Essential Business Data Analysis Tools Explained
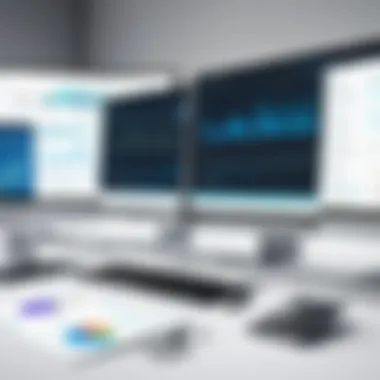
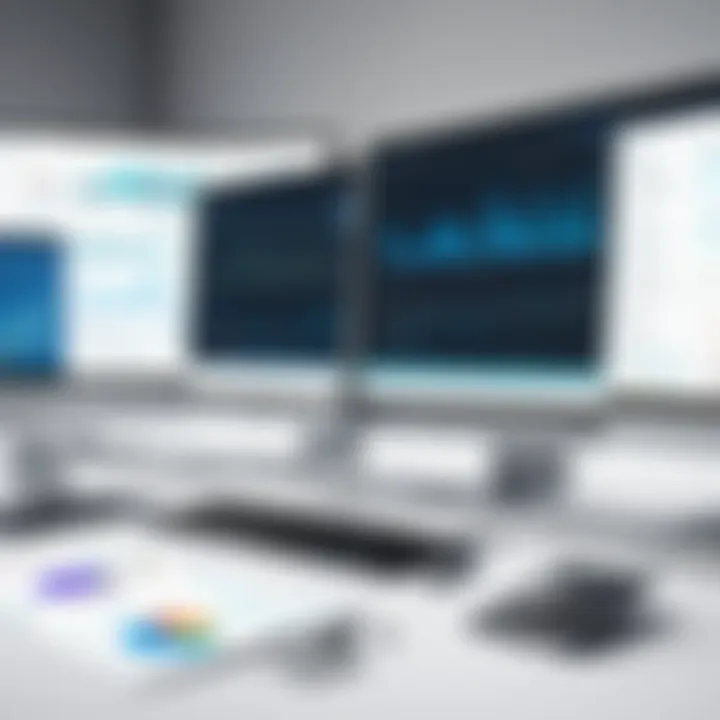
Intro
The rapid pace of development in data analysis tools for businesses mirrors the evolving landscape of modern enterprises. Companies today are increasingly reliant on comprehensive data to inform decisions, streamline operations, and enhance productivity. This need has spurred an influx of diverse tools, each offering unique functionalities tailored to various sectors and business sizes. In this article, we dissect the myriad of options available, aiming to provide a clear vantage point for decision-makers.
The decision to implement a data analysis tool is not one to be taken lightly. Understanding which tool fits best with your organizational needs necessitates a granular look at their features, applications across different industries, and user experiences. Therefore, reading this article will illuminate the complexities involved in choosing a data analysis solution, highlighting critical insights needed to make informed decisions.
Key Features and Functionalities
Comprehensive Overview
When evaluating data analysis tools, it's essential to grasp their key components. Most tools boast foundational features such as:
- Data Visualization: Presenting data in graphical formats helps in easier comprehension, thus aiding decision-making.
- Data Integration: The capacity to merge data from various sources allows companies to work with unified datasets.
- Predictive Analytics: Offering insights based on historical data, predictive capabilities help organizations anticipate future trends and behaviors.
- User-Friendly Interfaces: A good tool should have a straightforward layout that doesn't require extensive training.
- Collaboration Functions: Tools that facilitate team collaboration are invaluable, particularly in remote work settings.
These functionalities serve as the bedrock upon which effective data analysis is built. However, the effectiveness of these features often varies from one tool to another, and nuances in performance across different sectors shouldn't be overlooked.
Target Users
Understanding the demographics and professional backgrounds of tool users can provide context for their functionalities. Typically, the following groups heavily use these tools:
- IT Professionals: With a technical mindset, they often require deep analytical capabilities and customization options.
- Data Analysts: Focused on extracting insights, they benefit from robust data visualization and statistical analysis.
- Business Executives: Decision-makers who favor high-level overviews and succinct reports to guide strategic direction.
- Entrepreneurs: Often budget-conscious and seeking tools that are intuitive and scalable as their ventures grow.
