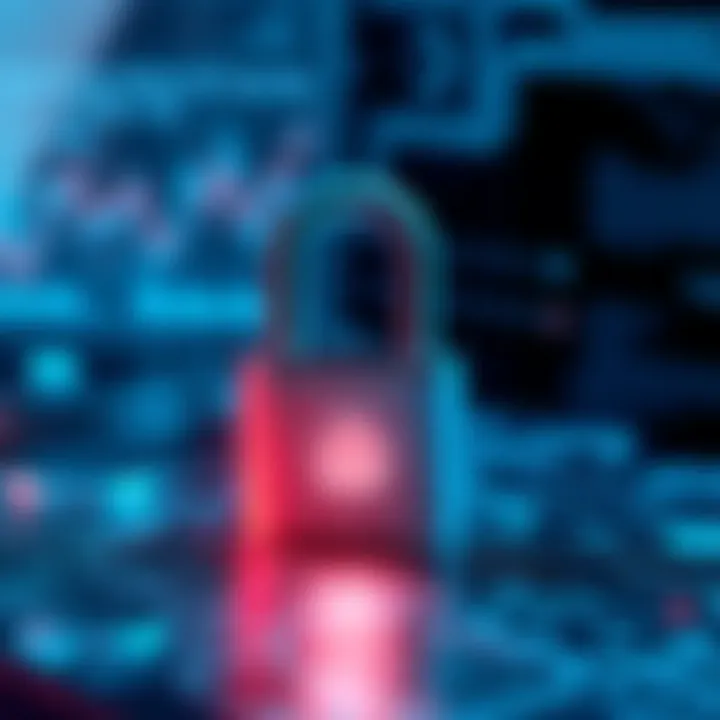
Intro
Fraud detection within the realm of financial services is a multi-faceted endeavor that continues to gain prominence in today’s economy. As more individuals rely on digital platforms for banking, investing, and transactions, the potential for fraudulent activities grows exponentially. In an environment where every click and swipe can lead to significant monetary losses, financial institutions are left grappling with the necessity to protect customer assets and maintain trust.
The stakes are exceptionally high, and the challenges multifarious. Fraudsters are becoming increasingly sophisticated, clever in their manipulation of technology and human behavior. This necessitates not just robust detection strategies but also a comprehensive understanding of the underlying mechanisms of fraud itself.
This article delves into various dimensions of fraud detection, from the nitty-gritty of regulatory frameworks to the cutting-edge technologies harnessed for prevention. Financial institutions must stay ahead of the curve, using data-driven approaches to enhance their defensive measures.
Understanding the intricacies involved not only arm decision-makers with insights but also equips IT professionals with the tools needed for implementation. This exploration fosters a clearer picture of how various elements come together in the fight against financial fraud, ultimately presenting both challenges and solutions.
Key Features and Functionalities
In any conversation about fraud detection, it’s crucial to pinpoint the key features that constitute an effective system.
- Comprehensive Overview: A thorough understanding of fraud detection requires awareness of various types of fraud, such as identity theft, online fraud, and insider threats. By mapping out these categories, institutions can better tailor their strategies to counter specific risks.
- Real-time Monitoring: Systems that provide real-time tracking capabilities are essential. Voyaging into historical data is useful, but being able to react immediately to suspicious activities can be the difference between prevention and loss.
- Machine Learning Algorithms: The integration of machine learning helps institutions analyze vast amounts of data to create predictive models. These models can identify patterns that humans might overlook, enabling early detection of fraudulent activities.
- Risk Assessment: Implementing tiers of risk assessment allows for prioritizing potential threats. Not all transactions carry the same level of risk, so an effective system should distinguish between high-risk and low-risk activities.
- User-Friendly Interface: Even the most sophisticated systems are ineffective if not user-friendly. A well-designed dashboard can help fraud analysts quickly identify anomalies and streamline their workflows, enhancing operational efficiency.
"In the world of finance, knowledge is power. The more we understand fraud, the better equipped we are to detect and prevent it."
Target Users
The target users of fraud detection systems span various roles within financial institutions. Decision-makers, including executives and managers, require insights for strategic planning. They must understand the implications of fraud detection within the broader context of organizational safety and customer service.
IT professionals are equally important; they need the technical know-how to implement and manage sophisticated fraud detection systems. With their expertise, they ensure that the technology deployed is effective and up to date with the latest developments in cybersecurity and analytics.
Finally, the front-line staff who interact with clients directly should be versed in recognizing potential fraudulent behavior. Empowering them with knowledge is key, as they often serve as the first line of defense against fraud.
With advancements in technology continually changing the landscape of fraud detection, an informed approach that combines the right tools and strategies is paramount in achieving secure financial services.
