Exploring Types of Predictive Maintenance in Industry
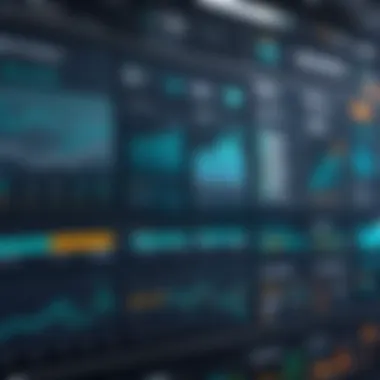
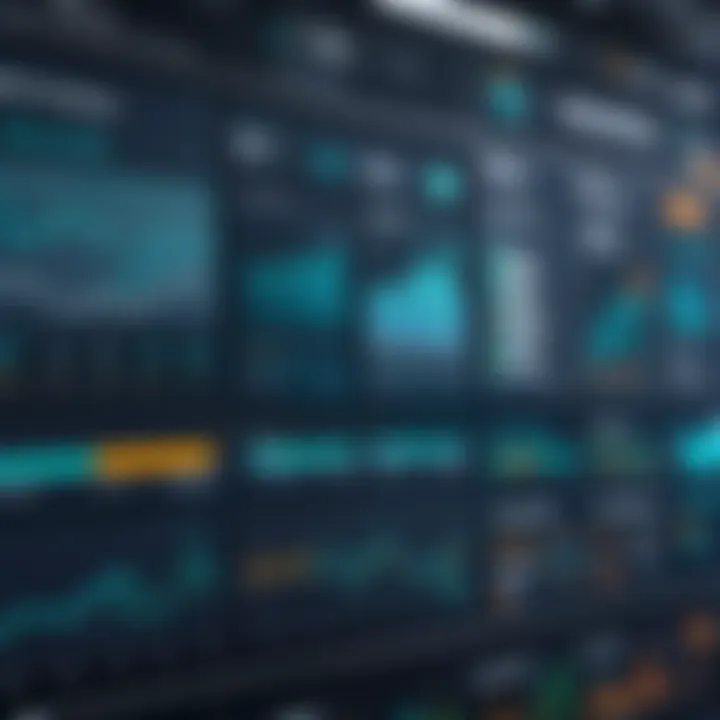
Intro
Predictive maintenance is an evolving field crucial for many industries today. The growing reliance on technology places emphasis on ensuring that systems run efficiently, minimizing downtime. Predictive maintenance involves utilizing data analysis and real-time monitoring to foresee potential equipment failures and address them preemptively. This not only reduces maintenance costs but also enhances overall operational effectiveness.
Industries ranging from manufacturing to transportation have already adopted various predictive maintenance strategies. The relevance of this topic cannot be overstated, as organizations seek ways to maintain a competitive edge while reducing unnecessary expenditure.
In this article, we will systematically explore the different types of predictive maintenance, highlighting key methodologies and their use cases.
Key Features and Functionalities
Comprehensive Overview
Understanding predictive maintenance involves grasping the methodologies designed to strengthen operational efficiency and extend machinery life. Typically included are:
- Condition Monitoring: Regular inspection of the operational condition of equipment using sensors and IoT devices.
- Data Analytics: Applying statistical tools to analyze trends and patterns in equipment performance.
- Failure Mode Analysis: Identifying key components that are likely to fail based on historical data.
These features aid organizations in foreseeing issues before they escalate, thus protecting investments and optimizing performance.
Target Users
Target users of predictive maintenance include a variety of stakeholders, such as:
- Decision-Makers: Executives prioritize strategies that support financial growth through efficient maintenance efforts.
- IT Professionals: Technicians and engineers need to implement systems that monitor and analyze equipment performance effectively.
- Entrepreneurs: Business owners seek cost-effective solutions that enhance their operational capacity and reliability.
As predictive maintenance is adopted more broadly, understanding its key functionalities shapes how users can leverage its benefits.
Pricing Models and Cost Analysis
Breakdown of Pricing Tiers
The cost of implementing predictive maintenance can vary significantly based on the specific technologies and methodologies employed. Major pricing components include:
- Software Licensing: Subscriptions or one-time fees for predictive maintenance software.
- Hardware Investments: Costs associated with necessary sensors and monitoring equipment.
- Training Programs: Investment in training employees on new systems and technologies.
Additional Costs to Consider
In addition to the initial setup costs, users should also be aware of ongoing expenses:
- Maintenance of Equipment: Regular upkeep needed for sensors and monitoring devices.
- Software Updates: Associated costs with keeping systems current.
- Operational Costs: Personnel time spent on monitoring and analyzing data.
Awareness of these factors helps organizations budget more effectively for predictive maintenance implementation.
"Predictive maintenance minimizes downtime, reduces costs, and improves operational performance โ a triad that enhances productivity in any industry."
Through these insights, the importance of predictive maintenance becomes clear. It serves as a vital tool in modern industries, encouraging a proactive rather than reactive approach to machinery and equipment management.
Foreword to Predictive Maintenance
Predictive maintenance is a strategic approach that harnesses data and analytical techniques to anticipate equipment failures in advance. It has gained significant importance in modern industries due to its potential to enhance operational efficiency. By aiming to minimize downtime, predictive maintenance plays a critical role in ensuring seamless operations. The ability to predict when maintenance is needed rather than relying on scheduled checks reduces costs associated with unexpected equipment failures. This method not only improves reliability but also extends the life cycle of machinery.
Definition and Importance
Predictive maintenance refers to the practice of monitoring the condition of equipment to predict when maintenance should be performed. The main goal is to prevent unforeseen failures and reduce the total cost of maintenance. This approach utilizes various techniques, including data analytics, machine learning, and real-time monitoring tools. A key benefit of predictive maintenance is that it shifts the focus from reactive or scheduled maintenance to a more proactive stance.
This shift aligns well with the goals of many organizations today. With the rising costs in maintenance and operation, finding efficient ways to manage equipment is more crucial than ever. Additionally, predictive maintenance significantly improves safety by reducing the likelihood of accidents caused by malfunctioning equipment. The overall improvement in productivity leads to favorable economic outcomes.