Each user group approaches tools with different expectations. For example, entrepreneurs might prioritize cost-effectiveness and ease of use, while data analysts could seek advanced features and flexibility.
Pricing Models and Cost Analysis
Breakdown of Pricing Tiers
Navigating the pricing landscape of data analysis tools can be a daunting task. Here’s a rough sketch of what to expect in terms of pricing models:
- Free Tools: Some entry-level options come with basic features but may impose limits on data volume or functionality.
- Subscription-Based Plans: Most tools operate on a monthly or yearly subscription model, offering tiered pricing based on the depth of features. Basic plans may start around $30, while more comprehensive packages can soar over $200 per month.
- One-Time Purchases: For some businesses, investing a lump sum in software licenses is preferred, though this model is becoming less common.
Additional Costs to Consider
While the sticker price gives an initial impression, hidden costs may arise, including:
- Training and Onboarding: New tools often necessitate employee training, which can add to overall expenses.
- Integration Fees: If a tool needs to be integrated with existing systems, there can be additional charges to consider.
- Updates and Maintenance: Some tools may have associated costs for regular updates or tech support.
In the world of data analyses, being informed about potential total costs helps organizations budget effectively and avoid unpleasant surprises down the line.
"Knowledge is power, but only if you know how to use it effectively in the data-driven age of business."
By examining these elements, organizations can position themselves favorably in an ever-competitive environment. Overall, understanding the features and pricing structures empowers business leaders to make confident, informed choices tailored to their specific needs.
Prologue to Business Data Analysis Tools
In the fast-paced realm of modern business, understanding and interpreting data has taken center stage. The sheer volume of information that organizations generate and collect can be staggering. Thus emerges the need for robust business data analysis tools that not only sift through this data but also extract meaningful insights. These tools have become indispensable, acting as navigators in the sometimes turbulent waters of data management.
Defining Business Data Analysis
Business data analysis refers to the process of systematically applying statistical and logical techniques to evaluate data. It involves collecting, transforming, and modeling data with the ultimate goal of discovering useful information, drawing conclusions, and supporting decision-making. This definition encapsulates a variety of methods and techniques, offering a panoramic view of how businesses can leverage data effectively.
While the terminology might seem straightforward, the underlying complexities can quickly multiply. For instance, businesses should distinguish between different types of analysis—such as descriptive, predictive, and prescriptive—to tailor their strategies accordingly. Each type offers different insights and serves distinct business needs. Moreover, the increasing prevalence of big data complicates this landscape further, laying down the importance of having the right tools in place.
Importance in Today's Business Environment
In today’s highly competitive market, the significance of business data analysis tools cannot be overstated. As organizations strive to meet customer expectations, streamline operations, and boost profitability, the tools designed for data analysis become critical allies in achieving these objectives.
"In the age of information, those who can analyze and act on data are often the ones who succeed."
Consider how some firms have turned around their fortunes by employing data-driven strategies. With the insights derived from data analysis, companies can identify trends, anticipate market shifts, and fine-tune their project offerings. Proper data analysis enhances decision-making quality, enabling businesses to pivot swiftly and avoid pitfalls that иначе could lead to significant losses.
Furthermore, the digital transformation that many companies are currently navigating has made data more accessible yet more difficult to harness effectively. By integrating strong data analysis tools, organizations can better manage their resources, allocate budgets judiciously, and make informed choices that drive growth.
The combination of sophisticated technology and analytical methodologies paves the way for new opportunities. As customer behaviors evolve, data analysis supports the continuous refinement of strategies that keep businesses agile and resilient. The ability to adapt based on real-time data insights is, without a doubt, a decisive factor in a company’s ability to succeed.
Ultimately, as this article progresses through different categories, features, and popular tools, our aim is to provide a detailed landscape of business data analysis. It is crucial for decision-makers, IT professionals, and entrepreneurs to grasp how these tools can be harnessed not just to survive, but to thrive in their respective markets.
Categories of Business Data Analysis Tools
In the evolving world of data-led decision making, categorization of business data analysis tools is essential. Understanding which type of tool fits your organization's needs can dramatically enhance operational efficiency and inform better strategic choices. Essentially, these categories define how organizations can extract, interpret, and leverage their data for maximum impact. They draw a clear line on what each type of tool specializes in, allowing decision-makers to hone in on the most appropriate solutions for their specific contexts.