Understanding Financial Fraud
Understanding financial fraud is important because it lays the groundwork for effective fraud detection techniques and strategies in the financial services sector. Fraud affects not just individual consumers but also entire institutions, which can suffer substantial economic and reputational damage. By grasping the makeup and nuances of financial fraud, stakeholders can implement more adapted measures that prioritize prevention and mitigation.
Fraud isn't a blanket term; a spectrum exists, each type carrying its own implications and detectable signs. Recognizing the unique characteristics of different types of fraud can significantly enhance detection capabilities. Moreover, a comprehensive understanding provides the insight into patterns that can serve as a pivotal marker for predictive modeling.
Types of Financial Fraud
Credit Card Fraud
Credit card fraud remains among the most prevalent types of financial fraud, impacting consumers and banks alike. With its rising occurrence, understanding it becomes essential in recognizing threat patterns. Credit card fraud involves unauthorized usage of someone else's card to make purchases or withdraw cash. The key characteristic here is the ease with which fraudsters can utilize stolen card data, particularly online. Factors like its widespread acceptance and the rise of online shopping make this type of fraud a pressing issue.
One unique feature of credit card fraud is its rapid execution; transactions can occur in an instant, making it challenging to catch in real-time. However, this very speed can sometimes work against the perpetrator if effective monitoring systems are in place. For financial institutions, reducing credit card fraud can lead to significant savings, but it often requires a hefty investment in technology and staff training.
Identity Theft
Identity theft is a cunning crime where someone’s personal information is stolen to assume another's identity for fraudulent purposes. Its contribution to financial fraud is notable, as it often serves as a gateway to other crimes, such as credit card fraud or loan fraud. The primary characteristic that makes identity theft particularly dangerous is its hidden nature; victims are often unaware until significant damage has been done.
The unique feature here is the long-term impact; identity theft can take months or even years to resolve completely, with victims often facing difficulties in restoring their credit ratings. Financial institutions need to adopt robust verification processes to combat identity theft effectively, which can sometimes interrupt service delivery and frustrate legitimate customers.
Investment Scams
Investment scams appear particularly sophisticated, often targeting individuals who are eager to grow their wealth. These scams promise substantial returns on unrealistic opportunities, often operating under a veneer of legitimacy. They contribute to the overall landscape of financial fraud by leveraging trust—scammers often cultivate relationships before launching into their schemes.
A key characteristic of investment scams is the appeal to emotions, like fear of missing out or the urge to secure a better future financially. They often present a unique danger due to their mimicking of credible investment vehicles, making it challenging for the average investor to identify red flags. On the flip side, financial institutions need to educate their clients on how to discern genuine opportunities from scams, which requires ongoing resources and awareness programs.
Insurance Fraud
Insurance fraud is a well-versed area of financial fraud, where individuals or entities deceive insurance providers for unwarranted benefits. The impact of such fraud can ripple through the entire industry, leading to increased premiums and strained relationships with honest policyholders. Its characteristic lies in the various forms it can take, from faking accidents to inflating claims.
The unique feature of insurance fraud is that it sometimes involves collusion between multiple parties, such as corrupt agents and policyholders. This kind of fraud is particularly challenging to mitigate due to the layers of deception involved. However, financial institutions implementing stringent verification checks can minimize the risks associated with this type of fraud, often at the expense of flexibility in processing claims.
Impact on Financial Institutions
Financial Losses
Financial losses due to fraud are staggering and often escalate quickly. Institutions may face direct monetary losses resulting from theft or indirect losses due to increased operational costs as they heighten security measures. In this article, understanding financial losses is crucial because they highlight the severe economic implications that can threaten the health of an organization.
A key characteristic of financial losses is that they not only impact profit margins but can also lead to layoffs and additional financial strain. The unique aspect of these losses is their compound effect; repeated incidents can snowball into a crisis, diminishing consumer trust and affecting market share. Hence, financial institutions must grasp the full spectrum of possible losses and not merely view fraud as isolated events.
Reputational Damage
The reputational damage caused by financial fraud can be insidious and long-lasting. Clients and the general public may lose faith in an institution if it becomes embroiled in a fraud scandal, leading to waves of defections to competitors. This is one of the reasons understanding reputational damage is a crucial piece of the puzzle presented in this article.