Historical Context
The evolution of predictive maintenance traces back several decades. Initially, maintenance strategies were largely reactive, relying on equipment failures to determine when to perform servicing. This often led to costly downtimes and inefficient operations. As technology advanced, particularly with the rise of computing power and data analytics, there was a gradual shift towards more sophisticated methods.
In the late 20th century, industries began adopting condition-based maintenance. This methodology focused on monitoring specific equipment conditions to determine maintenance needs. The continuous advancements in sensors, data collection, and analytical capabilities have propelled the development of predictive maintenance. Today, organizations leverage real-time data from various sources, such as IoT devices, to forecast equipment requirements accurately.
"Predictive maintenance not only saves costs but also enhances the overall reliability of equipment, a need that industries cannot afford to overlook."
Embracing predictive maintenance strategies enables companies to stay competitive in a rapidly changing market. Understanding the historical context highlights how far the industry has come and sets the stage for exploring the different methodologies available today.
Key Concepts in Predictive Maintenance
Predictive maintenance fundamentally reshapes how organizations manage their assets. To enhance overall efficiency and minimize unplanned downtime, itโs critical to grasp the key concepts of this discipline. These concepts form the backbone of predictive maintenance strategies, driving organizations toward smarter operations and improved reliability.
Data Analysis and Analytics
Data analysis is a cornerstone of predictive maintenance. It involves gathering vast amounts of information from various sources, including sensors, operational records, and historical maintenance data. The objective is to derive actionable insights that inform maintenance decisions.
An important aspect of this analysis is the ability to detect patterns and anomalies in equipment behavior. By employing sophisticated algorithms, organizations can recognize early warning signs of potential failures. This proactive approach shifts maintenance from reactive to predictive, ensuring that maintenance tasks are performed only when necessary.
Analytics tools can also segment data to enable focused insights. For example, using real-time dashboards, technicians can monitor equipment health dynamically. This facilitates timely interventions, prevents costly downtimes, and extends the lifespan of critical machinery.
Implementing data analytics yields numerous benefits:
- Enhanced Operational Efficiency: Regular updates on equipment status foster informed decision-making.
- Cost Reduction: Predictive maintenance reduces the costs associated with unplanned downtime and resource wastage.
- Safety Improvements: Predictive insights can preempt hazardous equipment failures, enhancing workplace safety.
Machine Learning and AI Integration
The integration of machine learning and artificial intelligence into predictive maintenance elevates its effectiveness. Machine learning algorithms enable systems to learn from existing data, allowing for improved predictions without extensive manual programming.
For instance, employing AI-driven models can automate the analysis of complex datasets. These models can adapt over time, continually refining their predictions based on incoming data. This aspect is crucial as it can help organizations forecast maintenance needs more accurately, adjusting to equipment wear patterns and operational changes dynamically.
Moreover, machine learning can facilitate scenarios where traditional statistical methods fall short. It can process nonlinear relationships fed by vast amounts of diverse data. This results in insights that are both deeper and richer, ultimately benefiting the decision-makers.
Some notable advantages of integrating these technologies include:
- Predictive Accuracy: Increased precision in predicting equipment failures.
- Scalability: Systems can effectively scale with expanding data sources, accommodating growth seamlessly.
- Resource Optimization: Better allocation of maintenance resources leads to efficiency gains across operations.
Ultimately, understanding these concepts creates a framework for informed decision-making in predictive maintenance. This framework equips businesses to face the challenges of modern operations and leverage data to enhance efficiency and reliability.
"The shift from reactive to predictive maintenance is not merely evolution; it is a revolution in how organizations approach asset management."
With the frameworks of data analysis and AI integration in place, organizations can unlock the true potential of predictive maintenance, shifting from mere maintenance management towards a strategic operational advantage.
Condition-Based Maintenance
Condition-Based Maintenance (CBM) serves as a cornerstone of predictive maintenance strategies. It prioritizes the real-time condition of equipment rather than relying solely on fixed time schedules for maintenance tasks. This approach offers several compelling benefits. Firstly, it enhances safety by addressing potential issues before they escalate into more significant problems. Secondly, it reduces unnecessary maintenance interventions, which can incur labor costs and disrupt operations. The third benefit lies in extending the asset's lifespan, as timely interventions lead to improved performance and more efficient usage of resources.
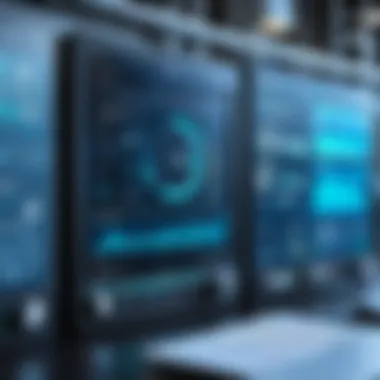
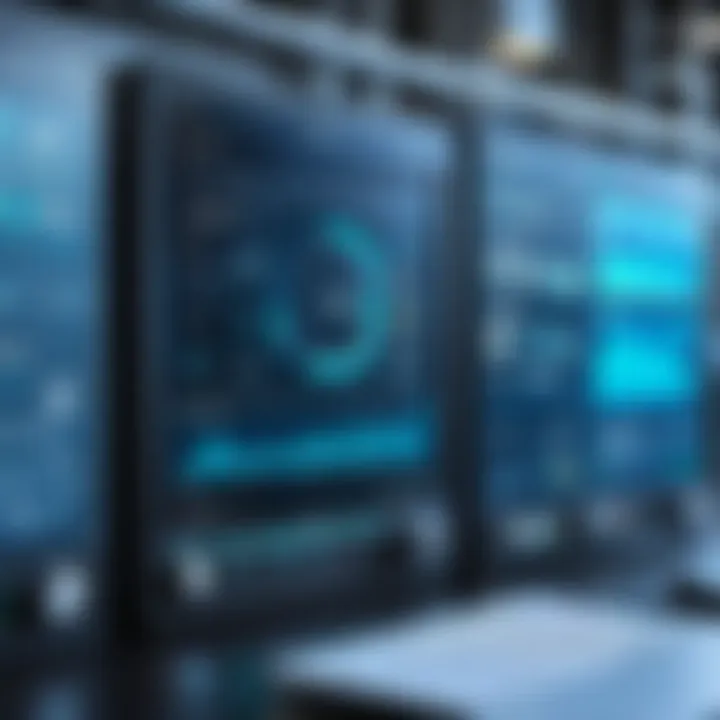
In this article, we will delve into the various aspects of Condition-Based Maintenance. By understanding its integral role within predictive maintenance, industries can adopt more effective maintenance strategies. CBM optimally aligns resources with operational activities, making it a valuable approach in today's competitive landscape.