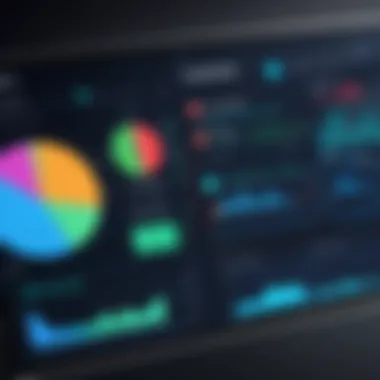
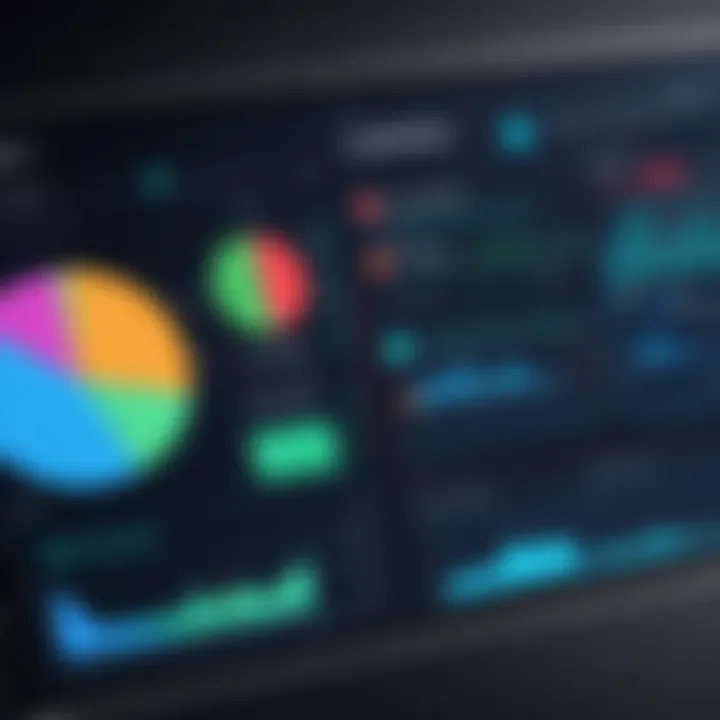
Descriptive Analytics Tools
Descriptive analytics is the starting point for any data analysis journey. These tools focus on what has happened in an organization and help in understanding historical data. Commonly used features include data aggregation and data mining, which yield insights about past performances. Take, for example, a retail business wanting to understand sales trends over the last year. By employing descriptive analytics tools like Tableau or Microsoft Power BI, the retailer can visualize patterns, segment customers, and analyze sales by different dimensions, such as region or product line.
Benefits include:
- Insight into Trends: Patterns and trends become evident through visual dashboards.
- Informed Decision-Making: Stakeholders can make well-grounded choices based on historical performance.
However, the downside lies in its limitation to historical data. Descriptive analytics does not predict future trends and could lead decision-makers to overlook pivotal upcoming trends or shifts in the market.
Predictive Analytics Tools
Moving up the ladder, predictive analytics tools enable businesses to anticipate what might happen in the future based on historical data. They employ statistical techniques and machine learning algorithms to analyze data patterns. For instance, a financial institution may use predictive analytics to forecast customer behavior, such as loan repayment probabilities. By using tools like IBM SPSS or SAS Analytics, analysts can create models that predict future trends with a certain degree of accuracy.
Key advantages of predictive analytics include:
- Future Insight: Ability to create simulations that can forecast future scenarios.
- Proactive Measures: Allows organizations to make preemptive adjustments to their strategies based on predicted outcomes.
Despite the advantages, there are challenges involved, like the requirement for high-quality data and the nuance in interpreting outcomes. As the old saying goes, "GIGO"—Garbage In, Garbage Out. If your data is flawed, predictions will likely be too.
Prescriptive Analytics Tools
Prescriptive analytics, on the other hand, takes it up a notch further. These tools guide organizations on what actions to take in different scenarios. Utilizing algorithms and optimization techniques, prescriptive analytics provides recommendations for decision-making. A classic example is supply chain management, where a logistics company uses prescriptive analytics to determine the most efficient routes and delivery schedules using tools like Oracle Spatial and Graph.