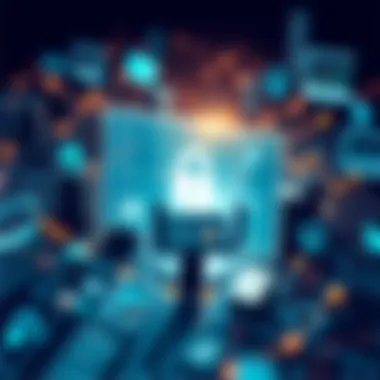
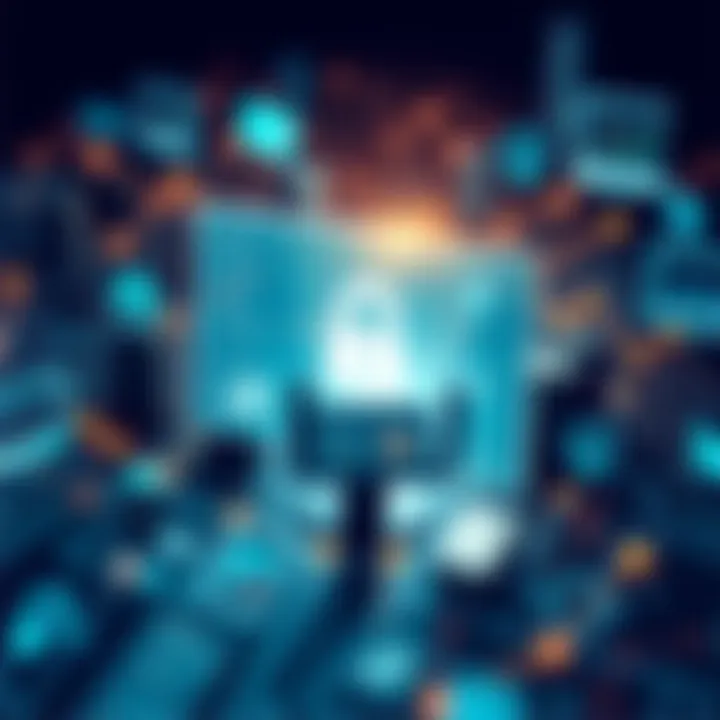
Reputational damage can be characterized by public sentiment and media portrayal. Once a negative perception takes root, it can be resilient. A unique feature of reputational damage is that it might not always correlate with actual losses; a bank could have an ironclad security system but may still appear vulnerable due to bad press. Addressing reputational issues often requires a comprehensive public relations strategy, which adds another layer of complexity.
Regulatory Penalties
Regulatory penalties can serve as a double-edged sword for financial institutions. While they are intended to safeguard consumers and maintain market integrity, these penalties can result in crippling fines. Understanding regulatory penalties is critical because they compel financial services to adhere to laws that govern fraud prevention.
A hallmark of regulatory penalties is that they can arise unexpectedly, plunging institutions into a state of crisis management. The unique aspect is that these penalties can also tarnish credibility with regulators, leading to heightened scrutiny in the future. Mitigating the risk of regulatory penalties necessitates continual updates to compliance measures, often leading institutions to invest significantly in legal advice and technology.
The Importance of Fraud Detection
Fraud detection is a cornerstone of stability and trust within financial services. Its importance is multifaceted, greatly affecting not just financial institutions but also customers and regulatory frameworks. Without effective fraud detection mechanisms in place, institutions would face a multitude of risks that could spiral out of control, impacting their operational integrity and customer relationships.
Protecting Customer Assets
In a world where financial transactions are relentless and digital channels abound, safeguarding customer assets takes on a central role. Customers expect their sensitive information and funds to be protected at all costs. When fraud occurs, the repercussions can be devastating not just financially but emotionally. Consumer trust can erode faster than a house of cards, leading to disengagement from services. Institutions actively investing in robust fraud detection programs reinforce their commitment to customer safety.
Moreover, effective fraud detection systems provide a safety net, catching suspicious activities before they escalate into full-blown crises. For example, a credit card issuer utilizing advanced monitoring tools can quickly identify unusual spending patterns that might signal fraud. In the event of a customer’s account being compromised, prompt alerts can mitigate loss, ensuring that customer assets remain secure.
Ensuring Regulatory Compliance
Financial institutions are not only responsible for their customers but also for adhering to a myriad of regulatory requirements. Compliance isn’t just a box to tick off; it carries heavy implications. Regulation such as the Bank Secrecy Act and Anti-Money Laundering laws mandates that financial entities have effective measures in place to detect and report fraudulent activities. Failing to meet these requirements can lead to hefty fines or even the revocation of licenses.
Securing compliance through fraud detection not only shields institutions from penalties but enhances their reputation in the competitive landscape. When an institution demonstrates its commitment to preventing fraud, it reels in customers who prioritize security.
“Effective fraud detection mechanisms are not merely tools but vital partners in securing the financial ecosystem.”
Additionally, compliance extends beyond legalities; it fosters a culture of accountability and ethical practices. Employees become well-versed in identifying and reporting suspicious behavior, building a resilient institution that stays ahead of potential threats. Moreover, regulators favor institutions that exhibit transparency and a proactive stance against fraud.