Overview of Condition Monitoring
Condition monitoring is the practice of measuring specific parameters to assess the health of a piece of equipment. It often involves the continuous observation of machinery performance through various sensors and instruments. These parameters may include temperature, vibration, noise levels, and fluid conditions. By actively monitoring these conditions, organizations can detect anomalies and address potential failures in advance.
Condition monitoring can be integrated into existing systems without significant capital investment. This integration allows for ongoing data collection, which aids in trend analysis and decision-making. It provides insights that are beneficial not only for maintenance engineers but also for management teams who aim to optimize efficiency. Moreover, modern IoT devices often play a crucial role in condition monitoring due to their ability to transmit real-time data seamlessly.
Techniques and Tools
A variety of techniques and tools are employed in Condition-Based Maintenance. Some of the prominent methods include:
- Vibration Analysis: This technique helps in assessing mechanical imbalances or misalignments by analyzing the vibration data from rotating machinery. It is an effective way of early detection of wear and tear in components.
- Thermal Imaging: This method uses infrared cameras to detect heat patterns in equipment. Unusual heat signatures can indicate potential failures, making it critical for electrical components.
- Ultrasonic Testing: Ultrasonic sensors can identify leaks in systems that may not be visibly detectable. They convert sound waves into images or data, facilitating analysis.
- Oil Condition Monitoring: Regular analysis of oil can reveal the condition of engine components. Monitoring properties such as viscosity and contaminant levels is crucial for maintenance planning.
In addition to these techniques, a robust set of tools exists to assist maintenance teams. Industrial analytics software, cloud-based platforms, and data visualization tools help streamline the gathering and interpretation of monitoring data. By using these advanced tools, organizations can improve their decision-making process and maintain operational efficiency to a considerable extent.
"Condition-Based Maintenance not only enhances machinery reliability but also optimizes resource allocation, making it a smart choice for industries aiming for greater efficiency."
Predictive Modeling Techniques
Predictive modeling techniques play a crucial role in predictive maintenance by providing data-driven insights into equipment performance and potential failures. These techniques leverage historical data to anticipate future operational conditions and help organizations make informed decisions about maintenance activities. By implementing these modeling techniques, businesses can enhance their maintenance strategies, leading to increased efficiency and reduced downtime.
Statistical Methods
Statistical methods form the foundation of predictive modeling. They utilize mathematical models to analyze historical data and extract meaningful patterns. For instance, regression analysis can uncover relationships between various operational parameters and equipment failures. This approach allows professionals to predict the remaining useful life of assets, thereby enabling timely interventions.
Statistical methods can also help delineate the normal operating range of equipment. By identifying outlier conditions, organizations can take proactive measures to prevent failures. Common techniques in statistical analysis include:
- Regression Analysis: Determines the relationship between variables.
- Time Series Analysis: Examines data points collected over time to forecast future values.
- Control Charts: Monitors process variation over time, helping to signal when maintenance is needed.
These methods are especially useful because they provide a quantitative basis for decision-making.
Simulation-Based Approaches
Simulation-based approaches offer another layer of predictive modeling. These methods involve creating detailed models of systems or processes to evaluate various scenarios without physical experimentation. Using computer simulations, organizations can replicate and study the operational conditions of their machinery.
This approach is advantageous because it allows what-if analysis, helping to understand the impact of different variables on equipment performance. For example, using tools like Monte Carlo simulations, organizations can predict the probability of failure under various conditions.
The key benefits of simulation-based approaches include:
- Risk Assessment: Evaluating different scenarios helps identify potential risks and their impacts.
- Resource Optimization: Simulations can reveal the most efficient use of resources in maintenance processes.
- Cost Reduction: By predicting failures ahead of time, organizations can schedule maintenance activities in a way that minimizes disruption and costs.
These modeling techniques are essential in the field of predictive maintenance, as they provide the tools necessary to analyze, predict, and optimize machine performance. By combining statistical methods with simulation-based approaches, decision-makers can obtain a comprehensive understanding of their assets and enhance the effectiveness of maintenance programs.
Vibration Analysis
Vibration analysis plays a crucial role in predictive maintenance strategies. As machinery operates, it produces vibrations that can indicate the health status of the equipment. By monitoring these vibrations, organizations can identify irregularities before they lead to significant failures. This proactive approach contributes to maintaining the reliability of assets while optimizing operational efficiency.
Vibration analysis assists in recognizing patterns in machinery behavior. It allows engineers to monitor specific metrics, such as frequency, amplitude, and intensity of vibrations. The insights derived from analyzing these metrics can result in substantial cost savings through reduced downtime and extended equipment lifespan. Moreover, consistent vibration monitoring can help in pinpointing the root cause of mechanical issues, leading to more informed decision-making around maintenance interventions.
Principles of Vibration Monitoring
Vibration monitoring relies on various principles that help to accurately assess the condition of machinery. The primary concept involves measuring the vibration signals produced by rotating equipment. Typically, accelerometers are used to capture the vibrational energy and convert it into electronic signals. These signals then undergo analysis to determine the health of the equipment.