The benefits here include:
- Actionable Recommendations: Provides tailored strategies that are grounded in data analysis.
- Optimized Outcomes: The potential to enhance resource allocation and operational efficiency is significant.
But it’s worth noting that prescriptive analytics can also be complex, requiring cross-functional collaboration across departments. Therefore, its successful implementation relies on effective communication and a clear understanding of the complexities involved.
"In a world awash with data, knowing what category of analysis to apply can mean the difference between seizing an opportunity and missing the boat."
Selecting the right category of business data analysis tools demands a clear comprehension not only of the tool features but also of the organizational goals and contextual nuances. Each category plays a crucial role in seamlessly transforming data into knowledge for effective decision-making.
Key Features of Effective Data Analysis Tools
In today's fast-paced business environment, choosing the right data analysis tool is like picking the right fishing rod for bass fishing; having the right features can make all the difference between landing a big catch or coming home empty-handed. The tools available are diverse, but their effectiveness largely hinges on specific features that make them stand out. Understanding these features is crucial for decision-makers as it directly influences the quality and effectiveness of data-driven decisions. In this section, we will unpack three key features that make data analysis tools effective: data visualization capabilities, integration with existing systems, and user-friendliness and accessibility.
Data Visualization Capabilities
Data visualization is more than just pretty graphs and charts—it’s a vital component that aids in analyzing complex data trends and patterns swiftly. Imagine trying to communicate a year’s worth of sales data using only numbers; the meaning can get lost faster than a sock in a dryer. With robust data visualization tools, stakeholders can gain insights at a glance. Well-designed visual tools allow users to transform heaps of data into visual representations, making it easier to spot anomalies and trends.
- Benefits:
- Enhances comprehension: Human brains process visuals faster than text; compelling visuals can make understanding possible for all team members, not just the data scientists.
- Facilitates storytelling: A good visualization enables business leaders to tell a compelling story with data, making presentations more engaging and impactful.
Ultimately, if a tool doesn’t offer effective visualization capabilities, it might be wise to think twice before investing.
Integration with Existing Systems
In a business ecosystem, effective data analysis tools must play nice with the tools already in place. No one wants to disrupt the flow more than a wrench in a finely-tuned machine. Integrating data analysis tools with existing systems—such as CRM, ERP, and other analytics platforms—ensures that all departments are singing from the same hymn sheet.
- Considerations:
- Ease of integration: Look for tools that allow drag-and-drop features or straightforward API accessibility so teams can set them up with minimal hassle.
- Data integrity: Integration safeguards data consistency across various platforms, ensuring reliable analytics without double entry that can create errors.
Significantly, integration can streamline operations, allowing for a centralized approach to data management, often saving time and reducing costs.
User-Friendliness and Accessibility
We’ve all encountered overly complicated software that makes one feel like they’re trying to decipher ancient hieroglyphs. Ideally, a data analysis tool should be user-friendly, offering intuitive interfaces that allow both tech-savvy individuals and laypeople to navigate easily. Accessibility matters as well; if a tool is only operable on certain devices or networks, it can restrict its user's ability to access information.
- Benefits:
- Training time: The easier it is to use a tool, the less time employees will need for training, allowing teams to get to work without steep learning curves.
- Broader participation: Involving non-technical staff in the data analysis process encourages a culture of data-driven decision-making across the organization.
A consistent user experience across platforms ensures that insights can be generated anywhere, anytime, catering to today’s flexibility demands in the workplace.
"The most valuable decision-making tool isn't the tool itself, but how well it's integrated and embraced within the organization."
When scouting for effective data analysis tools, it’s the presence of these key features that often determines their success. By focusing on data visualization capabilities, integration with existing systems, and user-friendliness, organizations are better equipped to choose tools that will not only meet their immediate needs but also adapt to future growth.