The integration of fraud detection systems serves as both a protective measure for customer assets and a robust framework for ensuring regulatory compliance. In the fast-paced world of finance, the stakes are high, and institutions that prioritize these facets will not only weather the storm but thrive.
Traditional Fraud Detection Methods
Traditional fraud detection methods play a crucial role in the broader context of fraud prevention. These methods lay down the groundwork for identifying suspicious activities before they escalate into significant financial losses. In a sector where every penny counts, especially for banking systems, effective fraud detection ensures not only profitability but also customer trust. This section explores some of the most established techniques, such as rule-based systems and manual investigations, and elucidates their functionalities, advantages, and drawbacks.
Rule-Based Systems
Rule-based systems have stood the test of time as a principal method in fraud detection. They operate on established rules that trigger alerts upon detecting specific conditions or patterns that indicate potential fraud.
Thresholds and Alerts
Thresholds and alerts are a foundational element of rule-based systems. By defining specific parameters, such as transaction amounts or frequency, organizations can flag activities that deviate from expected norms. This automated response mechanism is a key characteristic of such systems, making them a reliable choice for initial fraud detection.
Unique to thresholds and alerts is their ability to deliver quick insights; an unusually high transaction or a sudden increase in account access might send alarms at the click of a button. However, while they are beneficial, they come with limitations. For instance, these systems can easily overwhelm investigators with false alarms, particularly if the thresholds are poorly set.
Human Oversight
Human oversight complements rule-based systems by adding a layer of judgment to the automated processes. This characteristic is especially significant in refining detections, as humans can offer insights and context that machines simply do not possess.
What sets human oversight apart is the potential to assess situations holistically. Trained analysts can investigate alerts and differentiate between actual fraud attempts and legitimate customer behavior. However, this approach isn't without its drawbacks. Dependence on human availability can lead to inconsistencies and variances in outcomes, which can stretch resources thin.
Manual Investigations
Manual investigations, while labor-intensive, serve as another vital tool in the anti-fraud arsenal. These methods involve thorough examination by investigating teams who sift through data, looking for signs of duplicity.
Limitations of Human Analysis
Despite its benefits, there are notable limitations associated with manual analysis. The primary issue is the expertise required to spot red flags efficiently. This intensive process can often miss subtler fraud patterns, owing to the sheer volume of data analysts must grapple with. Moreover, the variability in analysts’ experience can lead to inconsistent conclusions.
Another downside is the potential for human bias. Preconceived notions about customer behaviors can skew an analyst's judgment, leading to distorted findings.
Time Constraints
Time constraints are arguably one of the most pressing challenges in manual investigations. In an era where fraudsters constantly innovate, investigators must act quickly. The need for timely analysis weighs heavily on teams, ensuring that any lags can give fraudsters a head start.
These constraints highlight the need for swift decision-making within the financial industry. A delayed response can mean a larger financial catastrophe. Thus, while manual investigations have their merits, their effectiveness can often be hampered by the relentless pace at which fraudulent activities evolve.
"In the world of fraud detection, speed is as important as accuracy; one can't afford to weigh every option for too long."
Modern Techniques in Fraud Detection
The financial world is continuously evolving, and with it, the methods employed by those aiming to commit fraud. Traditional detection techniques often struggle to keep up with rapid technological advancements. Consequently, modern techniques have become indispensable in the quest to combat fraud effectively. Leveraging innovations like machine learning and advanced data analytics tools, this contemporary approach enhances the ability of financial institutions to identify and mitigate fraudulent activities. Through these methods, businesses can not only protect their assets and reputations but also instill greater confidence among their customer base.