Key principles involved in vibration monitoring include:
- Frequency spectrum analysis: By evaluating the frequency at which vibrational energies occur, analysts can identify specific issues such as imbalance, misalignment, or wear in components.
- Time domain analysis: This method focuses on measuring vibrational data over specified time intervals, identifying transient events, and providing insights into machinery behavior.
- Envelope analysis: This technique enhances the detection of faults by isolating specific frequency components, making it easier to analyze certain conditions like bearing failures.
These principles guide the systematic monitoring and interpretation of vibration data, resulting in actionable insights that can inform maintenance actions.
Applications and Benefits
Vibration analysis is widely applied across various industries, offering distinct benefits that enhance maintenance practices. This technique is commonly used in sectors such as manufacturing, power generation, and aerospace. Here are some notable applications and their associated benefits:
- Application in manufacturing: Vibration analysis can identify potential failures in industrial machinery, thus preventing costly production halts. Continuous monitoring enables manufacturers to refine their maintenance schedules, aligning them with actual equipment condition.
- Power generation efficiency: Power plants operate heavy machinery that is critical for energy output. By employing vibration analysis, operators can detect early signs of failure, ensuring consistent energy generation and reducing operational risks.
- Aerospace safety: In aviation, ensuring the reliability of components is paramount. Continuous vibration monitoring can enhance safety protocols by identifying faults that may compromise aircraft operation.
- Cost savings: Ultimately, the benefits of vibration analysis extend to significant financial advantages. With consistent monitoring and analysis, companies minimize repair costs and mitigate the risk of unexpected downtime, translating to improved profitability.
In summary, vibration analysis is an indispensable tool in predictive maintenance. By understanding its principles and applications, organizations can leverage this technique to benefit from enhanced equipment reliability and operational efficiency.
Thermal Imaging
Thermal imaging plays an essential role in the field of predictive maintenance. This technology leverages infrared sensors to detect heat emitted by machinery and electrical equipment. By monitoring thermal patterns, organizations can identify issues before they result in equipment failure. The critical advantage of thermal imaging lies in its ability to provide a non-invasive and real-time analysis of equipment health. This capability is invaluable for maintaining operational efficiency and averting costly downtimes.
Fundamentals of Thermal Monitoring
Thermal monitoring involves the use of thermal cameras to visualize temperature variations across equipment surfaces. These cameras capture infrared radiation, which is then converted into visible images. Each color in the thermal image represents a different temperature range. Understanding these variations is vital for maintenance personnel. For example, higher temperatures can signify friction in bearings or electrical overloads.
The process can be summarized as follows:
- Data Collection: Thermal cameras capture images, often as part of a routine inspection schedule.
- Analysis: The thermal images are analyzed to detect abnormal spikes in temperature levels.
- Action: Upon identifying a problem, maintenance teams can investigate further and schedule necessary repairs before failures occur.
Technology has advanced significantly, leading to more accessible thermal monitoring solutions. The integration of thermal imaging with digital platforms enhances data sharing and analysis, allowing teams to track machinery performance over time. This proactive approach leads to reduced wear and tear on equipment, promoting longevity.
Use Cases in Predictive Maintenance
Thermal imaging is employed across various industries for predictive maintenance, and its applications are diverse. Here are a few notable use cases:
- Manufacturing: In manufacturing setups, thermal imaging is often used to monitor motors and gearboxes. Overheating in these components can directly impact production efficiency.
- Electrical Systems: Electrical panels and connections are prime candidates for thermal analysis. Hot spots in electrical systems can signal problems such as loose connections or excess load.
- HVAC Systems: Heating, ventilation, and air conditioning units rely on thermal monitoring to ensure optimal performance. Overheating in these systems can lead to operational inefficiencies and costly breakdowns.
- Building Inspections: Thermal imaging identifies insulation failures and air leaks in buildings. This application promotes energy efficiency by ensuring temperature stability.
"Thermal imaging effectiveness hinges on regular monitoring and analysis, transforming how maintenance teams address equipment health issues."
By implementing thermal imaging into a predictive maintenance strategy, organizations can expect significant improvements in operational continuity. The insights gained through this technology provide a competitive edge, allowing firms to act swiftly in addressing potential failures. In summary, thermal imaging serves as a vital tool for proactive maintenance, fostering enhanced efficiency and extended asset life.
Ultrasonic Testing
Ultrasonic testing is a non-destructive testing method that uses high-frequency sound waves to detect imperfections, measure thickness, and analyze material properties. Its significance in predictive maintenance lies in its ability to identify faults before they evolve into critical failures. By implementing ultrasonic testing, industries can enhance safety, optimize maintenance schedules, and extend the lifespan of equipment. The precision of this method helps minimize unexpected downtime and maintenance costs, making it a valuable tool in various sectors such as manufacturing, aerospace, and energy.
Principles of Ultrasonic Testing
The core principle of ultrasonic testing is based on the propagation of sound waves through materials. A transducer generates ultrasonic waves and transmits them into the material being tested. When these waves encounter discontinuities, such as cracks or voids, they reflect back to the transducer, which converts the signals into a visual representation for evaluation. The primary methods used include:
- Pulse-Echo Technique: This method sends a pulse of sound into the material and measures the time taken for echoes to return. It helps detect flaws and measures the thickness of materials.
- Through-Transmission Method: In this approach, the sound waves pass through the object from one transducer to another, gauging the materialโs integrity.
- Phased Array Ultrasonic Testing: This advanced technique utilizes multiple transducer elements to produce a series of beams, allowing for detailed imaging of complex geometries.
Each method has its specific applications and advantages, contributing to better decision-making in predictive maintenance practices.