Popular Business Data Analysis Tools
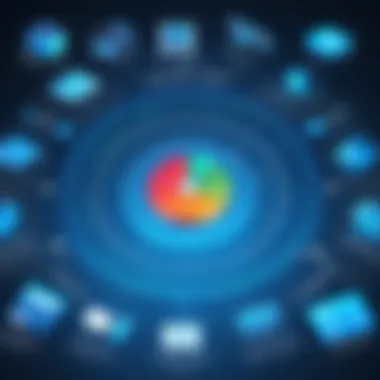
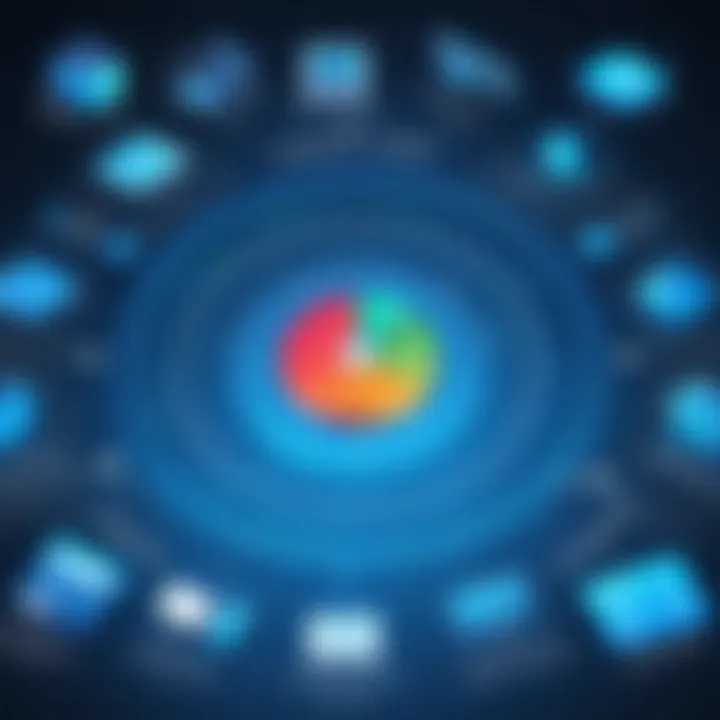
Understanding the tools available for business data analysis is crucial in today’s digitally-driven marketplace. A robust tool can offer insights that inform strategic decisions, enhancing both efficiency and effectiveness. In this section, we will dive into some of the most popular tools that have made significant impacts in the arena of business analytics.
Tableau
Overview and Features
Tableau stands as a titan in the landscape of data visualization tools, primarily known for its ability to turn data into accessible and comprehensible visuals with ease. It’s like giving a magnifying glass to raw data; suddenly, what was once complex and nebulous becomes straightforward and clear as day. One of Tableau’s strongest suits is its drag-and-drop interface which allows users, regardless of their technical expertise, to create meaningful visualizations without needing to write code.
Uniquely, Tableau caters to various data sources, enabling connections not just to spreadsheets, but also to databases and even cloud services like Google Analytics or Amazon Web Services. This flexibility makes it a formidable choice for businesses spanning multiple sectors.
Benefits
The benefits of using Tableau are manifold. Firstly, its powerful data visualization capabilities can help any organization clearly depict trends and patterns over extensive datasets. This feature isn’t just beneficial; it can be a game-changer. Moreover, the ability to share reports and dashboards online fosters collaboration and informed decision-making among team members.
Another noteworthy characteristic is its community support. With countless resources, forums, and tutorials available, users can easily find help—an aspect that significantly reduces learning curves for new users.
Drawbacks
However, it’s not all sunshine and rainbows with Tableau. One of its primary drawbacks is the cost factor. For small businesses or startups, the licensing fees can seem a bit steep. Additionally, while Tableau offers a wide range of visualization styles, customization options can be somewhat limited. This can lead frustrated users to grapple with workarounds that detract from their intended straightforward experience.
Microsoft Power BI
Overview and Features
Power BI from Microsoft is another heavyweight contender for data analysis tools. Built to analyze and visualize data from a myriad of sources, Power BI stands apart with its integration within the Microsoft ecosystem. Its seamless connections to Excel, Azure, and even Dynamics 365 provide users with a familiar interface, making it easier to adopt.
One defining feature is Power BI’s real-time dashboards which allow businesses to track live data as it’s generated. This can help organizations stay agile, reacting swiftly to changes in market conditions.
Benefits
The strengths of Power BI speak volumes. It offers comprehensive reporting tools that let users drill down into data. This detailed analysis can uncover patterns that would likely go unnoticed using simpler tools. Furthermore, the pricing model of Power BI tends to be more favorable for small to medium-sized businesses, offering a range of subscription options.
Moreover, Power BI facilitates easy sharing and collaboration, integrating directly into Microsoft Teams and SharePoint. This means teams can work collectively in real-time, improving efficiencies and outcomes.