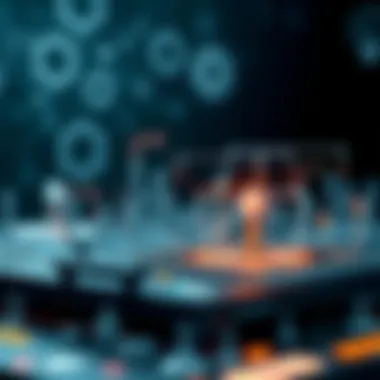
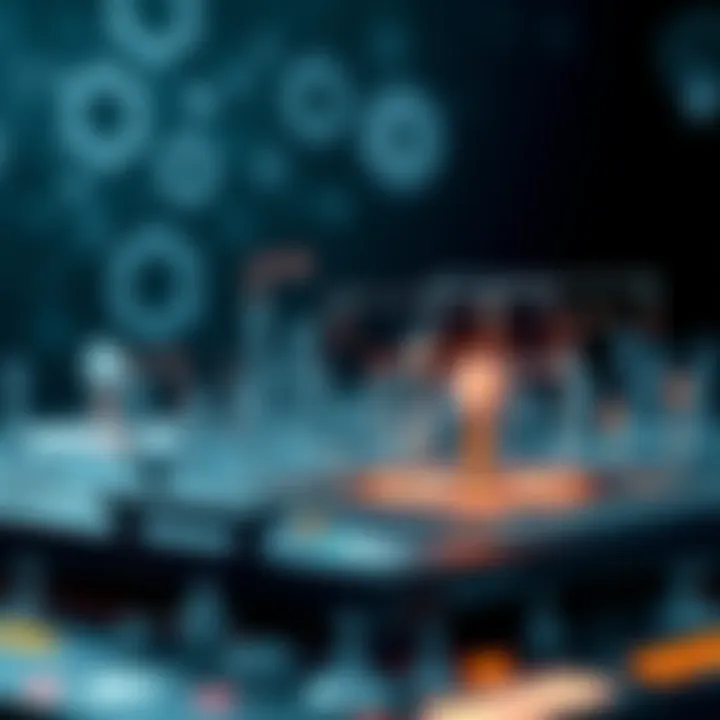
Machine Learning Applications
Predictive Modeling
Predictive modeling harnesses historical data to forecast future events, making it a cornerstone of modern fraud detection. By analyzing past behaviors and transactions, predictive models can identify patterns and trends that signal potential fraudulent activity. One key characteristic of this method is its ability to continuously learn and adapt. This machine learning feature makes it popular, as it provides financial institutions with a proactive means to outpace fraudsters.
A unique aspect of predictive modeling lies in its scalability. As more data flows into the system, its accuracy and efficiency can improve, enhancing its predictive capabilities. However, there can be downsides; if the data fed into the model is biased or flawed, it can lead to misleading conclusions. Ultimately, predictive modeling offers significant advantages to the financial sector, including improved accuracy in identifying high-risk transactions and a more robust defense against evolving fraud tactics.
Anomaly Detection
Anomaly detection zeroes in on unusual patterns or behaviors that deviate from the norm. This approach is particularly effective in spotting emerging fraud tactics, as it doesn’t rely on pre-existing fraud indicators. The key characteristic of anomaly detection is its focus on individual transactions or behaviors, which allows it to catch suspicious activity that might otherwise slip through the cracks.
The unique feature here is its ability to work in real-time, flagging activities as they occur. This immediacy is a massive advantage, allowing institutions to take swift action. However, it does come with challenges; false positives can be a concern, leading to unnecessary investigations and frustrations for genuine customers. The interplay between detection accuracy and operational efficiency becomes crucial. Through effective use of anomaly detection, banks and financial entities can enhance their vigilance against fraud while balancing customer experience.
Data Analytics Tools
Real-Time Monitoring
Real-time monitoring establishes a crucial mechanism for spotting fraudulent activities as soon as they occur. This method enables financial institutions to respond promptly, thus minimizing potential losses. The hallmark of real-time monitoring is its immediacy; it provides a live view into transactions, allowing suspicious activities to be flagged almost instantaneously. For today's fast-paced financial environment, this real-time capability is immensely beneficial, as fraud can escalate quickly without prompt intervention.
However, implementing effective real-time monitoring systems demands significant resources and investment. Institutions must maintain underlying infrastructure and fine-tune algorithms to ensure they are both robust and efficient. Balancing the cost of continuous monitoring with the benefits it brings is critical for financial entities, providing a two-sided dynamic that needs careful consideration.
Data Visualization
Data visualization plays a pivotal role in translating complex datasets into understandable formats. By presenting data graphically, organizations can highlight patterns, trends, and anomalies that might not be evident from raw data alone. An important aspect of data visualization is its ability to facilitate quick interpretations, enabling professionals to spot potential fraudulent activities swiftly.
The unique aspect of data visualization tools is their interactive nature—users can manipulate data views to explore various facets. While this offers immense benefits in gleaning insights, it can also lead to oversimplifications. Over-relying on visual tools without deep analysis might overlook nuanced data that tells a more comprehensive fraud story. to balance the benefits— like clarity and understanding—with potential oversights is vital in the evolution of fraud detection strategies.
"In the age of data, it's not just about having information; it's about deriving actionable insights that can steer the course of fraud detection."
Incorporating these modern techniques in fraud detection ensures that financial institutions stay ahead of fraudsters. The integration of machine learning applications and data analytics tools establishes a solid foundation. As the fraud landscape continues to evolve, so must the strategies employed to counteract threats.