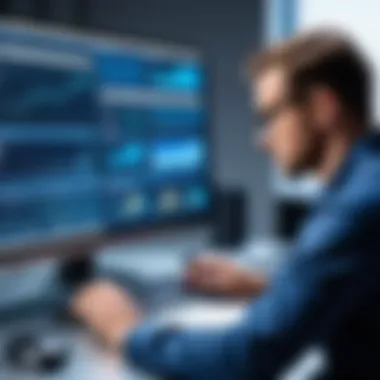
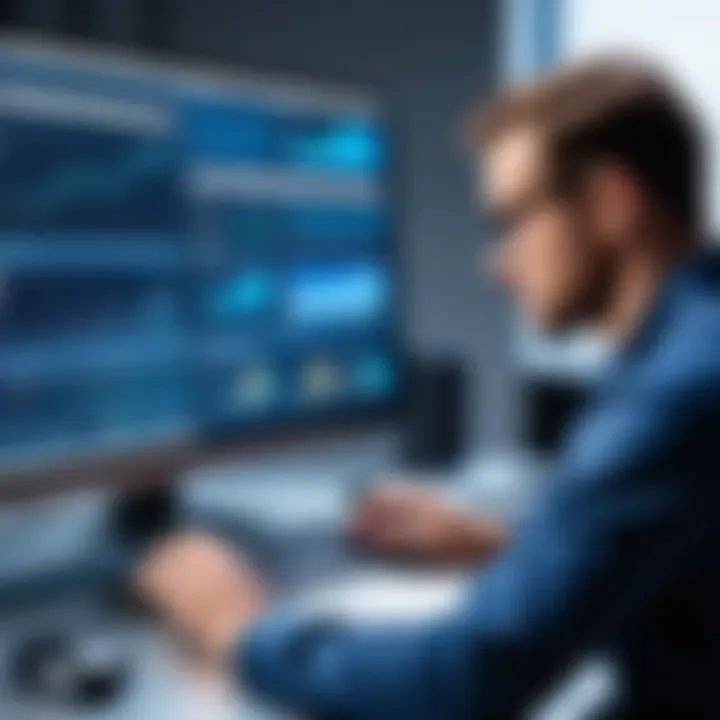
Advantages and Limitations
Advantages:
- High Sensitivity to Flaws: Ultrasonic testing can detect small defects that other methods may overlook.
- Flexible and Portable: Equipment can be easily transported, allowing for versatile applications across different environments.
- Quantitative Results: Measurements can be made with high accuracy, providing clear data for analysis.
- Non-Destructive: The testing does not damage the material, maintaining its usability after inspection.
Limitations:
- Surface Condition Requirement: The surface must be clean and smooth for accurate results, which might necessitate preparation.
- Operator Skill Dependency: The effectiveness of ultrasonic testing heavily relies on the operator's skill and expertise in interpreting data.
- Material Limitations: Certain materials, such as those with highly coarse grain or those that are very thin, may present challenges in achieving reliable readings.
Oil Analysis
Oil analysis serves a pivotal role in predictive maintenance. It involves monitoring the condition of lubricants used in machinery to predict potential failures and extend equipment life. The process is not merely about analyzing oil quality, but it also encompasses the examination of wear particles and contaminants. Effective oil analysis can significantly reduce downtime and maintenance costs, thus improving operational efficiency.
Key benefits of oil analysis include:
- Early Detection of Issues: Regular monitoring allows for the identification of problems before they escalate into major failures.
- Maximized Equipment Lifespan: By ensuring lubricants are in optimal condition, machinery operates smoothly, minimizing wear and tear.
- Cost Savings: The incidental expense of oil testing is often overshadowed by the savings gained from preventing catastrophic equipment failure.
The considerations for implementing oil analysis include choosing the right testing frequency and understanding how the data aligns with machinery specifics. Organizations must also ensure they have trained personnel capable of interpreting the results correctly.
Overview of Oil Condition Monitoring
Oil condition monitoring employs various strategies to analyze lubricant health. This can include physical tests such as viscosity, acid number, and moisture content analysis. Chemical tests examine contaminants and wear metals present in the oil itself. By assessing these factors, maintenance teams can gauge lubricant effectiveness over time.
Key components of an oil condition monitoring program consist of:
- Sampling Schedule: Establishing a routine for sample collection is necessary. Too infrequent testing may miss critical changes, while too frequent testing could lead to unnecessary costs.
- Analysis Methods: Diverse methods are available, ranging from simple visual inspections to complex spectrometric analysis, tailoring approaches to specific operational scenarios.
- Data Integration: Collecting data in a centralized manner for analysis helps in spotting trends and making informed decisions.
Interpretation of Analysis Results
Interpreting oil analysis results requires a clear understanding of baseline values for the specific equipment in question. Key parameters like Total Acid Number (TAN), particle count, and elemental analysis give insight into the state of the lubricant.
Factors for accurate interpretation include:
- Trend Analysis: Examine shifts over time rather than single data points. Observing trends can reveal whether a lubricant is degrading or if contamination is worsening.
- Benchmarking: Compare results against industry standards or manufacturer recommendations to assess adequacy.
- Action Plans: Clearly defined response plans based on results enable proactive maintenance strategies. For example, if rising wear metals are detected, specific actions should be taken before severe wear occurs.
Oil analysis transforms the approach to maintenance, allowing businesses to turn reactive strategies into proactive, data-driven solutions.
Integration of IoT in Predictive Maintenance
The integration of Internet of Things (IoT) in predictive maintenance is foundational for enhancing efficiency and operational effectiveness in various industries. It brings numerous benefits, such as real-time monitoring, data-driven insights, and improved resource management. IoT devices are taking center stage in the landscape of predictive maintenance, providing tools that empower organizations to transition from reactive maintenance to more proactive strategies.
Role of IoT Devices
IoT devices play a massive role in predictive maintenance by establishing a network of interconnected sensors and instruments. These devices monitor equipment conditions continuously, gathering data on parameters like temperature, vibration, and noise. This data is transmitted to centralized systems for analysis. By utilizing devices such as smart sensors, wearables, and connected machinery, organizations can achieve a more real-time perspective of their assets.
- Smart Sensors: They provide accurate data about operational parameters. This information is crucial for identifying potential failures before they occur.
- Wearables: In certain applications, these devices can alert workers to equipment issues. If an operator is wearing a device that tracks machine performance, they get immediate alerts about any irregularities.