Drawbacks
Nonetheless, Power BI is not free of its own pitfalls. One issue is the steep learning curve that can accompany more complex functionalities. To fully harness its capabilities, users may need training or extensive practice. Furthermore, while the integration with Microsoft products is a major advantage, it could also feel limiting for those who rely on other systems or platforms for their reporting needs.
Google Analytics
Overview and Features
Google Analytics, initially a tool for tracking website performance, has expanded its prowess into business data analysis, offering insights into user behavior and operational efficiency. Its primary feature revolves around tracking metrics such as traffic volumes, user demographics, and site engagement metrics. For businesses, understanding these factors can help shape marketing strategies effectively.
Notably, Google Analytics provides a wealth of customer insights at no cost, making it an accessible entry point for organizations wanting to dip their toes into data analysis without a hefty investment.
Benefits
The benefits are rather pronounced. The ability to gather and analyze website data helps businesses tweak and enhance their online strategies. Users can set up goals and track conversions, ensuring they leverage their marketing efforts on strategic initiatives that yield returns.
Another remarkable aspect is the integration with Adwords and other Google services, creating a holistic view of marketing effectiveness, which greatly assists in optimizing campaigns.
Drawbacks
But, much like its counterparts, Google Analytics isn't without flaws. Many users find the platform’s interface overwhelming at first glance, especially with its depth of information presented. Additionally, while the free version is powerful, businesses requiring advanced features must consider the premium version, which could strain budgets.
In summary, popular business data analysis tools like Tableau, Microsoft Power BI, and Google Analytics offer unique features, benefits, and challenges. Understanding these tools is paramount for businesses striving to navigate the modern data landscape effectively.
Selecting the Right Business Data Analysis Tool
Choosing the appropriate data analysis tool is no walk in the park. The right tool can be a game-changer in how a business operates and makes critical decisions. In today’s fast-paced environment, the selection process needs to consider a variety of elements, including specific business needs, budget, and the potential for future growth. Emphasizing these considerations can lead organizations to a tool that not only meets their current requirements but also adapts as they evolve.
Identifying Organizational Needs
Before diving headfirst into the sea of options, taking a step back to identify the unique organizational needs is crucial. Businesses vary significantly in what they require from data analysis tools.
- What data types do you work with? Understanding the nature of your data—be it structured or unstructured—will help narrow the choices.
- What type of insights are you looking for? Descriptive, predictive, or prescriptive analytics might be the answer, and the right tool will align with these aims.
- Who will be using the tool? It is essential to consider the skillsets of your team. Some tools are designed for analysts, while others cater to end-users with little technical know-how.
Understanding these factors helps set the stage for a considered choice, ensuring that the selected tool brings real value to the table.
Assessing Budget Constraints

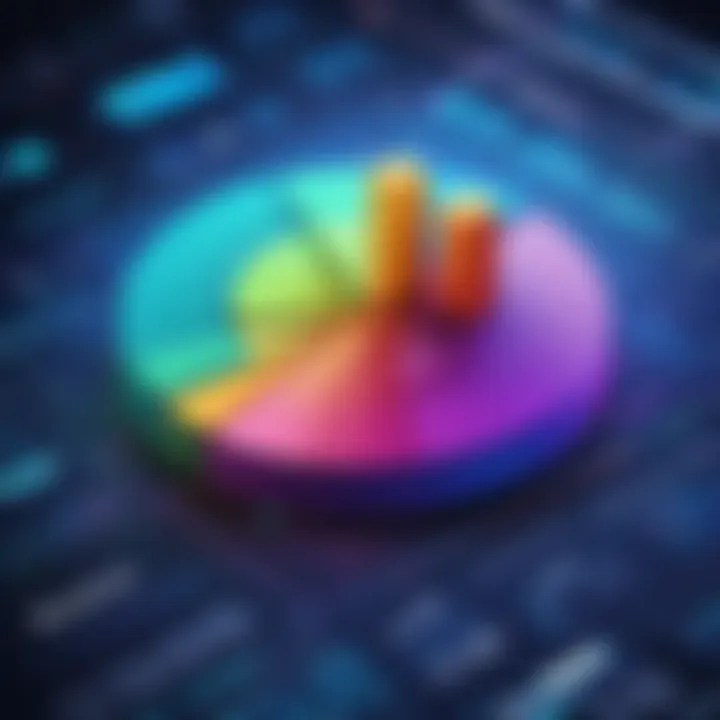
Money speaks volumes in the decision-making process. Budget constraints should never be an afterthought. Before zeroing in on a tool, it's essential to—
- Evaluate total ownership costs: Beyond just the purchase price, consider maintenance, training, and potential scaling costs. Some tools come with hidden fees that can add up unexpectedly.