Role of Artificial Intelligence
Artificial Intelligence (AI) plays an increasingly pivotal role in enhancing the capabilities of fraud detection systems within financial services. As financial fraud evolves in complexity, implementing AI becomes not just advantageous but vital. The technology offers various benefits, enabling institutions to keep pace with fraudsters who are constantly refining their tactics. By leveraging AI, financial institutions can improve detection mechanisms, better manage risks, and ultimately, safeguard their operations more effectively.
Enhancing Detection Accuracy
One of the most significant contributions of AI in fraud detection is the enhancement of detection accuracy. Traditional methods often rely on predefined rules and thresholds, resulting in limitations that hinder their effectiveness. This is where machine learning algorithms step in. They analyze vast datasets to identify patterns and anomalies that human analysts or rule-based systems might overlook.
- Continuous Learning: AI systems learn from new data, continuously improving their accuracy. They're not static; they adapt as they encounter fresh data and scenarios.
- Behavioral Analytics: By examining user behavior over time, AI can establish a baseline. Any significant deviation from this baseline potentially triggers alerts for further investigation. This nuance allows financial institutions to catch suspicious activities that are cleverly disguised as legitimate transactions.
- Predictive Models: These models anticipate future fraudulent activities by analyzing historical data, thereby allowing institutions to act preemptively rather than reactively.
This focused scrutiny leads to a smarter detection process that not only identifies fraud more accurately but also enhances overall risk management strategies.
Reducing False Positives
Another compelling advantage of integrating AI into fraud detection is its ability to effectively reduce false positives. Traditional systems often raise alarms for transactions that, on further inspection, turn out to be legitimate. This not only frustrates customers but also burdens human analysts with an overwhelming volume of investigations.
- Intelligent Scoring Systems: AI algorithms can assign risk scores based on multiple variables, allowing a more nuanced assessment of each transaction. This scoring helps prioritize investigations, targeting those most likely to be fraudulent.
- Contextual Analysis: AI can consider the context in which a transaction occurs. For instance, if a customer frequently travels and suddenly makes a large purchase from a new location, AI recognizes this as a possible red flag, but also weighs the pattern against the user's historical behavior.
- Tailored Fraud Models: With AI, institutions can create customized fraud detection solutions tailored to their unique clientele and transaction types. This leads to a marked reduction in unnecessary alerts.
By decreasing the rate of false positives, financial institutions not only streamline operations but also enhance customer satisfaction since clients are less likely to face unwarranted scrutiny.
"The integration of AI in fraud detection is not merely a technological upgrade; it's a paradigm shift. It transforms how financial institutions protect their assets and foster trust."
In summary, the role of Artificial Intelligence in fraud detection is multifaceted, encompassing better accuracy in identifying real threats while minimizing the disruption caused by erroneous alerts. As technology progresses, its importance will only deepen, shaping the future of secure financial transactions.
Framework for Effective Fraud Detection
Establishing a sound framework for effective fraud detection is critical in today's financial landscape. This framework acts as a comprehensive guide, helping institutions navigate the often murky waters of financial fraud. It encompasses technology, people, processes, and partnerships, creating a multi-faceted approach to tackling fraud. A well-structured framework not only aids in timely detection and response but also fosters a culture of proactivity, essential for long-term success.
Building a Comprehensive Strategy
Integration of Technology
When it comes to the integration of technology, it's about harnessing tools that can amplify the fraud detection process. Financial institutions are now squeezing the juice out of sophisticated tech—think machine learning algorithms, advanced analytics, and even AI bots. These technologies can analyze vast amounts of data quickly and accurately, spotting atypical patterns that may suggest fraudulent activity.
A key characteristic of this integration is its adaptability. Systems must continually evolve to keep up with the rapid pace of both technology and fraud. Organizations can adopt technologies like automated monitoring systems, which not only save time but also significantly increase detection rate. However, it’s important to note the potential initial costs and the need for technical know-how, which can be a financial and training burden on smaller institutions. Still, those willing to invest can see a payoff in better fraud response times and more secure transactions.
Ongoing Training for Staff
Ongoing training for staff plays a crucial role in any fraud detection strategy. Staff members, especially in operational roles, are the first line of defense against fraud. Regular training sessions can help them recognize suspicious behavior and improve their ability to respond effectively. Furthermore, keeping them updated on new fraud trends keeps everyone on their toes—that's the goal here.