- Connected Machinery: Older machines can be upgraded with IoT retrofits, making it easier to integrate them into a modern predictive maintenance strategy.
"The deployment of IoT devices allows organizations to make informed decisions, significantly reducing maintenance-related costs and downtime."
Data Collection and Processing
Data collection and processing are critical components of predictive maintenance, especially when integrated with IoT. The volume of data generated by IoT devices can be enormous. Therefore, having an efficient system to process this data is vital.
- Data Sources: The data come from various sensors monitoring mechanical and operational indicators.
- Real-time Analytics: Companies utilize platforms that offer real-time analytics. This enables them to analyze incoming data to identify patterns and anomalies quickly.
- Cloud Computing: Many organizations utilize cloud-based solutions to store and analyze the data. This ensures accessibility and scalability, allowing for extensive data sets to be analyzed without the constraints of local storage.
- Algorithms and Models: Advanced algorithms are used to interpret the data. By leveraging machine learning techniques, organizations can predict when maintenance should be performed, which allows for better planning and resource allocation.
Integrating IoT devices streamlines data collection and processing. This enhances predictive capabilities, leading to effective maintenance strategies that not only minimize downtime but can also extend the lifespan of equipment.
Case Studies of Predictive Maintenance Implementation
In the realm of predictive maintenance, case studies serve as vital components for understanding the practical application and effectiveness of these strategies. As industries seek more cost-effective and efficient approaches to maintenance, examining real-world examples helps illuminate successful implementations. This section will delve into specific cases, highlighting benefits, considerations, and lessons learned across various sectors.
Successful Applications in Manufacturing
Manufacturing is one of the sectors that has reaped substantial benefits from predictive maintenance. The integration of predictive analytics into operations has transformed how production facilities manage their assets. For instance, a notable case is the implementation by General Electric in their industrial manufacturing plants. Through the use of advanced sensors and IoT technology, they successfully monitor the condition of machinery in real-time.
This approach has proven to reduce unplanned downtime significantly. GE reported a 10% reduction in maintenance costs after implementing predictive strategies. The predictive models developed allow for timely interventions, preventing equipment failures before they occur.
The success at GE emphasizes a few key elements:
- Data-Driven Decisions: Their predictive maintenance relies on comprehensive data analysis. By collecting data from equipment sensors, operators can make informed decisions about maintenance schedules.
- System Integration: The integration of predictive maintenance systems with existing operational technology is crucial. This ensures seamless communication across departments, enhancing overall efficiency.
- Training and Expertise: Success also hinges on providing training for staff. Familiarity with predictive tools allows technicians to respond quickly to alerts and interpret data accurately.
Impacts in Other Industries
Beyond manufacturing, predictive maintenance finds application in diverse fields such as energy, transportation, and healthcare. The energy sector, for instance, has seen significant advancements through predictive strategies. A case study worth noting involves Siemens' work in wind energy. They utilized predictive maintenance to monitor wind turbines effectively.
This initiative led to a 15% increase in operational efficiency due to reduced failures and minimized downtime for repairs. Analysts employed machine learning algorithms to predict potential issues based on weather patterns and operational data. This proactive approach to maintenance not only saved costs but also contributed to better resource management and sustainability in energy production.
In healthcare, predictive maintenance can enhance asset management in hospitals. A notable example includes the implementation by Cleveland Clinic. They have integrated predictive models to monitor medical devices and equipment. Ensuring that essential machinery operates efficiently has directly improved patient care outcomes.
Such successful applications across various industries showcase:
- Versatility: Predictive maintenance is adaptable, applicable in sectors beyond manufacturing.
- Enhanced Efficiency: Organizations can reduce operational disruptions, leading to more streamlined processes.
- Cost Reduction: By anticipating failures, companies can save on emergency repair costs and enhance the lifespan of assets.
"The insights from these cases highlight the transformative potential of predictive maintenance across industries, reiterating its role as a fundamental part of future operational strategies."
Through these examples, it is evident that the implementation of predictive maintenance can bring about substantial improvements in efficiency and effectiveness. By learning from these case studies, organizations can gain valuable insights that facilitate their journey toward adopting predictive maintenance practices. Blending technology with operational knowledge is essential for realizing the full benefits of predictive maintenance.
Challenges in Predictive Maintenance
Predictive maintenance offers numerous benefits, including reduced downtime and increased operational efficiency. However, implementing such strategies presents several challenges that must be addressed to fully realize these advantages. A thorough understanding of these challenges ensures that organizations can create effective solutions that harness the potential of predictive maintenance while mitigating risks associated with its adoption.
Data Privacy and Security Concerns
As industries lean more on data-driven maintenance strategies, the importance of data privacy and security cannot be overstated. Organizations gather large volumes of data from various sources, including machines, sensors, and IoT devices. This data often contains sensitive information that, if mishandled, could lead to significant privacy breaches.
There are regulatory frameworks such as GDPR that govern how data must be managed. Companies need to ensure compliance with these regulations to avoid costly penalties and maintain customer trust. Implementing strong cybersecurity measures is essential. This includes encrypting sensitive data, regularly updating security protocols, and training staff on best practices for data handling.
Key Actions for Data Security:
- Conduct regular data audits to assess vulnerabilities.
- Use encryption and secure networks for data transmission.
- Develop incident response plans for potential data breaches.
*"Data security is not just an IT issue; itโs a fundamental aspect of an organizationโs integrity and trustworthiness."