- Consider alternatives: Sometimes, less popular but effective tools can provide similar functionality at a fraction of the cost. Open-source options might also be worth exploring.
- Look for trial periods: Many vendors offer trial versions. Using these can provide insight into whether the tool delivers value without a hefty financial commitment.
Budgeting wisely is not only about keeping costs down but ensuring that investments lead to measurable benefits.
Considering Scalability and Future Growth
A tool might be a perfect fit today, but what about tomorrow? Future-proofing is an often overlooked aspect of selection.
- Assess scalability: Will your chosen tool be able to handle increasing data volumes as your organization grows? Consider if it can accommodate new features or integrate with emerging technologies.
- Stay open to evolution: Business needs can change in the blink of an eye. Evaluating tools for flexibility allows businesses to pivot without starting over from scratch.
- Investigate vendor reputation: Look closely at customer feedback. Is the vendor known for evolving their tools? Keeping this in mind can help mitigate the risk of having to switch tools down the line due to stagnation.
True sustainability in a data analysis tool hinges on whether it can adapt alongside your organization. Keeping these elements in check during tool selection can lead to more efficient operations and improved decision-making.
Challenges in Business Data Analysis
Understanding the hurdles in business data analysis is crucial for decision-makers looking to harness the full potential of their analytical tools. These challenges can significantly impact the accuracy of insights drawn from data. Whether it’s data quality or the human aspects of adopting new technologies, these issues can’t be treated lightly. Tackling them head-on not only sharpens the effectiveness of data analysis but also aligns it closely with organizational goals. Let's dive into the most pressing challenges and explore how they affect businesses today.
Data Quality and Accuracy
Data quality is often the backbone of effective data analysis. When decisions are made based on inaccurate or incomplete data, it’s like building a house on sand; the structure will ultimately collapse. There are several dimensions to data quality, primarily focusing on completeness, consistency, and accuracy.
- Completeness: This refers to how much of the necessary data is actually available. Missing data can skew results, leading to misleading conclusions.
- Consistency: Data should not contradict itself across different sources. Inconsistencies can arise from disparate systems not talking to each other properly, resulting in a tangled web of unreliable information.
- Accuracy: This deals with how close data is to reality. Misentries, outdated information, or simple typos can compromise the entire analysis.
The above points showcase that ensuring high-quality data requires robust data governance practices. Organizations must establish protocols for data collection, storage, and management to ensure that they have a solid foundation for analysis. As the saying goes, "Garbage in, garbage out!" If the initial data is flawed, the insights generated will be similarly affected.
Overcoming Resistance to Adoption
Change is never easy, especially when it comes to integrating new tools into longstanding processes. Resistance to adopting advanced data analysis tools can stem from various factors, including a lack of understanding, fear of the unknown, or even dissatisfaction with previous software implementations.
Some strategies to mitigate this resistance include:
- Training Programs: Providing comprehensive training can reduce the anxiety around new tools. When employees understand how to use a tool effectively, they’re less likely to resist it.
- Involvement in Decision-Making: Engaging team members in the selection process can foster a sense of ownership and lessen pushback. When they feel their opinions count, they are more likely to support the change.
- Highlighting Benefits: Clearly articulating the advantages of the new tool—whether it be efficiency gains, increased accuracy, or time savings—can incentivize users to embrace the new system.
Adopting new analysis tools is not merely a technological shift; it's also a cultural change within the organization. To quote a well-known adage, "You can't teach an old dog new tricks" – unless you show them why the new trick is valuable.
In summary, addressing the challenges of data quality and overcoming resistance to adoption are vital steps for any organization aiming to thrive in today's data-driven landscape. By focusing on these challenges, companies can pave the way for smoother transitions and more effective analysis.