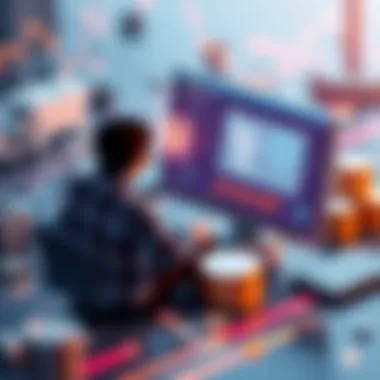
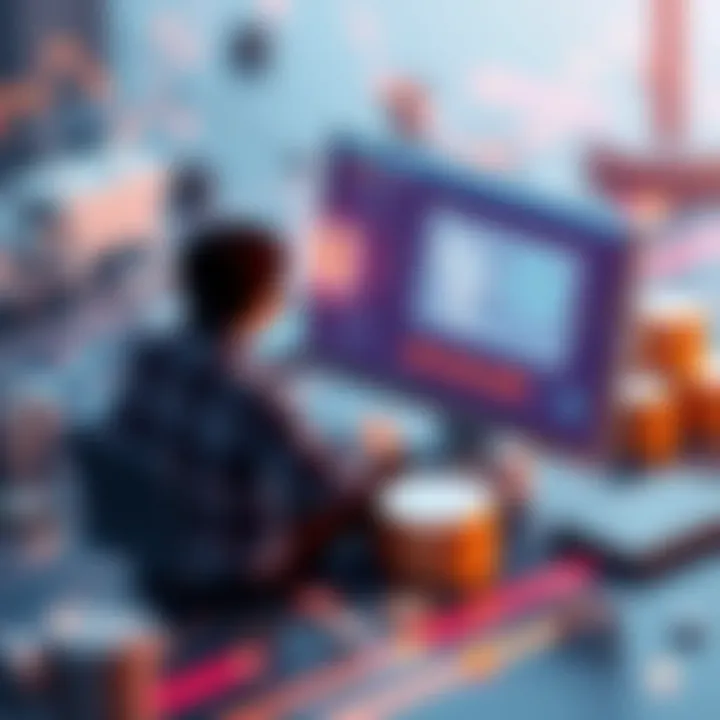
This training is beneficial simply because it fosters awareness. Employees become vigilant, understanding that they play an instrumental part in preventing fraud. One unique feature of ongoing training is its evolving curriculum. As fraud tactics get more sophisticated, so does the training, which can include real-life simulations or case studies to provide practical knowledge. However, implementing an ongoing training program requires dedication and resources, which some firms may struggle with.
Collaboration with Regulatory Bodies
Sharing Best Practices
Collaboration with regulatory bodies allows financial institutions to engage in sharing best practices, thus creating a healthy ecosystem for fraud detection. By pooling collective knowledge, firms can learn from one another’s experiences, adopting strategies proven effective in mitigating risk. This connection also enhances communication, meaning firms stay abreast of regulatory updates that might affect their operations.
A major strength of sharing best practices is the accelerated learning curve it provides. When entities band together, they are not just working in silos; instead, they bring diverse perspectives to the table. However, it can be tricky to balance the competition that exists in finance with the collaboration needed to tackle fraud effectively. Partners must ensure this sharing doesn’t lead to a loss of competitive advantage.
Ensuring Compliance Standards
Ensuring compliance standards is not just a box-ticking exercise; it's essential for fortifying fraud detection processes. Financial institutions must continually adapt to ever-shifting regulations which govern their operations to protect themselves and their clients. Striving for top-notch compliance can greatly reduce vulnerabilities to fraud, aligning operational practices with legal requirements.
One distinguishing feature of adhering to compliance standards is the structured approach it provides. It creates a clear roadmap for institutions to follow, reducing ambiguity in policy implementation. On a practical level, this can mean additional training expenses or audits; still, the long-term benefits outweigh these costs as they build trust with customers and regulators alike. However, compliance can sometimes feel cumbersome, which is a potential disadvantage as it may hinder agility or speed in responding to fraud.
Effective fraud detection isn't merely about technology and numbers; it's about creating a culture of vigilance, collaboration, and continuous learning.
Challenges in Fraud Detection
The landscape of financial fraud detection is in a constant state of flux, presenting a myriad of challenges that financial institutions must navigate. Understanding these obstacles is vital for developing robust strategies to combat fraud. The increasing sophistication of fraudsters, coupled with the ever-evolving technology available to them, makes it paramount for institutions to stay ahead in their detection methods.
Evolving Tactics of Fraudsters
Fraudsters are not merely opportunists; they are strategic thinkers who adapt quickly to changing environments. As institutions fortify their defenses, these criminals are simultaneously innovating their tactics.
- Cybercrime: With the rise of digital banking, fraudsters have leveraged technology, employing techniques such as phishing, malware, and social engineering to exploit vulnerabilities in systems. This approach not only swindles money but also erodes customer trust.