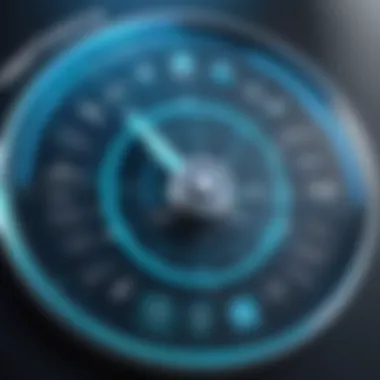
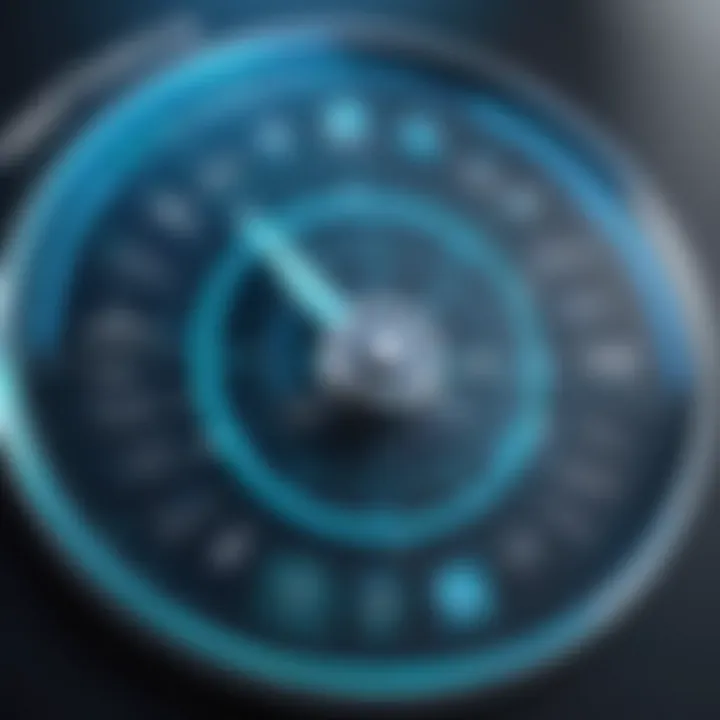
Integration with Existing Systems
The integration of predictive maintenance solutions with existing systems is another critical challenge. Many organizations use legacy systems that may not be fully compatible with new predictive technologies. This can lead to data silos, where valuable information is trapped in disparate systems, preventing holistic analysis.
Moreover, the complexity of integrating various systems can result in unanticipated downtime during the transition period. To address these issues, organizations should take a strategic approach to integration. This involves starting with thorough assessments of existing infrastructure, identifying compatibility issues early, and selecting scalable predictive maintenance solutions that offer interoperability.
Strategies for Successful Integration:
- Begin with pilot projects to gauge integration feasibility.
- Engage cross-functional teams to ensure all perspectives are considered.
- Opt for platforms that support data sharing across multiple systems.
By understanding and addressing these challenges, organizations can better position themselves to implement predictive maintenance effectively. Managing data privacy and seamless integration with existing systems will enhance the overall efficiency and effectiveness of maintenance strategies.
Regulatory Considerations
In the realm of predictive maintenance, regulatory considerations play a crucial role. This topic addresses the obligatory frameworks that organizations must adhere to. Ignoring these regulations may lead to severe penalties, jeopardized safety, and compromised reliability of systems. Therefore, understanding these regulations is essential for facilitating compliance and promoting best practices.
Compliance Requirements
Compliance requirements relate directly to legal obligations imposed on industries regarding equipment and maintenance standards. Each industry has specific guidelines that must be followed. These guidelines often stem from national or regional legislation aimed at ensuring safety and environmental sustainability.
Some key factors influencing compliance requirements include:
- Safety Standards: These standards ensure that equipment operates safely, minimizing risks to personnel and the environment.
- Environmental Regulations: Compliance with environmental laws protects against harmful emissions and waste disposal issues.
- Reporting Requirements: Regular reporting to regulatory bodies is often needed to demonstrate adherence to compliance standards.
Ensuring compliance establishes trust with clients and stakeholders, reinforcing an organizationโs reputation. Moreover, it aids in avoiding potential interruptions caused by penalties or shutdowns. Companies investing in predictive maintenance should, therefore, continually assess their compliance with prevailing laws and regulations to remain ahead of the curve.
Industry-Specific Regulations
Beyond broad compliance requirements, industry-specific regulations introduce additional criteria tailored to unique operational environments. Each sector may have distinct challenges and risks, leading to diverse regulatory stipulations.
Notable industry-specific regulations include:
- Healthcare: Regulations like HIPAA necessitate robust data privacy measures in predictive maintenance solutions that manage sensitive health information.
- Aerospace and Defense: The rigorous standards of FAA and DOD ensure that predictive maintenance not only enhances efficiency but also strengthens security protocols.
- Manufacturing: OSHA regulations focus on workplace safety and equipment reliability, shaping predictive maintenance strategies to prioritize hazard prevention.
Understanding and implementing these industry-specific regulations is vital for organizations. They ensure not just compliance but also that best practices are integrated into operational workflows.
Adhering to regulatory considerations helps organizations to mitigate risks while enhancing operational effectiveness and ensuring longevity through sustainable practices.
Economic Implications of Predictive Maintenance
The economic implications of predictive maintenance (PdM) are profound and multifaceted. They extend beyond mere cost savings to encompass enhanced operational efficiency, increased equipment lifespan, and a strategic competitive edge. This section delves deeper into these economic aspects, illustrating how implementing predictive maintenance can lead to a substantial return on investment and foster a culture of proactive maintenance rather than reactive fixes.
Cost-Benefit Analysis
A comprehensive cost-benefit analysis is critical in evaluating the effectiveness of predictive maintenance implementations. This analysis typically examines both direct and indirect costs associated with maintenance strategies.
Direct costs may include:
- Implementation costs: Initial investments in technology, training, and software systems.
- Operational costs: Ongoing expenses related to data collection and analysis tools.
Indirect costs, on the other hand, often reflect areas that might be overlooked in traditional maintenance strategies:
- Downtime reduction: Predictive maintenance significantly lowers downtime due to unexpected equipment failure. Minimizing loss in production time directly correlates to increased revenue.
- Extension of asset lifespan: Regular monitoring and maintenance can extend the lifespan of machinery, thereby deferring capital expenses for replacements.