Emerging Trends in Data Analysis Tools
This section dives into the emerging trends in data analysis tools that significantly shape the current business transformation. As the landscape of data analysis continues to shift, organizations are increasingly recognizing the importance of integrating new solutions that not only streamline processes but also enhance decision-making capabilities. These trends signify a move toward a more data-driven approach, empowering businesses to harness insights and improve strategies. Here, we’ll explore some key elements, benefits, and considerations surrounding these trends.
Artificial Intelligence and Machine Learning Integration
The integration of Artificial Intelligence (AI) and Machine Learning (ML) in data analysis tools marks a groundbreaking shift in how businesses interpret and use their data. This advancement allows for more sophisticated analytics, enabling data to be processed and analyzed far beyond basic metrics.
"Harnessing the power of AI and ML can drastically improve an organization's ability to adapt to the market and anticipate customer needs."
Some important aspects worth noting include:
- Automated Insights: AI can uncover patterns and trends in large datasets, providing insights that would take human analysts much longer to discover.
- Predictive Analytics: Machine learning algorithms can predict future trends based on historical data, allowing companies to proactively respond to market changes.
- Improving Customer Experience: Personalized recommendations powered by AI lead to better-targeted marketing and enhanced user satisfaction.
However, while the benefits are clear, organizations must consider the initial setup costs, the need for skilled personnel to manage these systems, and the ongoing challenge of data privacy and ethical use of AI.
Real-time Data Processing
In today's fast-paced business environment, the ability to process data in real-time is no longer a luxury; it's a necessity. Real-time data processing enables organizations to analyze data as it comes in, rather than relying on historical data that may be obsolete. This trend is instrumental in industries where timely decision-making is crucial, such as finance and e-commerce.
Real-time processing offers several advantages:
- Immediate Responsiveness: Businesses can react to market conditions, customer behaviors, or potential issues as they unfold.
- Enhanced Operational Efficiency: By having access to current data, organizations can optimize processes and allocate resources more effectively.
- Data-Driven Decisions: Decision-makers can rely on the most up-to-date information, minimizing risks associated with outdated analytics.
Nonetheless, implementing real-time data processing can be complex. Organizations must invest in the right technology infrastructure, ensure data quality, and train staff adequately to leverage this capability.
End
In the realm of business, the ability to make informed decisions based on data is invaluable. Concluding this exploration of business data analysis tools, the significance of understanding this topic cannot be overstated. With the vast selection of tools available, organizations must not only grasp their features but also comprehend how each can align with their unique needs. This conclusion sums up the pivotal insights presented throughout the article.
Recap of Key Points
- Diverse Categories: The tools can be categorized into descriptive, predictive, and prescriptive analytics, each serving a distinct purpose in decision-making.
- Key Features: Essential features include data visualization, ease of integration with existing systems, and user-friendliness, which are critical in ensuring that the analysis resonates with its end-users.
- Popular Tools: From Tableau's impressive visualization capabilities to Microsoft Power BI's robust integration, businesses have a variety of effective options.
- Challenges: Data quality and resistance to adoption can hinder successful implementation of data analysis tools, making it crucial to address these obstacles upfront.
- Emerging Trends: Trends like the integration of AI and real-time processing are reshaping the analytics landscape, making it imperative for organizations to stay informed and adapt.
Final Thoughts on Choosing Analysis Tools
When selecting the most suitable business data analysis tools, decision-makers should take a multi-faceted approach. It’s not merely about the tool's capabilities, but also about how it fits within the broader strategy of the organization. Here are a few final considerations:
- Align Tool with Business Goals: Each tool should align with what the organization aims to achieve. Whether it's improving operational efficiency or enhancing customer insights, clarity in goals helps narrow options.
- Conduct Thorough Research: Explore different options, read reviews, and consider demo versions to understand functionality firsthand. It’s important to see how these tools perform in real-world scenarios.
- Evaluate Support and Training: Companies should factor in the availability of customer support and training resources. Effective training can optimize tool usage and mitigate any initial resistance from team members.
- Scalability: The chosen tools should have the flexibility to grow alongside the organization. A tool that works for a small business may not be ideal as it scales—consideration of scalability is key.
In summary, as data continues to play an increasingly critical role in business success, the frameworks and tools selected for analysis must not only meet immediate needs but also accommodate future growth and challenges. Embracing a thoughtful approach to these decisions will undoubtedly lead to a more data-driven organizational culture.