- Synthetic Identity Fraud: In this method, fraudsters create fictitious identities using real and fabricated information. This tactic is particularly challenging to detect as it targets gaps in existing identification processes within institutions.
- Account Takeover: Fraudsters may often hijack existing accounts through stolen information. This type of fraud is alarming because it directly impacts customer assets and can happen swiftly, leaving institutions little time to respond.
As fraudsters evolve their games, institutions must continuously enhance their detection capabilities, blending technology with human oversight to combat these ever-changing techniques.
Integration of Legacy Systems
A significant hurdle in fraud detection lies in the integration of legacy systems. Many financial institutions rely on long-standing systems that were not designed for modern cybersecurity challenges.
- Inflexibility: These systems often come with rigid architectures that limit their ability to adapt to new threats or incorporate advanced detection technologies. This restricts the institution's agility in responding to fraud.
- Data Silos: Legacy systems can create barriers in data sharing across departments. This siloed data can hinder comprehensive analysis, making it difficult to spot patterns or anomalies indicative of fraud.
- High Maintenance Costs: Older systems may require substantial resources to maintain, diverting funds from innovative fraud prevention measures that could be more effective.
Tackling the challenges posed by outdated systems is critical for financial institutions aiming to implement an effective fraud detection framework.
"As technology advances, so too must our approaches to fraud detection; failing to adapt is a surefire way to be left behind."
Future Trends in Fraud Detection
Fraud detection is an ever-evolving field, primarily due to the sophisticated techniques that fraudsters employ to exploit vulnerabilities. Keeping abreast of future trends is crucial for financial services, as it enables institutions to adapt their strategies and bolster their defenses. This article will delve into emerging technologies and the role of big data, shedding light on how these factors are reshaping the landscape of fraud detection.
Emerging Technologies
Blockchain Technology
Blockchain technology has become synonymous with security and transparency. One key characteristic of Blockchain is its decentralized nature, which records transactions across many computers, making it almost impossible for any single entity to alter the data. This feature allows for a tamper-proof ledger that aids in audit trails, thus significantly lowering the risk of fraud within financial dealings.
Use of Blockchain can enhance transaction verification processes. In this article, Blockchain's robust security is highlighted, as its foundational principle—immovable data—makes it a particularly appealing choice for fraud detection. Its main advantage lies in the fact that every participant in a transaction can see the details, reducing discrepancies and potential fraudulent activities.
However, it's essential to point out some concerns. The technology isn't fully mature, which may lead to integration challenges with existing systems, not to mention potential scalability issues for larger organizations.
Biometric Authentication
Biometric authentication is another leap forward in consumer verification methods. This technology fundamentally relies on unique traits like fingerprints, facial recognition, or retinal scans. A crucial aspect of this method is its convenience; individuals no longer need to remember passwords or security questions, providing a seamless user experience. This feature makes biometric authentication increasingly popular in the financial sector, ensuring both security and user satisfaction.
Incorporating biometric solutions into fraud detection enables financial institutions to authenticate users more accurately. With lower chances of ID theft compared to traditional methods, this technology protects both customers and institutions alike. Yet, there's a flip side: biometric data requires robust storage and handling protocols to protect against breaches. Once compromised, unlike a password, biometric data cannot be changed, leading to irreversible risks.
The Role of Big Data
Data Harvesting Techniques
In the finance world, data harvesting techniques allow organizations to collect vast amounts of user data from various sources. Notably, the ability to gain insights from both structured and unstructured data boosts the chances of identifying patterns indicative of fraudulent behavior. Understanding this aspect positions financial institutions to pre-empt fraudulent activities before they escalate.
Key characteristics of these techniques often involve using algorithms that sift through millions of data points to detect anomalies. With this capability, organizations can build a more comprehensive view of their customer base, aligning their fraud prevention efforts accordingly. The upside is clear: organizations better equipped with data insights can respond faster to potential threats.
Yet, along with these advantages, challenges arise. Legal and ethical considerations surrounding data privacy cannot be overlooked. Balancing effective fraud detection with respecting user privacy remains a significant hurdle for many institutions.
Predictive Analytics
Predictive analytics significantly elevates the fraud detection game. By leveraging historical data, predictive models can forecast potential fraudulent transactions based on patterns and behaviors previously recorded. This analytical prowess offers institutions a proactive stance rather than a reactive one.
Institutions can integrate predictive analytics to reinforce their defenses, allowing them to assess risk profiles and prioritize alerts efficiently. This method is particularly beneficial as it creates a more intuitive, automated response that doesn't rely solely on human review.
However, the reliance on historical data introduces potential biases, which could lead to inaccurate predictions. Therefore, constant model refinement is necessary to maintain relevance and avoid misidentifications, a balancing act that carries its own challenges.