Furthermore, a focused analysis must consider risk reduction. In industries where unscheduled maintenance can lead to safety risks or regulatory penalties, predicting failures brings peace of mind and can translate to lower insurance premiums or reduced legal liabilities. Therefore, the benefits, both tangible and intangible, should provide a solid foundation for the financial justification of predictive maintenance initiatives.
Return on Investment Metrics
Measuring the return on investment (ROI) for predictive maintenance can be complex. However, several key metrics have emerged to provide clarity:
- Reduced Maintenance Costs: A key metric, this reflects the overall cost savings achieved by reducing emergency repairs and labor costs typically associated with breakdowns.
- Increased Production Efficiency: By maintaining optimal equipment performance, businesses can boost output rates, contributing to higher profitability.
- Asset Availability: Assessing the percentage of time equipment is operational versus down for repairs provides insight into overall efficiency gains.
- Mean Time Between Failures (MTBF): This widely used metric measures the average time between equipment failures. An increase in MTBF after implementing predictive maintenance suggests a successful application of the strategy.
Predictive maintenance is not just about the maintenance costs. Itโs a comprehensive approach that affects overall business performance.
Future Trends in Predictive Maintenance
Future trends in predictive maintenance are reshaping how industries approach their operational strategies. This section delves into innovative technologies and best practices that are emerging on the horizon. Understanding these trends is vital as they can directly impact maintenance efficiency, cost savings, and equipment lifespan.
Advancements in Technology
Technology is evolving rapidly, and predictive maintenance is no exception. Some notable advancements include:
- Artificial Intelligence and Machine Learning: These technologies allow for more accurate predictions of equipment failures. Using vast amounts of data, AI algorithms can identify patterns that human analysts might miss.
- Internet of Things (IoT): The integration of IoT devices enables real-time monitoring of assets. Sensors can collect data continuously, providing insights into performance that can lead to proactive maintenance.
- Cloud Computing: By utilizing cloud services, organizations can store and analyze large datasets without the constraints of on-premise hardware. This flexibility enhances decision-making accessibility regardless of location.
- Digital Twins: Creating digital representations of physical assets allows for simulations and predictive analytics. Maintenance teams can model scenarios before implementing changes in the real world, minimizing risks.
These advancements provide organizations with the tools to operate more efficiently and effectively. However, the benefits extend beyond just operational improvements; they also foster a culture of innovation.
Emerging Best Practices
As industries adopt new technologies, several best practices are emerging that help organizations maximize the benefits of predictive maintenance:
- Holistic Data Utilization: Companies should leverage all available data sources, not just equipment sensors. Maintenance teams must integrate data from operations, equipment, and external sources to enhance prediction models.
- Regular Updates of Predictive Models: The continuous nature of machine learning allows for ongoing adjustments to predictive models. Organizations should schedule regular reviews to ensure models reflect the latest operational realities.
- Employee Training and Engagement: Incorporating predictive maintenance into employee training programs ensures that personnel are familiar with new tools and methodologies. Engaged employees are more likely to adopt and contribute to innovation efforts.
- Cross-Departmental Collaboration: Departments such as IT, operations, and maintenance must work together. This collaboration can lead to a more cohesive strategy, allowing for quicker responses to emerging trends.
"Embracing future trends in predictive maintenance is not merely an option but a strategic necessity for organizations thriving in todayโs competitive landscape."
End
The conclusion is a crucial aspect of this article as it encapsulates the significance of predictive maintenance across various industries. It draws together the key insights from prior sections, reinforcing the profound impact predictive maintenance has on operational efficiency, cost savings, and overall management practices. The emphasis lies on how methodologies discussed in the article can lead to reduced downtime, improved safety, and enhanced reliability of equipment.
Summary of Key Points
In summary, several important themes emerge from the exploration of predictive maintenance:
- Predictive maintenance uses advanced analytics and technologies like machine learning and IoT to predict equipment failures before they occur.
- Techniques such as vibration analysis, thermal imaging, and ultrasonic testing enable organizations to monitor the health of their assets effectively.
- The economic implications largely favor predictive maintenance, as the initial investment often leads to significant long-term savings and increased return on investment.
- Regulatory considerations and compliance must be kept in mind to ensure effective and lawful implementation of these techniques.
- The blending of traditional maintenance methods with new technology establishes a more robust maintenance strategy, optimizing asset life-cycle management.
These points illustrate not only the various facets of predictive maintenance but also its indispensable role in modern operational strategies.
Final Thoughts on Implementation
Implementation of predictive maintenance strategies requires careful consideration and planning. Organizations must assess their current maintenance frameworks, identify appropriate predictive techniques, and understand the required technological investments.
Engaging all levels of the organization, from the operational staff to upper management, is vital in fostering a culture supportive of change. Training sessions can assist in smoothing the transition, ensuring everyone understands the new systems and their benefits. Furthermore, evaluating the actual performance of predictive maintenance initiatives through continuous monitoring will help refine methods and improve outcomes.
Ultimately, organizations that embrace predictive maintenance not only position themselves to maximize efficiency but also create opportunities for innovation while keeping costs in check.
Cited Works
The articulation of concepts around predictive maintenance is enriched by referencing seminal works and current research. The following sources are instrumental in informing the discussions and findings presented:
- Wikipedia: For general definitions and historical context, Wikipedia serves as an accessible entry point for individuals seeking a broad overview. Its articles provide foundational knowledge that must be validated by more rigorous academic sources.
- Britannica: The content found in Britannica offers well-researched insights into the mechanisms and operational perspectives of predictive maintenance, making it a reliable source for deeper understanding.
- Reddit: Discussions and shared experiences on platforms like Reddit contribute to the real-world applications and challenges faced by industry practitioners, offering practical insights that theory alone may not address.
- Facebook: Groups and pages related to predictive maintenance on Facebook often feature case studies and user-generated content that highlight ongoing developments in the field, useful for those keeping up with latest trends.
Utilizing these resources allows readers to engage with a variety of perspectives and applications, reinforcing the diverse methodologies around predictive maintenance. It also underscores the importance of integrating academic rigor with practical experience in making informed decisions in maintenance strategies.